Розробка моделі асоціативних правил з використанням машинного навчання для визначення звичок користувачів соціальних мереж
DOI:
https://doi.org/10.15587/1729-4061.2024.305116Ключові слова:
звички, поведінка, текстові дані, асоціації, машинне навчання, соціальні мережіАнотація
Об'єктом дослідження є звички користувачів соціальних мереж. Проблема дослідження полягає в тому, що на платформах соціальних мереж існує велика кількість даних – від даних зображень, текстових даних до аудіоданих, що ускладнює визначення моделей поведінки, певних інтересів та звичок користувачів. У дослідженні вивчалися розрізнені текстові дані, оскільки текстові дані не були ретельно проаналізовані з точки зору структури слів, що приховує багато інформації та ускладнює проведення аналізу для визначення шаблонів та поведінки користувачів у соціальних мережах. Результати, отримані в даному дослідженні представлені у вигляді аналізу та моделей, що дозволяють визначити моделі поведінки користувачів у соціальних мережах за допомогою асоціативних правил та методів машинного навчання. При застосуванні методу асоціацій використовується апріорний алгоритм, який у процесі розбиває всі текстові дані на набори елементів для визначення звичок користувачів соціальних мереж, а в процесі машинного навчання відбувається процес формування моделі для порівняння результатів. Процес визначення звичок користувачів складається з декількох етапів, таких як очищення даних, зміна неструктурованих даних на структуровані та етап асоціативних правил за допомогою апріорного алгоритму, що застосовується до даних соціальних мереж для визначення взаємозв'язку між словами. Після цього застосовується машинне навчання, що дозволяє провести порівняння та визначити взаємозв'язки між словами. Це нове дослідження зі створення моделі для визначення поведінки користувачів за допомогою асоціативних правил та машинного навчання, яка може бути використана для відображення позитивної, негативної або нейтральної поведінки користувачів
Посилання
- El Rahman, S. A., AlOtaibi, F. A., AlShehri, W. A. (2019). Sentiment Analysis of Twitter Data. 2019 International Conference on Computer and Information Sciences (ICCIS). https://doi.org/10.1109/iccisci.2019.8716464
- Kumar, S., Khan, M. B., Hasanat, M. H. A., Saudagar, A. K. J., AlTameem, A., AlKhathami, M. (2022). An Anomaly Detection Framework for Twitter Data. Applied Sciences, 12 (21), 11059. https://doi.org/10.3390/app122111059
- Lubis, A. R., Prayudani, S., Lubis, M., Nugroho, O. (2022). Sentiment Analysis on Online Learning During the Covid-19 Pandemic Based on Opinions on Twitter using KNN Method. 2022 1st International Conference on Information System & Information Technology (ICISIT). https://doi.org/10.1109/icisit54091.2022.9872926
- Lubis, A. R., Nasution, M. K. M., Sitompul, O. S., Zamzami, E. M. (2023). A new approach to achieve the users’ habitual opportunities on social media. IAES International Journal of Artificial Intelligence (IJ-AI), 12 (1), 41. https://doi.org/10.11591/ijai.v12.i1.pp41-47
- Thakur, N. (2022). Twitter Big Data as a Resource for Exoskeleton Research: A Large-Scale Dataset of about 140,000 Tweets from 2017–2022 and 100 Research Questions. Analytics, 1 (2), 72–97. https://doi.org/10.3390/analytics1020007
