Experimental investigation and modelling of residual stresses in face milling of Al-6061-T3 using neural network
DOI:
https://doi.org/10.15587/1729-4061.2022.267032Keywords:
face milling, X-ray diffraction (XRD), residual stress (RS), aluminum alloy (AA 6061-T3), artificial neural network (ANN)Abstract
Milling process is a common machining operation that is used in the manufacturing of complex surfaces. Machining-induced residual stresses (RS) have a great impact on the performance of machined components and the surface quality in face milling operations with parameter cutting. The properties of engineering material as well as structural components, specifically fatigue life, deformation, impact resistance, corrosion resistance, and brittle fracture, can all be significantly influenced by residual stresses. Accordingly, controlling the distribution of residual stresses is indeed important to protect the piece and avoid failure. Most of the previous works inspected the material properties, tool parameters, or cutting parameters, but few of them provided the distribution of RS in a direct and singular way. This work focuses on studying and optimizing the effect of cutting speed, feed rate, and depth of cut for 6061-T3 aluminum alloy on the RS of the surface. The optimum values of geometry parameters have been found by using the L27 orthogonal array. Analysis and simulation of RS by using an artificial neural network (ANN) were carried out to predict the RS behavior due to changing machining process parameters. Using ANN to predict the behavior of RS due to changing machining process parameters is presented as a promising method. The milling process produces more RS at high cutting speed, roughly intermediate feed rate, and deeper cut, according to the results. The best residual stress obtained from ANN is ‒135.204 N/mm2 at a cutting depth of 5 mm, feed rate of 0.25 mm/rev and cutting speed of 1,000 rpm. ANN can be considered a powerful tool for estimating residual stress
Supporting Agency
- We sincerely thank the Al-Khwarizmi College of Engineering and the mechanical applied Laboratory of the Department of Automated Manufacturing Engineering for their assistance in carrying out this work.
References
- Treuting, R. G., Read, W. T. (1951). A Mechanical Determination of Biaxial Residual Stress in Sheet Materials. Journal of Applied Physics, 22 (2), 130–134. doi: https://doi.org/10.1063/1.1699913
- Lucca, D. A., Brinksmeier, E., Goch, G. (1998). Progress in Assessing Surface and Subsurface Integrity. CIRP Annals, 47 (2), 669–693. doi: https://doi.org/10.1016/s0007-8506(07)63248-x
- Tang, Z. T., Liu, Z. Q., Pan, Y. Z., Wan, Y., Ai, X. (2009). The influence of tool flank wear on residual stresses induced by milling aluminum alloy. Journal of Materials Processing Technology, 209 (9), 4502–4508. doi: https://doi.org/10.1016/j.jmatprotec.2008.10.034
- Masmiati, N., Sarhan, A. A. D., Hassan, M. A. N., Hamdi, M. (2016). Optimization of cutting conditions for minimum residual stress, cutting force and surface roughness in end milling of S50C medium carbon steel. Measurement, 86, 253–265. doi: https://doi.org/10.1016/j.measurement.2016.02.049
- Wu, Q., Li, D.-P., Zhang, Y.-D. (2016). Detecting Milling Deformation in 7075 Aluminum Alloy Aeronautical Monolithic Components Using the Quasi-Symmetric Machining Method. Metals, 6 (4), 80. doi: https://doi.org/10.3390/met6040080
- Hlembotska, L., Melnychuk, P., Balytska, N., Melnyk, O. (2018). Modelling the loading of the nose-free cutting edges of face mill with a spiral-stepped arrangement of inserts. Eastern-European Journal of Enterprise Technologies, 1 (1 (91)), 46–54. doi: https://doi.org/10.15587/1729-4061.2018.121712
- Huang, X., Sun, J., Li, J. (2015). Experimental investigation of the effect of tool geometry on residual stresses in high speed milling 7050-T7451 aluminium alloy. International Journal of Surface Science and Engineering, 9 (4), 359. doi: https://doi.org/10.1504/ijsurfse.2015.070813
- Mia, M., Bashir, M. A., Khan, M. A., Dhar, N. R. (2016). Optimization of MQL flow rate for minimum cutting force and surface roughness in end milling of hardened steel (HRC 40). The International Journal of Advanced Manufacturing Technology, 89 (1-4), 675–690. doi: https://doi.org/10.1007/s00170-016-9080-8
