Розробка алгоритму стиснення аерокосмічних зображень для подальшого розпізнавання та ідентифікації різних об'єктів
DOI:
https://doi.org/10.15587/1729-4061.2024.306973Ключові слова:
вейвлет-перетворення, вейвлет-функція Хаара, стиснення, гіперспектральні аерокосмічні зображення, алгоритм стиснення, Дистанційне зондуванняАнотація
Об'єктом дослідження є розпізнавання та ідентифікація різних об'єктів на аерокосмічних знімках. Для вирішення задач стиснення гіперспектральних аерокосмічних зображень з втратами пропонується розробка алгоритму стиснення. В результаті був розроблений алгоритм стиснення аерокосмічних зображень для подальшого розпізнавання та ідентифікації різних об'єктів з використанням вейвлет-перетворення для обробки космічних знімків високого і середнього дозволу при моніторингу з супутників дистанційного зондування Землі, заснований на використанні структурних особливостей зображень об'єктів. Зокрема, представлені ортогональне і вейвлет-перетворення, адаптовані для стиснення гіперспектральних аерокосмічних зображень з втратами, представлений адаптивний алгоритм дискретного косинусного перетворення з подальшим квантуванням з рівнем втрат і стисненням. Завдяки серії експериментів на гіперспектральних аерокосмічних знімках можна виділити ефективність запропонованого алгоритму з точки зору ступеня стиснення, а також характеристики меж його застосовності. Використання вейвлетів забезпечує прогресивне стиснення бітового потоку, що дозволяє досягти стиснення без втрат з мінімальною втратою інформації за рахунок модифікованого алгоритму Хаффмана зі ступенем стиснення 9, більш ніж в 2,5 рази в порівнянні з існуючими алгоритмами, а за показником якості відновлених зображень пікове відношення сигнал/шум істотно нижче 32,56.
Розроблений алгоритм стиснення демонструє ефективність свого застосування по набору характеристик і перевершує аналоги. Областю застосування та умовами практичного використання отриманих результатів є порівняння запропонованого алгоритму з результатами експериментів, отриманими для універсальних алгоритмів стиснення для архіваторів і компресора
Посилання
- Mallat, S. (1999). A Wavelet Tour of Signal Processing. Academic Press. https://doi.org/10.1016/b978-0-12-466606-1.x5000-4
- Salomon, D. (2007). Data compression: The complete reference. Springer, 1092.
- Ceamanos, X., Valero, S. (2016). Processing Hyperspectral Images. Optical Remote Sensing of Land Surface, 163–200. https://doi.org/10.1016/b978-1-78548-102-4.50004-1
- Xue, J., Zhao, Y., Liao, W., Chan, J. C.-W. (2019). Hyper-Laplacian regularized nonlocal low-rank matrix recovery for hyperspectral image compressive sensing reconstruction. Information Sciences, 501, 406–420. https://doi.org/10.1016/j.ins.2019.06.012
- Prabhakar, T. V. N., Geetha, P. (2017). Two-dimensional empirical wavelet transform based supervised hyperspectral image classification. ISPRS Journal of Photogrammetry and Remote Sensing, 133, 37–45. https://doi.org/10.1016/j.isprsjprs.2017.09.003
- Pranitha, K., Kavya, G. (2023). An efficient image compression architecture based on optimized 9/7 wavelet transform with hybrid post processing and entropy encoder module. Microprocessors and Microsystems, 98, 104821. https://doi.org/10.1016/j.micpro.2023.104821
- Shi, C., Zhang, J., Zhang, Y. (2016). Content-based onboard compression for remote sensing images. Neurocomputing, 191, 330–340. https://doi.org/10.1016/j.neucom.2016.01.048
- Puri, A., Sharifahmadian, E., Latifi, S. (2014). A Comparison of Hyperspectral Image Compression Methods. International Journal of Computer and Electrical Engineering, 6 (6), 493–500. https://doi.org/10.17706/ijcee.2014.v6.867
