Determining the invariant of inter-frame processing for constructing the image similarity metric
DOI:
https://doi.org/10.15587/1729-4061.2023.276650Keywords:
digital image, color atlas, geographic information object, ontology, measure, similarity, hash function, diffeomorphism, persistent homologyAbstract
The relevance of modeling digital images is determined by the need to implement approaches in the study of localization and identification of objects in order to reduce the amount of data. In this paper, the study object is the topology of a discrete two-dimensional image within the framework of the problem of determining the invariants of diffeomorphic transformations. Geographic information objects (GIOs) refer to objects that are on a given surface or objects that locally change the surface. With regard to objects, it is assumed that the change in their geolocation in the process of forming both single images and an extended series of frames obtained in the process of continuous monitoring is insignificant. In the process of scanning the surface, possible changes in the position of the image source are taken into account, for example, such as yawing, rolling, and pitch in the case of unmanned aerial vehicles (UAVs). These maneuvers are represented as a group of diffeomorphisms that are controlled by the internal gyroscopes of the carrier and the external navigation system. Based on the studies reported here, the initial ontology of digital images (ODI) has been determined by using the model of color spaces and functions of a special kind. The presence of an ontology makes it possible to build an adequate topology of color distribution in the image and take into account the specificity of the distribution of different colors in a digital image. The study results indicate that a promising method is to determine the similarity by constructing a color atlas structure graph (CASG) based on ODI and by determining invariants as a fragment of CASG inherited by all images in the sequence. The scope and conditions for the practical use of the result include its application to the analysis of images by methods of artificial intelligence
References
- Zhang, J., Li, W., Ogunbona, P., Xu, D. (2019). Recent Advances in Transfer Learning for Cross-dataset Visual Recognition: A Problem-oriented Perspective. ACM Computing Surveys, 52 (1), 1–38. doi: https://doi.org/10.1145/3291124
- Yuan, X., Shi, J., Gu, L. (2021). A review of deep learning methods for semantic segmentation of remote sensing imagery. Expert Systems with Applications, 169, 114417. doi: https://doi.org/10.1016/j.eswa.2020.114417
- Yasin, H. M., Ameen, S. Y. (2021). Review and Evaluation of End-to-End Video Compression with Deep-Learning. 2021 International Conference of Modern Trends in Information and Communication Technology Industry (MTICTI). doi: https://doi.org/10.1109/MTICTI53925.2021.9664790
- Fan, L., Zhang, T., Du, W. (2021). Optical-flow-based framework to boost video object detection performance with object enhancement. Expert Systems with Applications, 170, 114544. doi: https://doi.org/10.1016/j.eswa.2020.114544
- Xue, T., Chen, B., Wu, J., Wei, D., Freeman, W. T. (2019). Video Enhancement with Task-Oriented Flow. International Journal of Computer Vision, 127 (8), 1106–1125. doi: https://doi.org/10.1007/s11263-018-01144-2
- Que, Z., Lu, G., Xu, D. (2021). VoxelContext-Net: An Octree based Framework for Point Cloud Compression. 2021 IEEE/CVF Conference on Computer Vision and Pattern Recognition (CVPR). doi: https://doi.org/10.1109/cvpr46437.2021.00598
- Duan, L., Liu, J., Yang, W., Huang, T., Gao, W. (2020). Video Coding for Machines: A Paradigm of Collaborative Compression and Intelligent Analytics. IEEE Transactions on Image Processing, 29, 8680–8695. doi: https://doi.org/10.1109/tip.2020.3016485
- Lu, G., Cai, C., Zhang, X., Chen, L., Ouyang, W., Xu, D., Gao, Z. (2020). Content Adaptive and Error Propagation Aware Deep Video Compression. Lecture Notes in Computer Science, 456–472. doi: https://doi.org/10.1007/978-3-030-58536-5_27
- Ballé, J., Laparra, V., Simoncelli, E. P. (2017). End-to-end Optimized Image Compression. in Proc. Int. Conf. Learning Representations (ICLR). doi: https://doi.org/10.48550/arXiv.1611.01704
- Liu, D., Li, Y., Lin, J., Li, H., Wu, F. (2020). Deep Learning-Based Video Coding. ACM Computing Surveys, 53 (1), 1–35. doi: https://doi.org/10.1145/3368405
- Subramanian, N., Elharrouss, O., Al-Maadeed, S., Bouridane, A. (2021). Image Steganography: A Review of the Recent Advances. IEEE Access, 9, 23409–23423. doi: https://doi.org/10.1109/access.2021.3053998
- Hu, Y., Yang, W., Ma, Z., Liu, J. (2021). Learning End-to-End Lossy Image Compression: A Benchmark. IEEE Transactions on Pattern Analysis and Machine Intelligence. doi: https://doi.org/10.1109/tpami.2021.3065339
- Hu, Z., Lu, G., Xu, D. (2021). FVC: A New Framework towards Deep Video Compression in Feature Space. 2021 IEEE/CVF Conference on Computer Vision and Pattern Recognition (CVPR). doi: https://doi.org/10.1109/cvpr46437.2021.00155
- Horda, O. V. (2016). Construction of the color atlas for digital image of defects such as a “crack.” ScienceRise, 10 (2 (27)), 55–60. doi: https://doi.org/10.15587/2313-8416.2016.80791
- Horda, E. V., Mykhailenko, V. M. (2016). Struktura tsvetovoho atlasa tsyfrovoho yzobrazhenyia defekta typa "treshchyna ". Scientific discussion, 3, 58–60.
