Розробка методу прийняття управлінського рішення на основі аналізу інформації з космічних систем спостереження
DOI:
https://doi.org/10.15587/1729-4061.2022.269027Ключові слова:
управлінське рішення, космічна система спостереження, сегментування зображення, стан середовища, прогнозуванняАнотація
Об’єктом дослідження є процес прийняття управлінського рішення на основі аналізу інформації з космічних систем спостереження.
На відміну від відомих, метод прийняття управлінського рішення на основі аналізу інформації з космічних систем спостереження передбачає:
– сегментування оптико-електронного зображення;
– визначення та прогнозування апріорних ймовірностей можливих станів середовища;
– застосування для прийняття управлінського рішення комбінації критеріїв Байєса та мінімуму дисперсії.
Проведені експериментальні дослідження щодо прийняття управлінського рішення на основі аналізу інформації з космічних систем спостереження. Для проведення експериментальних досліджень щодо прийняття управлінського рішення на основі аналізу інформації з космічних систем спостереження сформульовано модельна задача. У якості зображень з космічних систем спостереження розглянуто зображення, що отримані з космічного апарату WorldView-2 (США) з різницею у чотири доби. Розраховано вегетаційний індекс та визначені ймовірності деградаційної динаміки рослинних сегментів. Встановлено, що максимальне значення оціночного функціоналу досягається при виборі рішення φ1, яке є оптимальним за байєсовським критерієм та критерієм мінімуму дисперсії.
Проведено оцінювання якості прийняття управлінського рішення відомими та розробленим методом. Для оцінювання якості прийняття управлінського рішення відомими та розробленим методом введені поняття об’єктивності методу прийняття рішення та селективності методу прийняття рішення. Встановлено, що обидва метода є об’єктивними та удосконалений метод є більш селективним (виграш складає 2,6 разів). Це стає можливим завдяки використанню інформації космічних систем спостереження
Посилання
- Khater, E.-S. G., Ali, S. A., Afify, M. T., Bayomy, M. A., Abbas, R. S. (2022). Using of geographic information systems (GIS) to determine the suitable site for collecting agricultural residues. Scientific Reports, 12 (1). doi: https://doi.org/10.1038/s41598-022-18850-0
- Horbulin, V. P. (2021). The use of space information in the system of geographic information provision of managerial decision-making on Ukraine’s national security and defense issues. Visnyk of the National Academy of Sciences of Ukraine, 9, 3–11. doi: https://doi.org/10.15407/visn2021.09.003
- Harrison, T., Strohmeyer, M. (2022). Commercial Space Remote Sensing and Its Role in National Security. CSIS BRIEFS. Available at: https://csis-website-prod.s3.amazonaws.com/s3fs-public/publication/220202_Harrison_Commercial_Space.pdf?VgV9.43i5ZGs8JDAYDtz0KNbkEnXpH21
- Military Imaging and Surveillance Technology (MIST) (Archived). Available at: https://www.darpa.mil/program/military-imaging-and-surveillance-technology
- Hosseini, S., Baziyad, H., Norouzi, R., Jabbedari Khiabani, S., Gidófalvi, G., Albadvi, A. et al. (2021). Mapping the intellectual structure of GIS-T field (2008–2019): a dynamic co-word analysis. Scientometrics, 126 (4), 2667–2688. doi: https://doi.org/10.1007/s11192-020-03840-8
