The development of a management decision-making method based on the analysis of information from space observation systems
DOI:
https://doi.org/10.15587/1729-4061.2022.269027Keywords:
management decision, space surveillance system, image segmentation, state of the environment, forecastingAbstract
The object of this study is the process of making a management decision based on the analysis of information from space surveillance systems.
Unlike the well-known ones, the method of making a management decision based on the analysis of information from space surveillance systems involves:
– segmentation of an optoelectronic image;
– determination and prediction of a priori probabilities of possible environmental states;
– an application for making a management decision of a combination of Bayes criteria and a minimum of variance.
Experimental studies have been carried out on making a management decision based on the analysis of information from space surveillance systems. To conduct experimental research on making a management decision based on the analysis of information from space surveillance systems, a model problem has been stated. As images from space surveillance systems, images obtained from the WorldView-2 spacecraft (USA) with a difference of four days were considered. The vegetation index was calculated, and the probabilities of degradation dynamics of plant segments were determined. It was established that the maximum value of the estimated functional is achieved when choosing a solution φ1, which is optimal according to the Bayesian criterion and the criterion of minimum variance.
The quality of management decision-making was assessed by the well-known and developed methods. To assess the quality of management decision-making, the concepts of objectivity of the decision-making method and the selectivity of the decision-making method by known and developed method were introduced. It has been established that both methods are objective, and the improved method is more selective (the gain is 2.6 times). This becomes possible through the use of information from space surveillance systems.
References
- Khater, E.-S. G., Ali, S. A., Afify, M. T., Bayomy, M. A., Abbas, R. S. (2022). Using of geographic information systems (GIS) to determine the suitable site for collecting agricultural residues. Scientific Reports, 12 (1). doi: https://doi.org/10.1038/s41598-022-18850-0
- Horbulin, V. P. (2021). The use of space information in the system of geographic information provision of managerial decision-making on Ukraine’s national security and defense issues. Visnyk of the National Academy of Sciences of Ukraine, 9, 3–11. doi: https://doi.org/10.15407/visn2021.09.003
- Harrison, T., Strohmeyer, M. (2022). Commercial Space Remote Sensing and Its Role in National Security. CSIS BRIEFS. Available at: https://csis-website-prod.s3.amazonaws.com/s3fs-public/publication/220202_Harrison_Commercial_Space.pdf?VgV9.43i5ZGs8JDAYDtz0KNbkEnXpH21
- Military Imaging and Surveillance Technology (MIST) (Archived). Available at: https://www.darpa.mil/program/military-imaging-and-surveillance-technology
- Hosseini, S., Baziyad, H., Norouzi, R., Jabbedari Khiabani, S., Gidófalvi, G., Albadvi, A. et al. (2021). Mapping the intellectual structure of GIS-T field (2008–2019): a dynamic co-word analysis. Scientometrics, 126 (4), 2667–2688. doi: https://doi.org/10.1007/s11192-020-03840-8
- Rashidi, K., Noorizadeh, A., Kannan, D., Cullinane, K. (2020). Applying the triple bottom line in sustainable supplier selection: A meta-review of the state-of-the-art. Journal of Cleaner Production, 269, 122001. doi: https://doi.org/10.1016/j.jclepro.2020.122001
- Cherfi, A., Nouira, K., Ferchichi, A. (2018). Very Fast C4.5 Decision Tree Algorithm. Applied Artificial Intelligence, 32 (2), 119–137. doi: https://doi.org/10.1080/08839514.2018.1447479