- Nazarenko, I., Dedov, O., Bernyk, I., Rogovskii, I., Bondarenko, A., Zapryvoda, A. et al. (2020). Determining the regions of stability in the motion regimes and parameters of vibratory machines for different technological purposes. Eastern-European Journal of Enterprise Technologies, 6 (7 (108)), 71–79. doi: https://doi.org/10.15587/1729-4061.2020.217747
- Gorda, E., Mihaylenko, V. (2017). Ontology of digital image of defects of type "crack" of objects of construction. Upravlinnia rozvytkom skladnykh system, 30, 142–145. Available at: http://urss.knuba.edu.ua/files/zbirnyk-30/21.pdf
- Serdyuk, A. A., Belyavskiy, L. S. (2018). Analiz veroyatnostno-geometricheskogo metoda opredeleniya mestopolozheniya dvizhuschikhsya obektov. Sovremennaya spetsial'naya tekhnika, 1 (52), 6–13.
- Wolfson, H. J., Rigoutsos, I. (1997). Geometric hashing: an overview. IEEE Computational Science and Engineering, 4 (4), 10–21. doi: https://doi.org/10.1109/99.641604
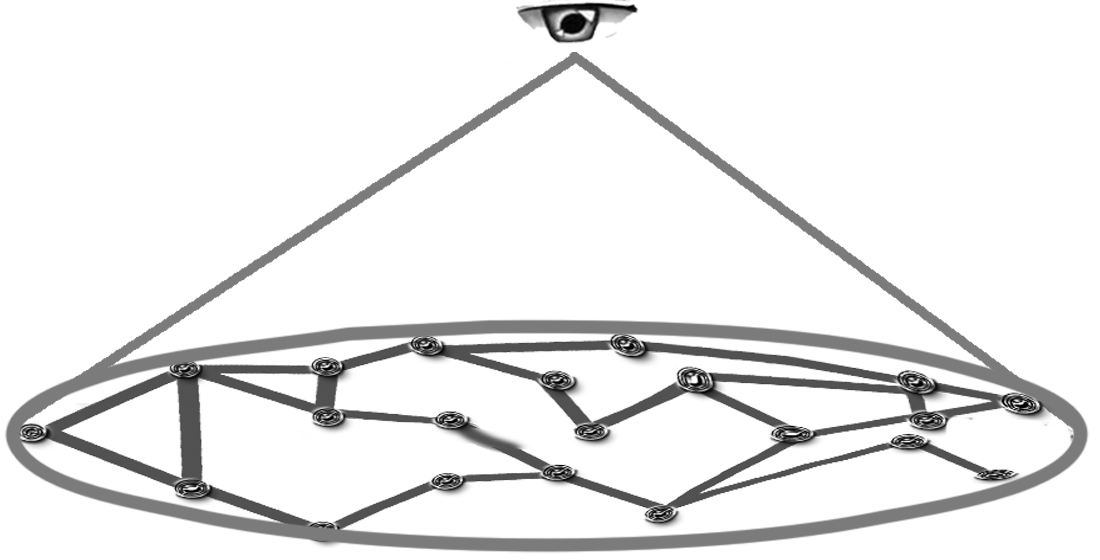
Downloads
Published
How to Cite
Issue
Section
License
Copyright (c) 2023 Elena Gorda, Anatolii Serdiuk, Ivan Nazarenko

This work is licensed under a Creative Commons Attribution 4.0 International License.
The consolidation and conditions for the transfer of copyright (identification of authorship) is carried out in the License Agreement. In particular, the authors reserve the right to the authorship of their manuscript and transfer the first publication of this work to the journal under the terms of the Creative Commons CC BY license. At the same time, they have the right to conclude on their own additional agreements concerning the non-exclusive distribution of the work in the form in which it was published by this journal, but provided that the link to the first publication of the article in this journal is preserved.
A license agreement is a document in which the author warrants that he/she owns all copyright for the work (manuscript, article, etc.).
The authors, signing the License Agreement with TECHNOLOGY CENTER PC, have all rights to the further use of their work, provided that they link to our edition in which the work was published.
According to the terms of the License Agreement, the Publisher TECHNOLOGY CENTER PC does not take away your copyrights and receives permission from the authors to use and dissemination of the publication through the world's scientific resources (own electronic resources, scientometric databases, repositories, libraries, etc.).
In the absence of a signed License Agreement or in the absence of this agreement of identifiers allowing to identify the identity of the author, the editors have no right to work with the manuscript.
It is important to remember that there is another type of agreement between authors and publishers – when copyright is transferred from the authors to the publisher. In this case, the authors lose ownership of their work and may not use it in any way.