- Mumali, F. (2022). Artificial neural network-based decision support systems in manufacturing processes: A systematic literature review. Computers & Industrial Engineering, 165, 107964. doi: https://doi.org/10.1016/j.cie.2022.107964
- Faizin, A., I Made Londen, B., Pramono, A. S., Wahjudi, A. (2021). Determination of the effect of thickness reduction ratio, die angle, and coefficient of friction on residual stresses in ironing process: an analysis using computer simulation. Eastern-European Journal of Enterprise Technologies, 5 (1 (113)), 70–78. doi: https://doi.org/10.15587/1729-4061.2021.243245
- Yao, C., Dou, X., Wu, D., Zhou, Z., Zhang, J. (2016). Surface integrity and fatigue analysis of shot-peening for 7055 aluminum alloy under different high-speed milling conditions. Advances in Mechanical Engineering, 8 (10), 168781401667462. doi: https://doi.org/10.1177/1687814016674628
- Jiang, X., Zhang, Z., Ding, Z., Fergani, O., Liang, S. Y. (2017). Tool overlap effect on redistributed residual stress and shape distortion produced by the machining of thin-walled aluminum parts. The International Journal of Advanced Manufacturing Technology, 93 (5-8), 2227–2242. doi: https://doi.org/10.1007/s00170-017-0693-3
- Ji, C., Sun, S., Lin, B., Fei, J. (2018). Effect of cutting parameters on the residual stress distribution generated by pocket milling of 2219 aluminum alloy. Advances in Mechanical Engineering, 10 (12), 168781401881305. doi: https://doi.org/10.1177/1687814018813055
- Perez, I., Madariaga, A., Cuesta, M., Garay, A., Arrazola, P. J., Ruiz, J. J. et al. (2018). Effect of cutting speed on the surface integrity of face milled 7050-T7451 aluminium workpieces. Procedia CIRP, 71, 460–465. doi: https://doi.org/10.1016/j.procir.2018.05.034
- Mohammadpour, M., Razfar, M. R., Jalili Saffar, R. (2010). Numerical investigating the effect of machining parameters on residual stresses in orthogonal cutting. Simulation Modelling Practice and Theory, 18 (3), 378–389. doi: https://doi.org/10.1016/j.simpat.2009.12.004
- Schajer, G. S. (1981). Application of Finite Element Calculations to Residual Stress Measurements. Journal of Engineering Materials and Technology, 103 (2), 157–163. doi: https://doi.org/10.1115/1.3224988
- Afazov, S. M., Becker, A. A., Hyde, T. H. (2012). Mathematical Modeling and Implementation of Residual Stress Mapping From Microscale to Macroscale Finite Element Models. Journal of Manufacturing Science and Engineering, 134 (2). doi: https://doi.org/10.1115/1.4006090
- Fuh, K.-H., Wu, C.-F. (1995). A residual-stress model for the milling of aluminum alloy (2014-T6). Journal of Materials Processing Technology, 51 (1-4), 87–105. doi: https://doi.org/10.1016/0924-0136(94)01355-5
- Zhou, R., Yang, W. (2019). Analytical modeling of machining-induced residual stresses in milling of complex surface. The International Journal of Advanced Manufacturing Technology, 105 (1-4), 565–577. doi: https://doi.org/10.1007/s00170-019-04219-7
- Huang, X., Sun, J., Li, J., Han, X., Xiong, Q. (2013). An Experimental Investigation of Residual Stresses in High-Speed End Milling 7050-T7451 Aluminum Alloy. Advances in Mechanical Engineering, 5, 592659. doi: https://doi.org/10.1155/2013/592659
- El-Axir, M. H. (2002). A method of modeling residual stress distribution in turning for different materials. International Journal of Machine Tools and Manufacture, 42 (9), 1055–1063. doi: https://doi.org/10.1016/s0890-6955(02)00031-7
- Cheng, M., Jiao, L., Yan, P., Feng, L., Qiu, T., Wang, X., Zhang, B. (2021). Prediction of surface residual stress in end milling with Gaussian process regression. Measurement, 178, 109333. doi: https://doi.org/10.1016/j.measurement.2021.109333
- Davis, J. R. (2001). Aluminum and Aluminum Alloys. ASM Internationa. Available at: https://materialsdata.nist.gov/bitstream/handle/11115/173/Aluminum%20and%20Aluminum%20Alloys%20Davis.pdf
- Standard test methods for tension testing wrought and cast aluminum- and magnesium-alloy products (Metric) (2015). ASTM International.
