Розробка передової моделі для аналізу психоемоційного стану людини на основі штучного інтелекту
DOI:
https://doi.org/10.15587/1729-4061.2023.293011Ключові слова:
розпізнавання мовних емоцій, глибинне навчання в розпізнаванні мовних емоцій, спектрограма MEL, аналіз MFCC, обробка звукових сигналів, емоційна класифікація, акустичні особливості, машинне навчання, виявлення емоцій, психолінгвістикаАнотація
Дослідження зосереджено на розробці нового методу автоматичного розпізнавання психоемоційних станів людини (ПСЛ) за допомогою технології глибокого навчання. Цей метод зосереджений на аналізі мовних сигналів для класифікації різних емоційних станів. Основним завданням цього дослідження є точна багатокласова класифікація семи психоемоційних станів людини, а саме радості, страху, гніву, смутку, огиди, здивування та нейтрального стану. Традиційні методи намагалися точно розрізнити ці складні емоційні нюанси в мові. Дослідження успішно розробило модель, здатну витягувати інформативні характеристики з аудіозаписів, зокрема мел-спектрограми та мел-частотні кепстральні коефіцієнти. Потім ці функції були використані для навчання двох глибоких згорткових нейронних мереж, що призвело до створення моделі класифікатора. Унікальність цього дослідження полягає у використанні подвійного підходу та використання глибоких згорткових нейронних мереж для класифікації. Цей підхід продемонстрував високу точність у розпізнаванні емоцій із показником точності 0,93 у підмножині перевірки. Високу точність і ефективність моделі можна пояснити комплексним і синергетичним використанням мел-спектрограм і мел-частотних кепстральних коефіцієнтів, які забезпечують детальніший аналіз емоційних проявів у мовленні. Метод, представлений у цьому дослідженні, має широке застосування в різних сферах, у тому числі для покращення взаємодії між людино-машинними інтерфейсами, впровадження в авіаційній промисловості, охороні здоров’я, маркетингу та інших сферах, де розуміння людських емоцій через мову має вирішальне значення
Спонсор дослідження
- This research was funded by the Science Committee of the Ministry of Education and Science of the Republic of Kazakhstan (Grant No. AP09258659).
Посилання
- Semigina, T., Vysotska, Z., Kyianytsia, I., Kotlova, L., Shostak, I., Kichuk, A. (2021). Psycho-Emotional State Of Students: Research And Regulation. Studies of Applied Economics, 38 (4). doi: https://doi.org/10.25115/eea.v38i4.4049
- Amirgaliyev, Y. N., Bukenova, I. N. (2021). Recognition of a psychoemotional state based on video surveillance: review. Journal of Mathematics, Mechanics and Computer Science, 112 (4). doi: https://doi.org/10.26577/jmmcs.2021.v112.i4.11
- Alharbi, S., Alrazgan, M., Alrashed, A., Alnomasi, T., Almojel, R., Alharbi, R. et al. (2021). Automatic Speech Recognition: Systematic Literature Review. IEEE Access, 9, 131858–131876. doi: https://doi.org/10.1109/access.2021.3112535
- Khare, S. K., Blanes-Vidal, V., Nadimi, E. S., Acharya, U. R. (2024). Emotion recognition and artificial intelligence: A systematic review (2014–2023) and research recommendations. Information Fusion, 102, 102019. doi: https://doi.org/10.1016/j.inffus.2023.102019
- Jwaid, W. M., Al-Husseini, Z. S. M., Sabry, A. H. (2021). Development of brain tumor segmentation of magnetic resonance imaging (MRI) using U-Net deep learning. Eastern-European Journal of Enterprise Technologies, 4 (9 (112)), 23–31. doi: https://doi.org/10.15587/1729-4061.2021.238957
- Lu, X. (2022). Deep Learning Based Emotion Recognition and Visualization of Figural Representation. Frontiers in Psychology, 12. doi: https://doi.org/10.3389/fpsyg.2021.818833
- Ahmed, N., Aghbari, Z. A., Girija, S. (2023). A systematic survey on multimodal emotion recognition using learning algorithms. Intelligent Systems with Applications, 17, 200171. doi: https://doi.org/10.1016/j.iswa.2022.200171