- Schmidt, S., Zorenböhmer, C., Arifi, D., Resch, B. (2023). Polarity-Based Sentiment Analysis of Georeferenced Tweets Related to the 2022 Twitter Acquisition. Information, 14 (2), 71. https://doi.org/10.3390/info14020071
- Firdaniza, F., Ruchjana, B., Chaerani, D., Radianti, J. (2021). Information Diffusion Model in Twitter: A Systematic Literature Review. Information, 13 (1), 13. https://doi.org/10.3390/info13010013
- Kwon, J.-H., Kim, S., Lee, Y.-K., Ryu, K. (2021). Characteristics of Social Media Content and Their Effects on Restaurant Patrons. Sustainability, 13 (2), 907. https://doi.org/10.3390/su13020907
- Wibowo, A., Chen, S.-C., Wiangin, U., Ma, Y., Ruangkanjanases, A. (2020). Customer Behavior as an Outcome of Social Media Marketing: The Role of Social Media Marketing Activity and Customer Experience. Sustainability, 13 (1), 189. https://doi.org/10.3390/su13010189
- Ali Hakami, N., Hosni Mahmoud, H. A. (2022). The Prediction of Consumer Behavior from Social Media Activities. Behavioral Sciences, 12 (8), 284. https://doi.org/10.3390/bs12080284
- Gupta, V., Jung, K., Yoo, S.-C. (2020). Exploring the Power of Multimodal Features for Predicting the Popularity of Social Media Image in a Tourist Destination. Multimodal Technologies and Interaction, 4 (3), 64. https://doi.org/10.3390/mti4030064
- Khafaga, D. S., Alharbi, A. H., Mohamed, I., Hosny, K. M. (2022). An Integrated Classification and Association Rule Technique for Early-Stage Diabetes Risk Prediction. Healthcare, 10 (10), 2070. https://doi.org/10.3390/healthcare10102070
- Orama, J. A., Borràs, J., Moreno, A. (2021). Combining Cluster-Based Profiling Based on Social Media Features and Association Rule Mining for Personalised Recommendations of Touristic Activities. Applied Sciences, 11 (14), 6512. https://doi.org/10.3390/app11146512
- Lee, S., Cha, Y., Han, S., Hyun, C. (2019). Application of Association Rule Mining and Social Network Analysis for Understanding Causality of Construction Defects. Sustainability, 11 (3), 618. https://doi.org/10.3390/su11030618
- Kruse, R., Lokukatagoda, T., Alkhushayni, S. (2022). A framework for association rule learning with social media networks. IOP SciNotes, 3 (1), 015001. https://doi.org/10.1088/2633-1357/abe9be
- Diaz-Garcia, J. A., Ruiz, M. D., Martin-Bautista, M. J. (2022). A survey on the use of association rules mining techniques in textual social media. Artificial Intelligence Review, 56 (2), 1175–1200. https://doi.org/10.1007/s10462-022-10196-3
- Shazad, B., Ullah khan, H., Rehman, Z., Farooq, M., Mahmood, A., Mehmood, I. et al. (2019). Finding Temporal Influential Users in Social Media Using Association Rule Learning. Intelligent Automation and Soft Computing. https://doi.org/10.31209/2019.100000130
- Zhang, J., Marino, C., Canale, N., Charrier, L., Lazzeri, G., Nardone, P., Vieno, A. (2022). The Effect of Problematic Social Media Use on Happiness among Adolescents: The Mediating Role of Lifestyle Habits. International Journal of Environmental Research and Public Health, 19 (5), 2576. https://doi.org/10.3390/ijerph19052576
- Zingla, M. A., Ettaleb, M., Latiri, C. C., Slimani, Y. (2014). INEX2014: Tweet Contextualization Using Association Rules between Terms. Working Notes for CLEF 2014 Conference, 574–584. Available at: https://ceur-ws.org/Vol-1180/CLEF2014wn-Inex-ZinglaEt2014.pdf