- Rashidi, K., Noorizadeh, A., Kannan, D., Cullinane, K. (2020). Applying the triple bottom line in sustainable supplier selection: A meta-review of the state-of-the-art. Journal of Cleaner Production, 269, 122001. doi: https://doi.org/10.1016/j.jclepro.2020.122001
- Cherfi, A., Nouira, K., Ferchichi, A. (2018). Very Fast C4.5 Decision Tree Algorithm. Applied Artificial Intelligence, 32 (2), 119–137. doi: https://doi.org/10.1080/08839514.2018.1447479
- Katranzhy, L., Podskrebko, O., Krasko, V. (2018). Modelling the dynamics of the adequacy of bank’s regulatory capital. Baltic Journal of Economic Studies, 4(1), 188–194. doi: https://doi.org/10.30525/2256-0742/2018-4-1-188-194
- Kachayeva, G. I., Mustafayev, A. G. (2018). The use of neural networks for the automatic analysis of electrocardiograms in diagnosis of cardiovascular diseases. Herald of Dagestan State Technical University. Technical Sciences, 45 (2), 114–124. doi: https://doi.org/10.21822/2073-6185-2018-45-2-114-124
- Zhdanov, V. V. (2016). Experimental method to predict avalanches based on neural networks. Ice and Snow, 56 (4), 502–510. doi: https://doi.org/10.15356/2076-6734-2016-4-502-510
- Kanev, A., Nasteka, A., Bessonova, C., Nevmerzhitsky, D., Silaev, A., Efremov, A., Nikiforova, K. (2017). Anomaly detection in wireless sensor network of the “smart home” system. 2017 20th Conference of Open Innovations Association (FRUCT). doi: https://doi.org/10.23919/fruct.2017.8071301
- Sreeshakthy, M., Preethi, J. (2016). Classification of Human Emotion from Deap EEG Signal Using Hybrid Improved Neural Networks with Cuckoo Search. BRAIN: Broad Research in Artificial Intelligence and Neuroscience, 6 (3-4), 60–73. Available at https://doaj.org/article/4c120012c5d44b6abc4e2b389888c7a3
- Chica, J., Zaputt, S., Encalada, J., Salamea, C., Montalvo, M. (2019). Objective assessment of skin repigmentation using a multilayer perceptron. Journal of Medical Signals & Sensors, 9 (2), 88. doi: https://doi.org/10.4103/jmss.jmss_52_18
- Massel, L. V., Gerget, O. M., Massel, A. G., Mamedov, T. G. (2019). The Use of Machine Learning in Situational Management in Relation to the Tasks of the Power Industry. EPJ Web of Conferences, 217, 01010. doi: https://doi.org/10.1051/epjconf/201921701010
- Abaci, K., Yamacli, V. (2019). Hybrid Artificial Neural Network by Using Differential Search Algorithm for Solving Power Flow Problem. Advances in Electrical and Computer Engineering, 19 (4), 57–64. doi: https://doi.org/10.4316/aece.2019.04007
- Mishchuk, O. S., Vitynskyi, P. B. (2018). Neural Network with Combined Approximation of the Surface of the Response. Research Bulletin of the National Technical University of Ukraine “Kyiv Politechnic Institute,” 2, 18–24. doi: https://doi.org/10.20535/1810-0546.2018.2.129022
- Kazemi, M., Faezirad, M. (2018). Efficiency estimation using nonlinear influences of time lags in DEA Using Artificial Neural Networks. Management Journal, 10 (1), 17–34. doi: https://doi.org/10.22059/IMJ.2018.129192.1006898
- Parapuram, G., Mokhtari, M., Ben Hmida, J. (2018). An Artificially Intelligent Technique to Generate Synthetic Geomechanical Well Logs for the Bakken Formation. Energies, 11 (3), 680. doi: https://doi.org/10.3390/en11030680
- Prokoptsev, N. G., Alekseenko, A. E., Kholodov, Y. A. (2018). Traffic flow speed prediction on transportation graph with convolutional neural networks. Computer Research and Modeling, 10 (3), 359–367. doi: https://doi.org/10.20537/2076-7633-2018-10-3-359-367