- Nosov, P., Zinchenko, S., Ben, A., Prokopchuk, Y., Mamenko, P., Popovych, I. et al. (2021). Navigation safety control system development through navigator action prediction by data mining means. Eastern-European Journal of Enterprise Technologies, 2 (9 (110)), 55–68. doi: https://doi.org/10.15587/1729-4061.2021.229237
- de Lope, J., Graña, M. (2023). An ongoing review of speech emotion recognition. Neurocomputing, 528, 1–11. doi: https://doi.org/10.1016/j.neucom.2023.01.002
- Costantini, G., Parada-Cabaleiro, E., Casali, D., Cesarini, V. (2022). The Emotion Probe: On the Universality of Cross-Linguistic and Cross-Gender Speech Emotion Recognition via Machine Learning. Sensors, 22 (7), 2461. doi: https://doi.org/10.3390/s22072461
- Chen, P., Wu, L., Wang, L. (2023). AI Fairness in Data Management and Analytics: A Review on Challenges, Methodologies and Applications. Applied Sciences, 13 (18), 10258. doi: https://doi.org/10.3390/app131810258
- Bankins, S., Formosa, P. (2023). The Ethical Implications of Artificial Intelligence (AI) For Meaningful Work. Journal of Business Ethics, 185 (4), 725–740. doi: https://doi.org/10.1007/s10551-023-05339-7
- Mahdi, Q. A., Shyshatskyi, A., Prokopenko, Y., Ivakhnenko, T., Kupriyenko, D., Golian, V. et al. (2021). Development of estimation and forecasting method in intelligent decision support systems. Eastern-European Journal of Enterprise Technologies, 3 (9 (111)), 51–62. doi: https://doi.org/10.15587/1729-4061.2021.232718
- Cai, Y., Li, X., Li, J. (2023). Emotion Recognition Using Different Sensors, Emotion Models, Methods and Datasets: A Comprehensive Review. Sensors, 23 (5), 2455. doi: https://doi.org/10.3390/s23052455
- Coretta, S., Casillas, J. V., Roettger, T. B. (2022). Multidimensional signals and analytic flexibility: Estimating degrees of freedom in human speech analyses. doi: https://doi.org/10.31234/osf.io/q8t2k
- Vakkantula, P. C. (2020). Speech Mode Classification using the Fusion of CNNs and LSTM Networks. West Virginia University. doi: https://doi.org/10.33915/etd.7845
- Brownlee, J. (2021). Gentle Introduction to the Adam Optimization Algorithm for Deep Learning. Machine Learning Mastery. Available at: https://machinelearningmastery.com/adam-optimization-algorithm-for-deep-learning/
- Hashem, A., Arif, M., Alghamdi, M. (2023). Speech emotion recognition approaches: A systematic review. Speech Communication, 154, 102974. doi: https://doi.org/10.1016/j.specom.2023.102974
- Chauhan, C., Parida, V., Dhir, A. (2022). Linking circular economy and digitalisation technologies: A systematic literature review of past achievements and future promises. Technological Forecasting and Social Change, 177, 121508. doi: https://doi.org/10.1016/j.techfore.2022.121508
- Kamath, U., Liu, J., Whitaker, J. (2019). Deep Learning for NLP and Speech Recognition. Springer International Publishing. doi: https://doi.org/10.1007/978-3-030-14596-5
- Ekman, P. (1971). Universals and cultural differences in facial expressions of emotion. Nebraska Symposium on Motivation, 19, 207–283.
- Rabiner, L. R., Schafer, R. W. (2007). Introduction to Digital Speech Processing. Foundations and Trends® in Signal Processing, 1 (1-2), 1–194. doi: https://doi.org/10.1561/2000000001
- Livingstone, S. R., Russo, F. A. (2018). The Ryerson Audio-Visual Database of Emotional Speech and Song (RAVDESS): A dynamic, multimodal set of facial and vocal expressions in North American English. PLOS ONE, 13 (5), e0196391. doi: https://doi.org/10.1371/journal.pone.0196391
- Haq, S., Jackson, P. J. B. (2009). Speaker-Dependent Audio-Visual Emotion Recognition. In Proc. Int'l Conf. on Auditory-Visual Speech Processing, 53–58.