- Lin, H.-C., Hwang, Y.-T. (2011). Lossless Compression of Hyperspectral Images Using Adaptive Prediction and Backward Search Schemes. Journal of Information Science and Engineering, 27, 419–435. Available at: https://www.researchgate.net/publication/220588090_Lossless_Compression_of_Hyperspectral_Images_Using_Adaptive_Prediction_and_Backward_Search_Schemes
- Mora Pascual, J., Mora Mora, H., Fuster Guilló, A., Azorín López, J. (2015). Adjustable compression method for still JPEG images. Signal Processing: Image Communication, 32, 16–32. https://doi.org/10.1016/j.image.2015.01.004
- KazEOSat-1. Available at: https://www.eoportal.org/satellite-missions/kazeosat-1
- Nian, Y., He, M., Wan, J. (2015). Lossless and near-lossless compression of hyperspectral images based on distributed source coding. Journal of Visual Communication and Image Representation, 28, 113–119. https://doi.org/10.1016/j.jvcir.2014.06.008
- Cheng, K., Dill, J. (2013). Hyperspectral images lossless compression using the 3D binary EZW algorithm. Image Processing: Algorithms and Systems XI. https://doi.org/10.1117/12.2002820
- Li, C., Guo, K. (2014). Lossless Compression of Hyperspectral Images Using Three-Stage Prediction with Adaptive Search Threshold. International Journal of Signal Processing, Image Processing and Pattern Recognition, 7 (3), 305–316. https://doi.org/10.14257/ijsip.2014.7.3.25
- Gashnikov, M., Glumov, N. (2016). Onboard processing of hyperspectral data in the remote sensing systems based on hierarchical compression. Computer Optics, 40 (4), 543–551. https://doi.org/10.18287/2412-6179-2016-40-4-543-551
- Gashnikov, M. (2017). Minimizing the entropy of post-interpolation residuals for image compression based on hierarchical grid interpolation. Computer Optics, 41 (2), 266–275. https://doi.org/10.18287/2412-6179-2017-41-2-266-275
- Sarinova, A., Zamyatin, A., Cabral, P. (2015). Lossless compression of hyperspectral images with pre-byte processing and intra-bands correlation. DYNA, 82 (190), 166–172. https://doi.org/10.15446/dyna.v82n190.43723
- Zamjatin, A. V., Sarinova, A. Zh. (2017). Algorithm for compressing hyperspectral aerospace images using mathematical processing and taking into account interband correlation. Materials of the IV International Scientific Conference "Regional Problems of Earth Remote Sensing", 157–160.
- Dubey, V. A., Dubey, R. (2013). A New Set Partitioning in Hierarchical (SPIHT) Algorithm and Analysis with Wavelet Filters. International Journal of Innovative Technology and Exploring Engineering, 3 (3), 125–128. Available at: https://www.ijitee.org/wp-content/uploads/papers/v3i3/C1132083313.pdf
- Kiely, A., Klimesh, M., Xie, H., Aranki, N. (2006). ICER-3D: A Progressive Wavelet-Based Compressor for Hyperspectral Images. The Interplanetary Network Progress Report.
- Sindhuja, N. M., Arumugam, A. S. (2013). SPIHT based compression of hyper spectral images. International Journal of Advanced Research in Electrical, Electronics and Instrumentation Engineering, 2 (10), 4933–4938. Available at: https://www.ijareeie.com/upload/2013/october/26SPIHT.pdf
- Penna, B., Tillo, T., Magli, E., Olmo, G. (2006). Progressive 3-D Coding of Hyperspectral Images Based on JPEG 2000. IEEE Geoscience and Remote Sensing Letters, 3 (1), 125–129. https://doi.org/10.1109/lgrs.2005.859942
- Sujithra, D. S., Manickam, T., Sudheer, D. S. (2013). Compression of hyperspectral image using discrete wavelet transform and Walsh Hadamard transform. International journal of advanced research in electronics and communication engineering (IJARECE), 2 (3), 314–319.