- Mennis, J., Liu, J. W. (2005). Mining Association Rules in Spatio-Temporal Data: An Analysis of Urban Socioeconomic and Land Cover Change. Transactions in GIS, 9 (1), 5–17. doi: https://doi.org/10.1111/j.1467-9671.2005.00202.x
- Miller, H. J., Han, J. (Eds.) (2009). Geographic Data Mining and Knowledge Discovery. CRC Press, 486. doi: https://doi.org/10.1201/9781420073980
- Manea, E., Di Carlo, D., Depellegrin, D., Agardy, T., Gissi, E. (2019). Multidimensional assessment of supporting ecosystem services for marine spatial planning of the Adriatic Sea. Ecological Indicators, 101, 821–837. doi: https://doi.org/10.1016/j.ecolind.2018.12.017
- Cressie, N. (1993). Statistics for spatial data. John Wiley & Sons, Inc. doi: https://doi.org/10.1002/9781119115151
- History and evolution of big data analytics. Available at https://www.sas.com/en_us/insights/analytics/big-data-analytics.html
- Li, S. Zhi, Hu, Q., Deng, X. Hong, Cai, Z. Quan (2019). Reversible image watermarking based on texture analysis of grey level co-occurrence matrix. International Journal of Computational Science and Engineering, 19 (1), 83. doi: https://doi.org/10.1504/ijcse.2019.099642
- De O. Bastos, L., Liatsis, P., Conci, A. (2008). Automatic texture segmentation based on k-means clustering and efficient calculation of co-occurrence features. 2008 15th International Conference on Systems, Signals and Image Processing. doi: https://doi.org/10.1109/iwssip.2008.4604387
- Hung, C.-C., Song, E., Lan, Y. (2019). Image Texture, Texture Features, and Image Texture Classification and Segmentation. Image Texture Analysis, 3–14. doi: https://doi.org/10.1007/978-3-030-13773-1_1
- Tian, Y., Li, Y., Liu, D., Luo, R. (2016). FCM texture image segmentation method based on the local binary pattern. 2016 12th World Congress on Intelligent Control and Automation (WCICA). doi: https://doi.org/10.1109/wcica.2016.7578571
- Khudov, H., Makoveichuk, O., Butko, I., Gyrenko, I., Stryhun, V., Bilous, O., Shamrai, N. et al. (2022). Devising a method for segmenting camouflaged military equipment on images from space surveillance systems using a genetic algorithm. Eastern-European Journal of Enterprise Technologies, 3 (9 (117)), 6–14. doi: https://doi.org/10.15587/1729-4061.2022.259759
- Jing, Z., Wei, D., Youhui, Z. (2012). An Algorithm for Scanned Document Image Segmentation Based on Voronoi Diagram. 2012 International Conference on Computer Science and Electronics Engineering. doi: https://doi.org/10.1109/iccsee.2012.144
- Shanmugavadivu, P., Sivakumar, V. (2012). Fractal Dimension Based Texture Analysis of Digital Images. Procedia Engineering, 38, 2981–2986. doi: https://doi.org/10.1016/j.proeng.2012.06.348
- Simon, P., V, U. (2020). Deep Learning based Feature Extraction for Texture Classification. Procedia Computer Science, 171, 1680–1687. doi: https://doi.org/10.1016/j.procs.2020.04.180
- Khudov, H., Makoveichuk, O., Khizhnyak, I., Oleksenko, O., Khazhanets, Y., Solomonenko, Y. et al. (2022). Devising a method for segmenting complex structured images acquired from space observation systems based on the particle swarm algorithm. Eastern-European Journal of Enterprise Technologies, 2 (9 (116)), 6–13. doi: https://doi.org/10.15587/1729-4061.2022.255203