- Katranzhy, L., Podskrebko, O., Krasko, V. (2018). Modelling the dynamics of the adequacy of bank’s regulatory capital. Baltic Journal of Economic Studies, 4(1), 188–194. doi: https://doi.org/10.30525/2256-0742/2018-4-1-188-194
- Kachayeva, G. I., Mustafayev, A. G. (2018). The use of neural networks for the automatic analysis of electrocardiograms in diagnosis of cardiovascular diseases. Herald of Dagestan State Technical University. Technical Sciences, 45 (2), 114–124. doi: https://doi.org/10.21822/2073-6185-2018-45-2-114-124
- Zhdanov, V. V. (2016). Experimental method to predict avalanches based on neural networks. Ice and Snow, 56 (4), 502–510. doi: https://doi.org/10.15356/2076-6734-2016-4-502-510
- Kanev, A., Nasteka, A., Bessonova, C., Nevmerzhitsky, D., Silaev, A., Efremov, A., Nikiforova, K. (2017). Anomaly detection in wireless sensor network of the “smart home” system. 2017 20th Conference of Open Innovations Association (FRUCT). doi: https://doi.org/10.23919/fruct.2017.8071301
- Sreeshakthy, M., Preethi, J. (2016). Classification of Human Emotion from Deap EEG Signal Using Hybrid Improved Neural Networks with Cuckoo Search. BRAIN: Broad Research in Artificial Intelligence and Neuroscience, 6 (3-4), 60–73. Available at https://doaj.org/article/4c120012c5d44b6abc4e2b389888c7a3
- Chica, J., Zaputt, S., Encalada, J., Salamea, C., Montalvo, M. (2019). Objective assessment of skin repigmentation using a multilayer perceptron. Journal of Medical Signals & Sensors, 9 (2), 88. doi: https://doi.org/10.4103/jmss.jmss_52_18
- Massel, L. V., Gerget, O. M., Massel, A. G., Mamedov, T. G. (2019). The Use of Machine Learning in Situational Management in Relation to the Tasks of the Power Industry. EPJ Web of Conferences, 217, 01010. doi: https://doi.org/10.1051/epjconf/201921701010
- Abaci, K., Yamacli, V. (2019). Hybrid Artificial Neural Network by Using Differential Search Algorithm for Solving Power Flow Problem. Advances in Electrical and Computer Engineering, 19 (4), 57–64. doi: https://doi.org/10.4316/aece.2019.04007
- Mishchuk, O. S., Vitynskyi, P. B. (2018). Neural Network with Combined Approximation of the Surface of the Response. Research Bulletin of the National Technical University of Ukraine “Kyiv Politechnic Institute,” 2, 18–24. doi: https://doi.org/10.20535/1810-0546.2018.2.129022
- Kazemi, M., Faezirad, M. (2018). Efficiency estimation using nonlinear influences of time lags in DEA Using Artificial Neural Networks. Management Journal, 10 (1), 17–34. doi: https://doi.org/10.22059/IMJ.2018.129192.1006898
- Parapuram, G., Mokhtari, M., Ben Hmida, J. (2018). An Artificially Intelligent Technique to Generate Synthetic Geomechanical Well Logs for the Bakken Formation. Energies, 11 (3), 680. doi: https://doi.org/10.3390/en11030680
- Prokoptsev, N. G., Alekseenko, A. E., Kholodov, Y. A. (2018). Traffic flow speed prediction on transportation graph with convolutional neural networks. Computer Research and Modeling, 10 (3), 359–367. doi: https://doi.org/10.20537/2076-7633-2018-10-3-359-367
- Mennis, J., Liu, J. W. (2005). Mining Association Rules in Spatio-Temporal Data: An Analysis of Urban Socioeconomic and Land Cover Change. Transactions in GIS, 9 (1), 5–17. doi: https://doi.org/10.1111/j.1467-9671.2005.00202.x
- Miller, H. J., Han, J. (Eds.) (2009). Geographic Data Mining and Knowledge Discovery. CRC Press, 486. doi: https://doi.org/10.1201/9781420073980
- Manea, E., Di Carlo, D., Depellegrin, D., Agardy, T., Gissi, E. (2019). Multidimensional assessment of supporting ecosystem services for marine spatial planning of the Adriatic Sea. Ecological Indicators, 101, 821–837. doi: https://doi.org/10.1016/j.ecolind.2018.12.017
- Cressie, N. (1993). Statistics for spatial data. John Wiley & Sons, Inc. doi: https://doi.org/10.1002/9781119115151
