Розробка методики прийняття рішень на основі сентимент-аналізу даних краудсорсингу в медичних соціальних ресурсах
DOI:
https://doi.org/10.15587/1729-4061.2023.289989Ключові слова:
медичні соціальні медіа, прийняття рішень, думки пацієнтів, діяльність клініки, аналіз настроївАнотація
Об’єктом дослідження є моделювання прийняття рішень у контексті медичних соціальних мереж для підвищення ефективності клінік. Проблема полягає в тому, щоб класифікувати відгуки пацієнтів, зібрані в сегменті пацієнт-клініка медичних соціальних медіа, і виявити ситуацію, пов’язану з діяльністю клінік, виявивши критерії, що характеризують діяльність клінік поза відгуками.
Запропонована методика стосується аналізу настроїв думок на основі лексикону, класифікації на основі словника Valence Aware та Sentiment Reasoner (VADER), перевірки точності результатів за допомогою Multinomial Naive Bayes and Support Vector Machine, ручного аналізу настроїв думок для виявлення критерії та класифікація думок відповідно до кожного критерію.
За допомогою цієї методики з 442587 відгуків пацієнтів, отриманих з бази даних cms_hospital_satisfaction_2020 компанії Kaggle, згенерованої на основі краудсорсингу відгуків пацієнтів у медичних соціальних мережах, 218914 відгуків пацієнтів класифікуються як позитивні, 190360 – як нейтральні, а 33313 – як негативні. Достовірність результатів перевіряється, а клініки оцінюються «позитивними» відгуками. Виявлено 6 нових критеріїв, що характеризують діяльність клінік, і наведено ідентифікацію ситуації, пов’язаної з діяльністю клінік, на основі порівняння «позитивних» і «негативних» думок за кожним критерієм.
Показано можливість використання результатів ідентифікації для підвищення ефективності прийняття рішень клініками.
Результати, отримані в цьому дослідженні, можуть бути використані для покращення роботи клінік відповідно до громадської думки. Ця можливість передбачає краудсорсинг думок про клініку в середовищі медичних соціальних мереж і збір думок у структурований спосіб.
Посилання
- Emin, M. (2018). A Qualitative Study on the Reasons for Social Media Addiction. European Journal of Educational Research, 7 (4), 861–865. doi: https://doi.org/10.12973/eu-jer.7.4.861
- Yusifov, F., Alguliyev, R., Aliguliyev, R. (2018). Role of Social Networks in E-government: Risks and Security Threats. Online Journal of Communication and Media Technologies, 8 (4). doi: https://doi.org/10.12973/ojcmt/3957
- Salikhova, N. R., Grigoryeva, O. V., Semenova-Poliakh, G. G., Salikhova, A. B., Smirnikova, O. V., Sopun, S. M. (2023). Communication tools and social media usage: Assessing self-perceived communication competence. Online Journal of Communication and Media Technologies, 13 (4), e202343. doi: https://doi.org/10.30935/ojcmt/13453
- Zaw, H. T. (2018). The Impact of Social Media on Cultural Adaptation Process: Study on Chinese Government Scholarship Students. Advances in Journalism and Communication, 06 (03), 75–89. doi: https://doi.org/10.4236/ajc.2018.63007
- Swan, M. (2009). Emerging Patient-Driven Health Care Models: An Examination of Health Social Networks, Consumer Personalized Medicine and Quantified Self-Tracking. International Journal of Environmental Research and Public Health, 6 (2), 492–525. doi: https://doi.org/10.3390/ijerph6020492
- Cesare, N., Grant, C., Hawkins, J. B., Brownstein, J. S., Nsoesie, E. O. (2017). Demographics in Social Media Data for Public Health Research: Does it matter? Bloomberg Data for Good Exchange Conference. doi: https://doi.org/10.48550/arXiv.1710.11048
- Renuka, D. K., Jeetha, B. R. (2017). Dynamic and reliable intelligent data mining technique on social media drug related posts. 2017 IEEE International Conference on Power, Control, Signals and Instrumentation Engineering (ICPCSI). doi: https://doi.org/10.1109/icpcsi.2017.8392022