- Pizzolante, R., Carpentieri, B. (2013). On the Compression of Hyperspectral Data. IT CoNvergence PRActice (INPRA), 1 (4), 24–38. Available at: https://isyou.info/inpra/papers/inpra-v1n4-02.pdf
- Christophe, E. (2011). Hyperspectral Data Compression Tradeoff. Optical Remote Sensing, 9–29. https://doi.org/10.1007/978-3-642-14212-3_2
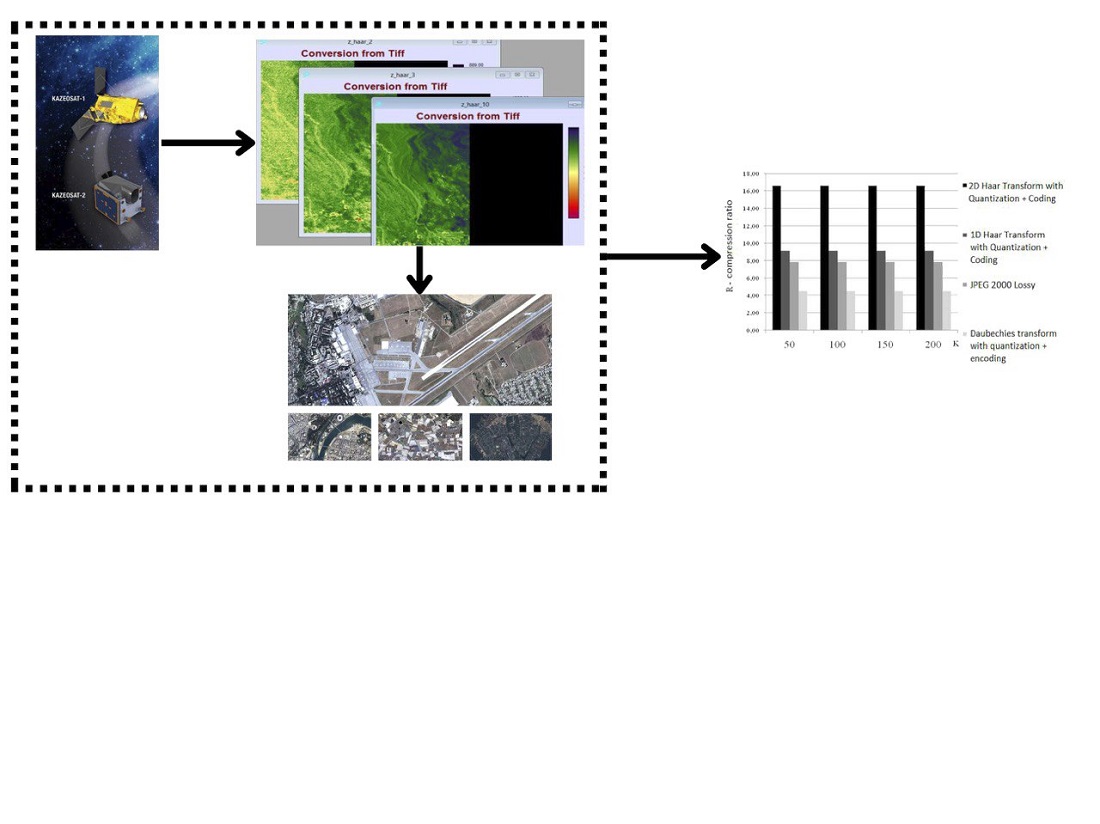
##submission.downloads##
Опубліковано
Як цитувати
Номер
Розділ
Ліцензія
Авторське право (c) 2024 Assiya Sarinova, Alexandr Neftissov, Leyla Rzayeva, Alimzhan Yessenov, Lalita Kirichenko, Ilyas Kazambayev

Ця робота ліцензується відповідно до Creative Commons Attribution 4.0 International License.
Закріплення та умови передачі авторських прав (ідентифікація авторства) здійснюється у Ліцензійному договорі. Зокрема, автори залишають за собою право на авторство свого рукопису та передають журналу право першої публікації цієї роботи на умовах ліцензії Creative Commons CC BY. При цьому вони мають право укладати самостійно додаткові угоди, що стосуються неексклюзивного поширення роботи у тому вигляді, в якому вона була опублікована цим журналом, але за умови збереження посилання на першу публікацію статті в цьому журналі.
Ліцензійний договір – це документ, в якому автор гарантує, що володіє усіма авторськими правами на твір (рукопис, статтю, тощо).
Автори, підписуючи Ліцензійний договір з ПП «ТЕХНОЛОГІЧНИЙ ЦЕНТР», мають усі права на подальше використання свого твору за умови посилання на наше видання, в якому твір опублікований. Відповідно до умов Ліцензійного договору, Видавець ПП «ТЕХНОЛОГІЧНИЙ ЦЕНТР» не забирає ваші авторські права та отримує від авторів дозвіл на використання та розповсюдження публікації через світові наукові ресурси (власні електронні ресурси, наукометричні бази даних, репозитарії, бібліотеки тощо).
За відсутності підписаного Ліцензійного договору або за відсутністю вказаних в цьому договорі ідентифікаторів, що дають змогу ідентифікувати особу автора, редакція не має права працювати з рукописом.
Важливо пам’ятати, що існує і інший тип угоди між авторами та видавцями – коли авторські права передаються від авторів до видавця. В такому разі автори втрачають права власності на свій твір та не можуть його використовувати в будь-який спосіб.