- History and evolution of big data analytics. Available at https://www.sas.com/en_us/insights/analytics/big-data-analytics.html
- Li, S. Zhi, Hu, Q., Deng, X. Hong, Cai, Z. Quan (2019). Reversible image watermarking based on texture analysis of grey level co-occurrence matrix. International Journal of Computational Science and Engineering, 19 (1), 83. doi: https://doi.org/10.1504/ijcse.2019.099642
- De O. Bastos, L., Liatsis, P., Conci, A. (2008). Automatic texture segmentation based on k-means clustering and efficient calculation of co-occurrence features. 2008 15th International Conference on Systems, Signals and Image Processing. doi: https://doi.org/10.1109/iwssip.2008.4604387
- Hung, C.-C., Song, E., Lan, Y. (2019). Image Texture, Texture Features, and Image Texture Classification and Segmentation. Image Texture Analysis, 3–14. doi: https://doi.org/10.1007/978-3-030-13773-1_1
- Tian, Y., Li, Y., Liu, D., Luo, R. (2016). FCM texture image segmentation method based on the local binary pattern. 2016 12th World Congress on Intelligent Control and Automation (WCICA). doi: https://doi.org/10.1109/wcica.2016.7578571
- Khudov, H., Makoveichuk, O., Butko, I., Gyrenko, I., Stryhun, V., Bilous, O., Shamrai, N. et al. (2022). Devising a method for segmenting camouflaged military equipment on images from space surveillance systems using a genetic algorithm. Eastern-European Journal of Enterprise Technologies, 3 (9 (117)), 6–14. doi: https://doi.org/10.15587/1729-4061.2022.259759
- Jing, Z., Wei, D., Youhui, Z. (2012). An Algorithm for Scanned Document Image Segmentation Based on Voronoi Diagram. 2012 International Conference on Computer Science and Electronics Engineering. doi: https://doi.org/10.1109/iccsee.2012.144
- Shanmugavadivu, P., Sivakumar, V. (2012). Fractal Dimension Based Texture Analysis of Digital Images. Procedia Engineering, 38, 2981–2986. doi: https://doi.org/10.1016/j.proeng.2012.06.348
- Simon, P., V, U. (2020). Deep Learning based Feature Extraction for Texture Classification. Procedia Computer Science, 171, 1680–1687. doi: https://doi.org/10.1016/j.procs.2020.04.180
- Khudov, H., Makoveichuk, O., Khizhnyak, I., Oleksenko, O., Khazhanets, Y., Solomonenko, Y. et al. (2022). Devising a method for segmenting complex structured images acquired from space observation systems based on the particle swarm algorithm. Eastern-European Journal of Enterprise Technologies, 2 (9 (116)), 6–13. doi: https://doi.org/10.15587/1729-4061.2022.255203
- Berezina, S., Solonets, O., Lee, K., Bortsova, M. (2021). An information technique for segmentation of military assets in conditions of uncertainty of initial data. Information Processing Systems, 4 (167), 6–18. doi: https://doi.org/10.30748/soi.2021.167.01
- Ruban, I., Khudov, H., Makoveichuk, O., Chomik, M., Khudov, V., Khizhnyak, I. et al. (2019). Construction of methods for determining the contours of objects on tonal aerospace images based on the ant algorithms. Eastern-European Journal of Enterprise Technologies, 5 (9 (101)), 25–34. doi: https://doi.org/10.15587/1729-4061.2019.177817
- Mittal, H., Pandey, A. C., Saraswat, M., Kumar, S., Pal, R., Modwel, G. (2021). A comprehensive survey of image segmentation: clustering methods, performance parameters, and benchmark datasets. Multimedia Tools and Applications, 81 (24), 35001–35026. doi: https://doi.org/10.1007/s11042-021-10594-9
- Khudov, H., Makoveichuk, O., Khudov, V., Maliuha, V., Andriienko, A., Tertyshnik, Y. et al. (2022). Devising a method for segmenting images acquired from space optical and electronic observation systems based on the Sine-Cosine algorithm. Eastern-European Journal of Enterprise Technologies, 5 (9 (119)), 17–24. doi: https://doi.org/10.15587/1729-4061.2022.265775
- Butko, I. (2021). Model and method of making management decisions based on the analysis of geospatial information. Advanced Information Systems, 5 (2), 42–48. doi: https://doi.org/10.20998/2522-9052.2021.2.07