- Yadav, S., Ekbal, A., Saha, S., Bhattacharyya, P. (2018). Medical Sentiment Analysis using Social Media: Towards building a Patient Assisted System. Proceedings of the Eleventh International Conference on Language Resources and Evaluation (LREC 2018). Miyazaki, 2790–2797. Available at: https://aclanthology.org/L18-1442.pdf
- Zhang, M., Zhang, M., Ge, C., Liu, Q., Wang, J., Wei, J., Zhu, K. Q. (2019). Automatic discovery of adverse reactions through Chinese social media. Data Mining and Knowledge Discovery, 33 (4), 848–870. doi: https://doi.org/10.1007/s10618-018-00610-2
- Wang, C., Han, L., Stein, G., Day, S., Bien-Gund, C., Mathews, A. et al. (2020). Crowdsourcing in health and medical research: a systematic review. Infectious Diseases of Poverty, 9 (1). doi: https://doi.org/10.1186/s40249-020-0622-9
- Tucker, J. D., Day, S., Tang, W., Bayus, B. (2019). Crowdsourcing in medical research: concepts and applications. PeerJ, 7, e6762. doi: https://doi.org/10.7717/peerj.6762
- Mammadova, M., Jabrayilova, Z. (2019). Electronic medicine: formation and scientific-theoretical problems. Baku: "Information Technologies" publishing house, 319. Available at: https://ict.az/uploads/files/E-medicine-monograph-IIT-ANAS.pdf
- Medina-Aguerrebere, P., Medina, E., Gonzalez-Pacanowski, T. (2023). Branding cancer research institutions through social media platforms. Online Journal of Communication and Media Technologies, 13 (2), e202313. doi: https://doi.org/10.30935/ojcmt/12955
- Swan, M. (2012). Crowdsourced Health Research Studies: An Important Emerging Complement to Clinical Trials in the Public Health Research Ecosystem. Journal of Medical Internet Research, 14 (2), e46. doi: https://doi.org/10.2196/jmir.1988
- Mammadova, M. H., Jabrayilova, Z. G., Isayeva, A. M. (2019). Analysis of physician-patient relations segment of social media: opportunities and challenges. Problems of information society, 2, 41–50. doi: https://doi.org/10.25045/jpis.v10.i2.04
- Mammadovа, M., Jabrayilova, Z., Isayeva, A. (2020). Conceptual Approach to the Use of Information Acquired in Social Media for Medial Decisions. Online Journal of Communication and Media Technologies, 10 (2). doi: https://doi.org/10.29333/ojcmt/7877
- Mammadova, M., Jabrayilova, Z., Shikhaliyeva, N. (2022). Lexicon-based sentiment analysis of medical data. Technology Transfer: Fundamental Principles and Innovative Technical Solutions, 7–10. doi: https://doi.org/10.21303/2585-6847.2022.002671
- Simsek, A., Elciyar, K., Kizilhan, T. (2019). A Comparative Study on Social Media Addiction of High School and University Students. Contemporary Educational Technology, 10 (2), 106–119. doi: https://doi.org/10.30935/cet.554452
- Tunc-Aksan, A., Evin, S. (2019). Smartphone Addiction, Fear of Missing Out, and Perceived Competence as Predictors of Social Media Addiction of Adolescents. European Journal of Educational Research, 8 (2), 559–569. doi: https://doi.org/10.12973/eu-jer.8.2.559
- Recio Moreno, D., Gil Quintana, J., Romero Riaño, E. (2023). Impact and engagement of sport & fitness influencers: A challenge for health education media literacy. Online Journal of Communication and Media Technologies, 13 (3), e202334. doi: https://doi.org/10.30935/ojcmt/13309
- Lyu, H., Wang, J., Wu, W., Duong, V., Zhang, X., Dye, T. D., Luo, J. (2022). Social media study of public opinions on potential COVID-19 vaccines: informing dissent, disparities, and dissemination. Intelligent Medicine, 2 (1), 1–12. doi: https://doi.org/10.1016/j.imed.2021.08.001
- Sri, V. I. S. R., Niharika, Ch., Maneesh, K., Ismail, Dr. M. (2019). Sentiment Analysis of Patients’ Opinions in Healthcare using Lexicon-based Method. International Journal of Engineering and Advanced Technology, 9 (1), 6977–6981. doi: https://doi.org/10.35940/ijeat.a2141.109119
- Khan, M. T., Khalid, S. (2015). Sentiment Analysis for Health Care. International Journal of Privacy and Health Information Management, 3 (2), 78–91. doi: https://doi.org/10.4018/ijphim.2015070105