- Koukaras, P., Tjortjis, C., Rousidis, D. (2022). Mining association rules from COVID-19 related twitter data to discover word patterns, topics and inferences. Information Systems, 109, 102054. https://doi.org/10.1016/j.is.2022.102054
- Güven, Z. A., Diri, B., Çakaloğlu, T. (2018). Classification of TurkishTweet emotions by n- stage Latent Dirichlet Allocation. 2018 Electric Electronics, Computer Science, Biomedical Engineerings’ Meeting (EBBT). https://doi.org/10.1109/ebbt.2018.8391454
- Drias, Y., Drias, H. (2021). Sentiment Evolution Analysis and Association Rule Mining for COVID-19 Tweets. Journal of Digital Art & Humanities, 2 (2), 3–21. https://doi.org/10.33847/2712-8148.2.2_1
- Lian, S., Gao, J., Li, H. (2018). A Method of Mining Association Rules for Geographical Points of Interest. ISPRS International Journal of Geo-Information, 7 (4), 146. https://doi.org/10.3390/ijgi7040146
- Kusumaningrum, R., Wiedjayanto, M. I. A., Adhy, S., Suryono (2016). Classification of Indonesian news articles based on Latent Dirichlet Allocation. 2016 International Conference on Data and Software Engineering (ICoDSE). https://doi.org/10.1109/icodse.2016.7936106
- Ranjan, R., Kumar, S. S. (2022). User behaviour analysis using data analytics and machine learning to predict malicious user versus legitimate user. High-Confidence Computing, 2 (1), 100034. https://doi.org/10.1016/j.hcc.2021.100034
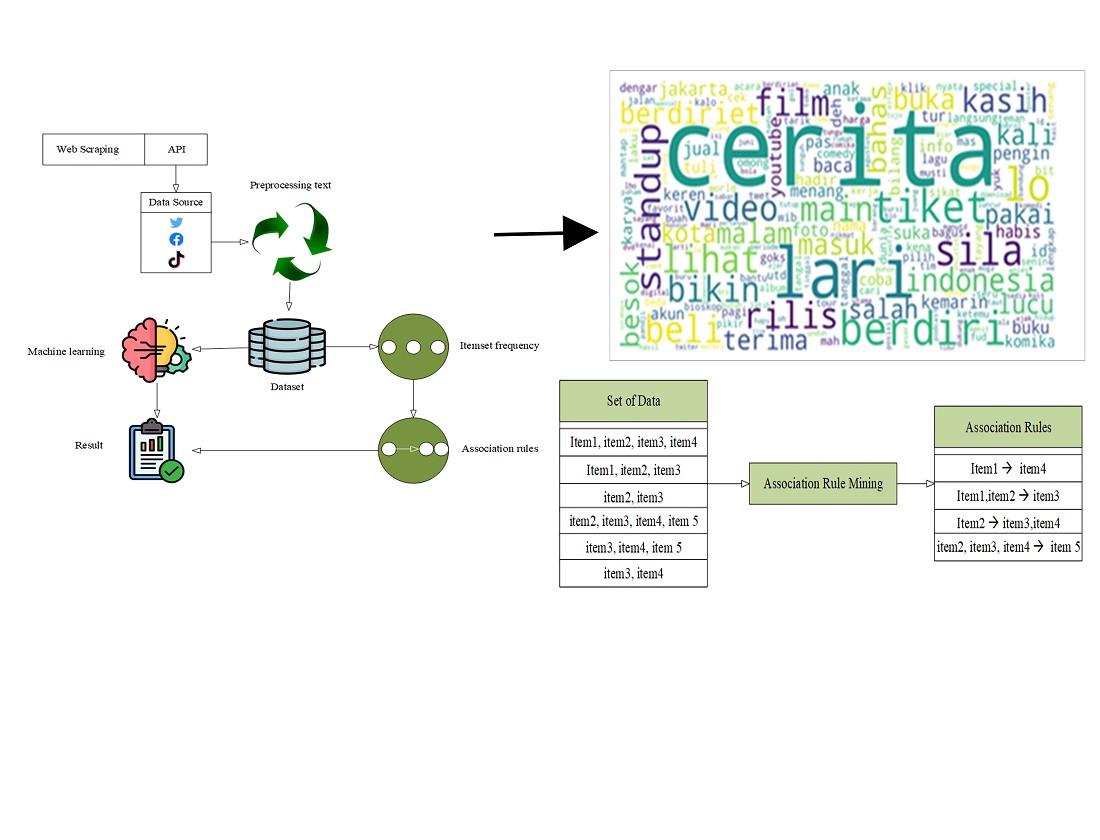
##submission.downloads##
Опубліковано
Як цитувати
Номер
Розділ
Ліцензія
Авторське право (c) 2024 Antoni, Mahrani Arfah, Ferry Fachrizal, Okvi Nugroho

Ця робота ліцензується відповідно до Creative Commons Attribution 4.0 International License.
Закріплення та умови передачі авторських прав (ідентифікація авторства) здійснюється у Ліцензійному договорі. Зокрема, автори залишають за собою право на авторство свого рукопису та передають журналу право першої публікації цієї роботи на умовах ліцензії Creative Commons CC BY. При цьому вони мають право укладати самостійно додаткові угоди, що стосуються неексклюзивного поширення роботи у тому вигляді, в якому вона була опублікована цим журналом, але за умови збереження посилання на першу публікацію статті в цьому журналі.
Ліцензійний договір – це документ, в якому автор гарантує, що володіє усіма авторськими правами на твір (рукопис, статтю, тощо).
Автори, підписуючи Ліцензійний договір з ПП «ТЕХНОЛОГІЧНИЙ ЦЕНТР», мають усі права на подальше використання свого твору за умови посилання на наше видання, в якому твір опублікований. Відповідно до умов Ліцензійного договору, Видавець ПП «ТЕХНОЛОГІЧНИЙ ЦЕНТР» не забирає ваші авторські права та отримує від авторів дозвіл на використання та розповсюдження публікації через світові наукові ресурси (власні електронні ресурси, наукометричні бази даних, репозитарії, бібліотеки тощо).
За відсутності підписаного Ліцензійного договору або за відсутністю вказаних в цьому договорі ідентифікаторів, що дають змогу ідентифікувати особу автора, редакція не має права працювати з рукописом.
Важливо пам’ятати, що існує і інший тип угоди між авторами та видавцями – коли авторські права передаються від авторів до видавця. В такому разі автори втрачають права власності на свій твір та не можуть його використовувати в будь-який спосіб.