- Baden, A. S. (2017). Prediction the effect of milling parameters upon the residual stresses through using taghuchi method. Iraqi journal of mechanical and material engineering, 17 (2), 211–222. Available at: https://www.iasj.net/iasj/download/bdfade35166a8370
- Muñoz-Escalona, P., Maropoulos, P. G. (2015). A geometrical model for surface roughness prediction when face milling Al 7075-T7351 with square insert tools. Journal of Manufacturing Systems, 36, 216–223. doi: https://doi.org/10.1016/j.jmsy.2014.06.011
- Sova, O., Shyshatskyi, A., Zhuravskyi, Y., Salnikova, O., Zubov, O., Zhyvotovskyi, R. et al. (2020). Development of a methodology for training artificial neural networks for intelligent decision support systems. Eastern-European Journal of Enterprise Technologies, 2 (4 (104)), 6–14. doi: https://doi.org/10.15587/1729-4061.2020.199469
- Silva, D. P., Bastos, I. N., Fonseca, M. C. (2020). Influence of surface quality on residual stress of API 5L X80 steel submitted to static load and its prediction by artificial neural networks. The International Journal of Advanced Manufacturing Technology, 108 (11-12), 3753–3764. doi: https://doi.org/10.1007/s00170-020-05621-2
- Nouioua, M., Laouissi, A., Yallese, M. A., Khettabi, R., Belhadi, S. (2021). Multi-response optimization using artificial neural network-based GWO algorithm for high machining performance with minimum quantity lubrication. The International Journal of Advanced Manufacturing Technology, 116 (11-12), 3765–3778. doi: https://doi.org/10.1007/s00170-021-07745-5
- Jebaraj, M., Pradeep Kumar, M., Yuvaraj, N., Mujibar Rahman, G. (2019). Experimental study of the influence of the process parameters in the milling of Al6082-T6 alloy. Materials and Manufacturing Processes, 34 (12), 1411–1427. doi: https://doi.org/10.1080/10426914.2019.1594271
- Reimer, A., Luo, X. (2018). Prediction of residual stress in precision milling of AISI H13 steel. Procedia CIRP, 71, 329–334. doi: https://doi.org/10.1016/j.procir.2018.05.036