- Mashtalir, V., Ruban, I., Levashenko, V. (Eds.) (2020). Advances in Spatio-Temporal Segmentation of Visual Data. Studies in Computational Intelligence. doi: https://doi.org/10.1007/978-3-030-35480-0
- Khudov, H., Makoveichuk, O., Misiuk, D., Pievtsov, H., Khizhnyak, I., Solomonenko, Y. et al. (2022). Devising a method for processing the image of a vehicle’s license plate when shooting with a smartphone camera . Eastern-European Journal of Enterprise Technologies, 1 (2 (115), 6–21. doi: https://doi.org/10.15587/1729-4061.2022.252310
- Khudov, H., Makoveychuk, O., Butko, I., Khizhnyak, I. (2021). A Model for Prediction of Geospatial Data in Systems for Processing Geospatial Information. Systems of Arms and Military Equipment, 2 (66), 123–28. doi: https://doi.org/10.30748/soivt.2021.66.16
- Box, G. E. P., Jenkins, G. M., Reinsel, G. C. (1994). Time Series Analysis: Forecasting and Control. Englewood Cliffs, NJ: Prentice Hall. Available at https://www.worldcat.org/title/28888762
- Brockwell, P. J., Davis, R. A. (2009). Time Series: Theory and Methods. Springer, 580. doi: https://doi.org/10.1007/978-1-4419-0320-4
- Khudov, G. V. (2003). Features of optimization of two-alternative decisions by joint search and detection of objects. Problemy Upravleniya i Informatiki (Avtomatika), 5, 51–59. Available at https://www.researchgate.net/publication/291431400_Features_of_optimization_of_two-alternative_decisions_by_joint_search_and_detection_of_objects
- Satellite Imagery. Available at https://www.maxar.com/products/satellite-imagery
- Ronneberger, O., Fischer, P., Brox, T. (2015). U-Net: Convolutional Networks for Biomedical Image Segmentation. Medical Image Computing and Computer-Assisted Intervention – MICCAI 2015, 234–241. doi: https://doi.org/10.1007/978-3-319-24574-4_28
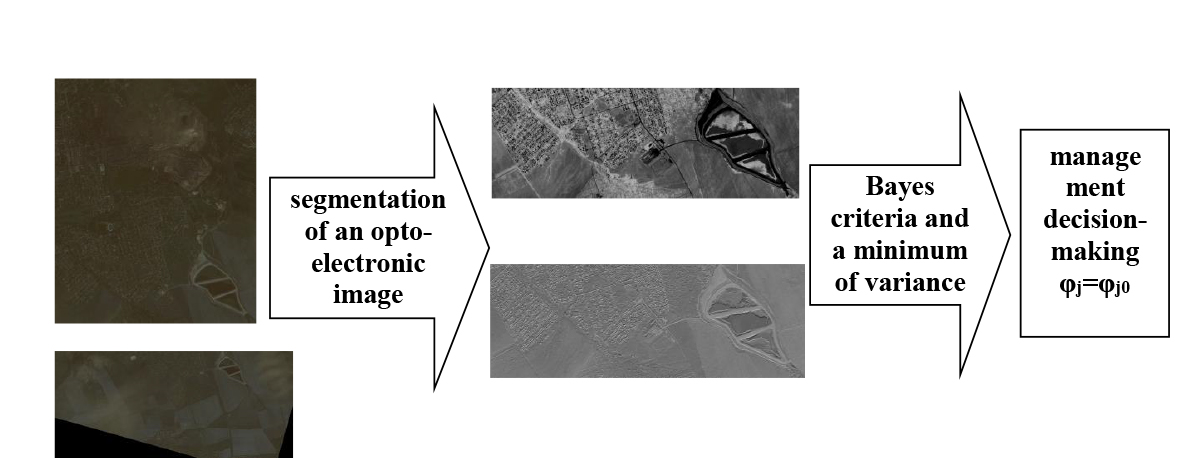
Downloads
Published
How to Cite
Issue
Section
License
Copyright (c) 2022 Hennadii Khudov, Oleksandr Makoveichuk, Ihor Butko, Mykola Butko, Veronika Khudolei, Stanislav Kukhtyk

This work is licensed under a Creative Commons Attribution 4.0 International License.
The consolidation and conditions for the transfer of copyright (identification of authorship) is carried out in the License Agreement. In particular, the authors reserve the right to the authorship of their manuscript and transfer the first publication of this work to the journal under the terms of the Creative Commons CC BY license. At the same time, they have the right to conclude on their own additional agreements concerning the non-exclusive distribution of the work in the form in which it was published by this journal, but provided that the link to the first publication of the article in this journal is preserved.
A license agreement is a document in which the author warrants that he/she owns all copyright for the work (manuscript, article, etc.).
The authors, signing the License Agreement with TECHNOLOGY CENTER PC, have all rights to the further use of their work, provided that they link to our edition in which the work was published.
According to the terms of the License Agreement, the Publisher TECHNOLOGY CENTER PC does not take away your copyrights and receives permission from the authors to use and dissemination of the publication through the world's scientific resources (own electronic resources, scientometric databases, repositories, libraries, etc.).
In the absence of a signed License Agreement or in the absence of this agreement of identifiers allowing to identify the identity of the author, the editors have no right to work with the manuscript.
It is important to remember that there is another type of agreement between authors and publishers – when copyright is transferred from the authors to the publisher. In this case, the authors lose ownership of their work and may not use it in any way.