- Ilyasova, R. (2019). Fundamentals of Health Economics. Kazan: Kazan University Publishing House, 86. Available at: https://kpfu.ru/staff_files/F167974908/POSOBIE__KFU__Osnovy_EZ_Ilyasova_A.R._2019__1_.pdf
- Botha, Y. (2023). Determinants of social organizational credibility: Towards a formal conceptualization. Online Journal of Communication and Media Technologies, 13 (3), e202329. doi: https://doi.org/10.30935/ojcmt/13218
- Aattouchi, I., Elmendili, S., Elmendili, F. (2021). Sentiment Analysis of Health Care: Review. E3S Web of Conferences, 319, 01064. doi: https://doi.org/10.1051/e3sconf/202131901064
- Kausar, S., Huahu, X., Ahmad, W., Shabir, M. Y., Ahmad, W. (2020). A Sentiment Polarity Categorization Technique for Online Product Reviews. IEEE Access, 8, 3594–3605. doi: https://doi.org/10.1109/access.2019.2963020
- Hamdan, H., Bellot, P., Bechet, F. (2015). Sentiment Lexicon-Based Features for Sentiment Analysis in Short Text. Research in Computing Science, 90 (1), 217–226. doi: https://doi.org/10.1007/978-3-319-18117-2_9
- Baid, P., Gupta, A., Chaplot, N. (2017). Sentiment Analysis of Movie Reviews using Machine Learning Techniques. International Journal of Computer Applications, 179 (7), 45–49. doi: https://doi.org/10.5120/ijca2017916005
- Yadav, S., Shukla, S. (2016). Analysis of k-Fold Cross-Validation over Hold-Out Validation on Colossal Datasets for Quality Classification. 2016 IEEE 6th International Conference on Advanced Computing (IACC). doi: https://doi.org/10.1109/iacc.2016.25
- U.S. Hospital Customer Satisfaction 2016-2020. Available at: https://www.kaggle.com/datasets/abrambeyer/us-hospital-customer-satisfaction-20162020?select=cms_hospital_patient_satisfaction_2020.csv
- Prakash, P. R., Aruna, K. D. (2019). Business intelligence analytics using sentiment analysis-a survey. International Journal of Electrical and Computer Engineering (IJECE), 9 (1), 613. doi: https://doi.org/10.11591/ijece.v9i1.pp613-620
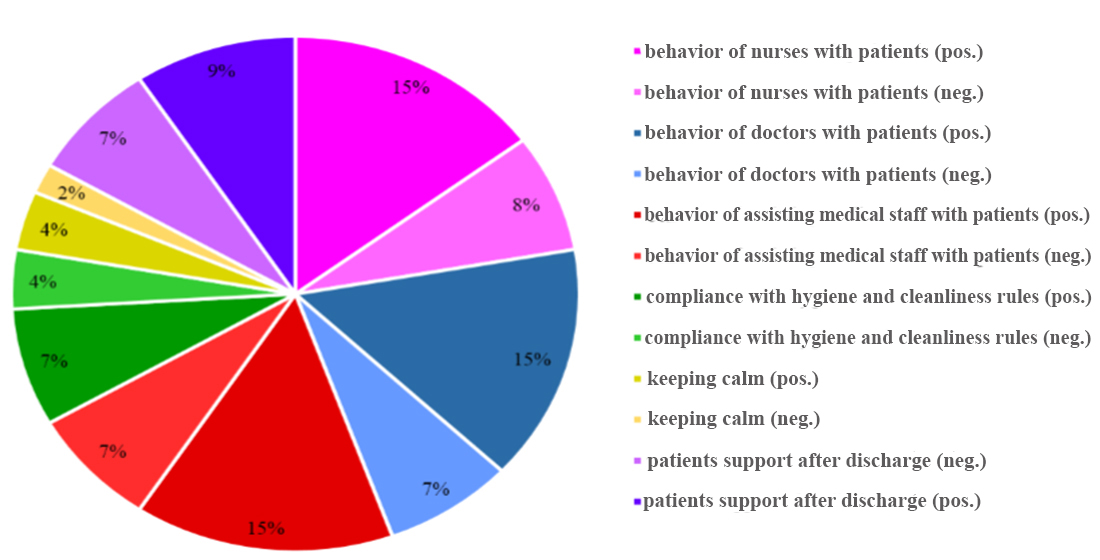
##submission.downloads##
Опубліковано
Як цитувати
Номер
Розділ
Ліцензія
Авторське право (c) 2023 Masuma Mammadova, Zarifa Jabrayilova, Nargiz Shikhaliyeva

Ця робота ліцензується відповідно до Creative Commons Attribution 4.0 International License.
Закріплення та умови передачі авторських прав (ідентифікація авторства) здійснюється у Ліцензійному договорі. Зокрема, автори залишають за собою право на авторство свого рукопису та передають журналу право першої публікації цієї роботи на умовах ліцензії Creative Commons CC BY. При цьому вони мають право укладати самостійно додаткові угоди, що стосуються неексклюзивного поширення роботи у тому вигляді, в якому вона була опублікована цим журналом, але за умови збереження посилання на першу публікацію статті в цьому журналі.
Ліцензійний договір – це документ, в якому автор гарантує, що володіє усіма авторськими правами на твір (рукопис, статтю, тощо).
Автори, підписуючи Ліцензійний договір з ПП «ТЕХНОЛОГІЧНИЙ ЦЕНТР», мають усі права на подальше використання свого твору за умови посилання на наше видання, в якому твір опублікований. Відповідно до умов Ліцензійного договору, Видавець ПП «ТЕХНОЛОГІЧНИЙ ЦЕНТР» не забирає ваші авторські права та отримує від авторів дозвіл на використання та розповсюдження публікації через світові наукові ресурси (власні електронні ресурси, наукометричні бази даних, репозитарії, бібліотеки тощо).
За відсутності підписаного Ліцензійного договору або за відсутністю вказаних в цьому договорі ідентифікаторів, що дають змогу ідентифікувати особу автора, редакція не має права працювати з рукописом.
Важливо пам’ятати, що існує і інший тип угоди між авторами та видавцями – коли авторські права передаються від авторів до видавця. В такому разі автори втрачають права власності на свій твір та не можуть його використовувати в будь-який спосіб.