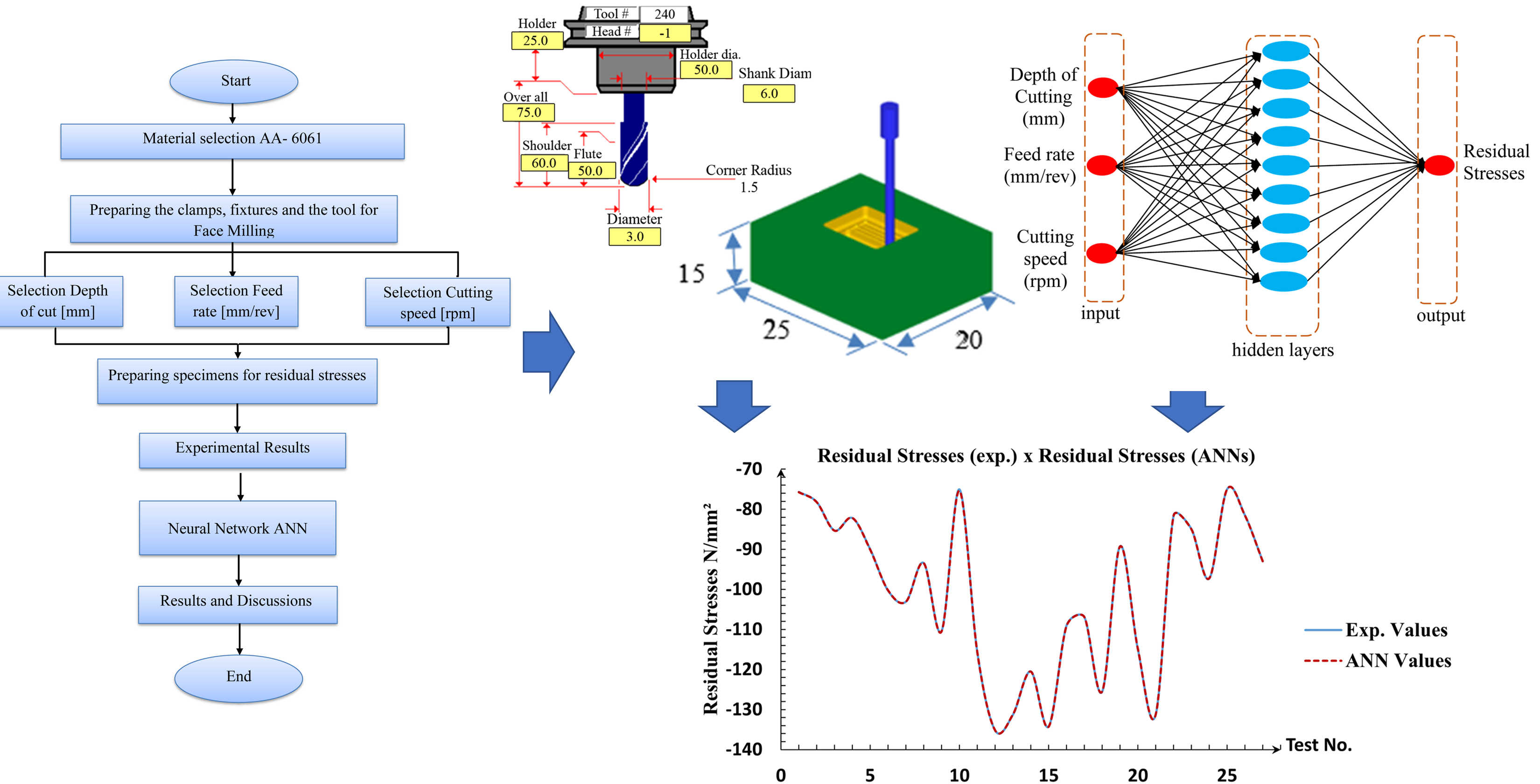
Downloads
Published
How to Cite
Issue
Section
License
Copyright (c) 2022 Basma L. Mahdi, Huda H. Dalef, Hiba K. Hussein

This work is licensed under a Creative Commons Attribution 4.0 International License.
The consolidation and conditions for the transfer of copyright (identification of authorship) is carried out in the License Agreement. In particular, the authors reserve the right to the authorship of their manuscript and transfer the first publication of this work to the journal under the terms of the Creative Commons CC BY license. At the same time, they have the right to conclude on their own additional agreements concerning the non-exclusive distribution of the work in the form in which it was published by this journal, but provided that the link to the first publication of the article in this journal is preserved.
A license agreement is a document in which the author warrants that he/she owns all copyright for the work (manuscript, article, etc.).
The authors, signing the License Agreement with TECHNOLOGY CENTER PC, have all rights to the further use of their work, provided that they link to our edition in which the work was published.
According to the terms of the License Agreement, the Publisher TECHNOLOGY CENTER PC does not take away your copyrights and receives permission from the authors to use and dissemination of the publication through the world's scientific resources (own electronic resources, scientometric databases, repositories, libraries, etc.).
In the absence of a signed License Agreement or in the absence of this agreement of identifiers allowing to identify the identity of the author, the editors have no right to work with the manuscript.
It is important to remember that there is another type of agreement between authors and publishers – when copyright is transferred from the authors to the publisher. In this case, the authors lose ownership of their work and may not use it in any way.