- Pichora-Fuller, M. K., Dupuis, K. (2020). Toronto emotional speech set (TESS). doi: https://doi.org/10.5683/SP2/E8H2MF
- Zhou, K., Sisman, B., Liu, R., Li, H. (2021). Seen and Unseen Emotional Style Transfer for Voice Conversion with A New Emotional Speech Dataset. ICASSP 2021 - 2021 IEEE International Conference on Acoustics, Speech and Signal Processing (ICASSP). doi: https://doi.org/10.1109/icassp39728.2021.9413391
- Zwicker, E., Fastl, H. (1999). Psychoacoustics. Facts and Models. Springer-Verlag, 417. doi: https://doi.org/10.1007/978-3-662-09562-1
- Davis, S., Mermelstein, P. (1980). Comparison of parametric representations for monosyllabic word recognition in continuously spoken sentences. IEEE Transactions on Acoustics, Speech, and Signal Processing, 28 (4), 357–366. doi: https://doi.org/10.1109/tassp.1980.1163420
- Goodfellow, I., Bengio, Y., Courville, A. (2016). Deep Learning. MIT Press. Available at: https://www.deeplearningbook.org/
- Sundgren, D., Rahmani, R., Larsson, A., Moran, A., Bonet, I. (2015). Speech emotion recognition in emotional feedback for Human-Robot Interaction. International Journal of Advanced Research in Artificial Intelligence, 4 (2). doi: https://doi.org/10.14569/ijarai.2015.040204
- Martin, O., Kotsia, I., Macq, B., Pitas, I. (2006). The eNTERFACE' 05 Audio-Visual Emotion Database. 22nd International Conference on Data Engineering Workshops (ICDEW’06). doi: https://doi.org/10.1109/icdew.2006.145
- Koshekov, К. Т., Savostin, А. А., Seidakhmetov, B. K., Anayatova, R. K., Fedorov, I. O. (2021). Aviation Profiling Method Based on Deep Learning Technology for Emotion Recognition by Speech Signal. Transport and Telecommunication Journal, 22 (4), 471–481. doi: https://doi.org/10.2478/ttj-2021-0037
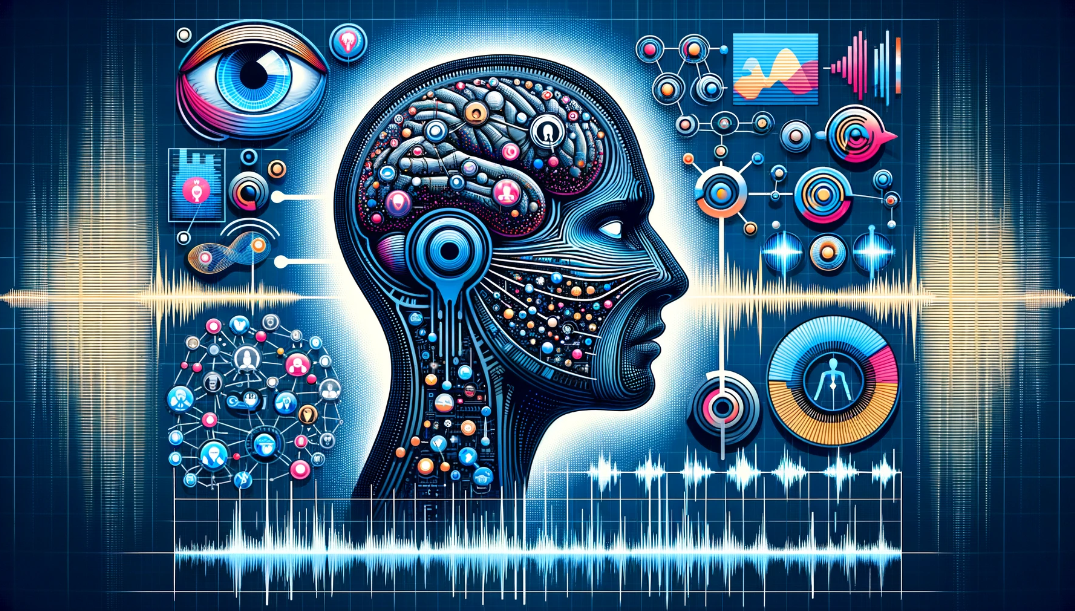
##submission.downloads##
Опубліковано
Як цитувати
Номер
Розділ
Ліцензія
Авторське право (c) 2023 Zharas Ainakulov, Kayrat Koshekov, Alexey Savostin, Raziyam Anayatova, Beken Seidakhmetov, Gulzhan Kurmankulova

Ця робота ліцензується відповідно до Creative Commons Attribution 4.0 International License.
Закріплення та умови передачі авторських прав (ідентифікація авторства) здійснюється у Ліцензійному договорі. Зокрема, автори залишають за собою право на авторство свого рукопису та передають журналу право першої публікації цієї роботи на умовах ліцензії Creative Commons CC BY. При цьому вони мають право укладати самостійно додаткові угоди, що стосуються неексклюзивного поширення роботи у тому вигляді, в якому вона була опублікована цим журналом, але за умови збереження посилання на першу публікацію статті в цьому журналі.
Ліцензійний договір – це документ, в якому автор гарантує, що володіє усіма авторськими правами на твір (рукопис, статтю, тощо).
Автори, підписуючи Ліцензійний договір з ПП «ТЕХНОЛОГІЧНИЙ ЦЕНТР», мають усі права на подальше використання свого твору за умови посилання на наше видання, в якому твір опублікований. Відповідно до умов Ліцензійного договору, Видавець ПП «ТЕХНОЛОГІЧНИЙ ЦЕНТР» не забирає ваші авторські права та отримує від авторів дозвіл на використання та розповсюдження публікації через світові наукові ресурси (власні електронні ресурси, наукометричні бази даних, репозитарії, бібліотеки тощо).
За відсутності підписаного Ліцензійного договору або за відсутністю вказаних в цьому договорі ідентифікаторів, що дають змогу ідентифікувати особу автора, редакція не має права працювати з рукописом.
Важливо пам’ятати, що існує і інший тип угоди між авторами та видавцями – коли авторські права передаються від авторів до видавця. В такому разі автори втрачають права власності на свій твір та не можуть його використовувати в будь-який спосіб.