- Berezina, S., Solonets, O., Lee, K., Bortsova, M. (2021). An information technique for segmentation of military assets in conditions of uncertainty of initial data. Information Processing Systems, 4 (167), 6–18. doi: https://doi.org/10.30748/soi.2021.167.01
- Ruban, I., Khudov, H., Makoveichuk, O., Chomik, M., Khudov, V., Khizhnyak, I. et al. (2019). Construction of methods for determining the contours of objects on tonal aerospace images based on the ant algorithms. Eastern-European Journal of Enterprise Technologies, 5 (9 (101)), 25–34. doi: https://doi.org/10.15587/1729-4061.2019.177817
- Mittal, H., Pandey, A. C., Saraswat, M., Kumar, S., Pal, R., Modwel, G. (2021). A comprehensive survey of image segmentation: clustering methods, performance parameters, and benchmark datasets. Multimedia Tools and Applications, 81 (24), 35001–35026. doi: https://doi.org/10.1007/s11042-021-10594-9
- Khudov, H., Makoveichuk, O., Khudov, V., Maliuha, V., Andriienko, A., Tertyshnik, Y. et al. (2022). Devising a method for segmenting images acquired from space optical and electronic observation systems based on the Sine-Cosine algorithm. Eastern-European Journal of Enterprise Technologies, 5 (9 (119)), 17–24. doi: https://doi.org/10.15587/1729-4061.2022.265775
- Butko, I. (2021). Model and method of making management decisions based on the analysis of geospatial information. Advanced Information Systems, 5 (2), 42–48. doi: https://doi.org/10.20998/2522-9052.2021.2.07
- Mashtalir, V., Ruban, I., Levashenko, V. (Eds.) (2020). Advances in Spatio-Temporal Segmentation of Visual Data. Studies in Computational Intelligence. doi: https://doi.org/10.1007/978-3-030-35480-0
- Khudov, H., Makoveichuk, O., Misiuk, D., Pievtsov, H., Khizhnyak, I., Solomonenko, Y. et al. (2022). Devising a method for processing the image of a vehicle’s license plate when shooting with a smartphone camera . Eastern-European Journal of Enterprise Technologies, 1 (2 (115), 6–21. doi: https://doi.org/10.15587/1729-4061.2022.252310
- Khudov, H., Makoveychuk, O., Butko, I., Khizhnyak, I. (2021). A Model for Prediction of Geospatial Data in Systems for Processing Geospatial Information. Systems of Arms and Military Equipment, 2 (66), 123–28. doi: https://doi.org/10.30748/soivt.2021.66.16
- Box, G. E. P., Jenkins, G. M., Reinsel, G. C. (1994). Time Series Analysis: Forecasting and Control. Englewood Cliffs, NJ: Prentice Hall. Available at https://www.worldcat.org/title/28888762
- Brockwell, P. J., Davis, R. A. (2009). Time Series: Theory and Methods. Springer, 580. doi: https://doi.org/10.1007/978-1-4419-0320-4
- Khudov, G. V. (2003). Features of optimization of two-alternative decisions by joint search and detection of objects. Problemy Upravleniya i Informatiki (Avtomatika), 5, 51–59. Available at https://www.researchgate.net/publication/291431400_Features_of_optimization_of_two-alternative_decisions_by_joint_search_and_detection_of_objects
- Satellite Imagery. Available at https://www.maxar.com/products/satellite-imagery
- Ronneberger, O., Fischer, P., Brox, T. (2015). U-Net: Convolutional Networks for Biomedical Image Segmentation. Medical Image Computing and Computer-Assisted Intervention – MICCAI 2015, 234–241. doi: https://doi.org/10.1007/978-3-319-24574-4_28
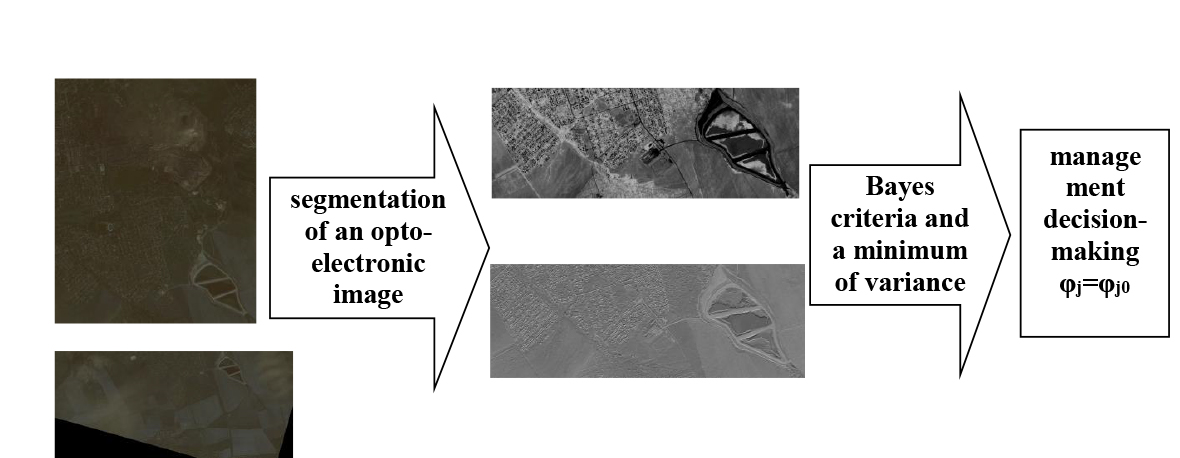
##submission.downloads##
Опубліковано
Як цитувати
Номер
Розділ
Ліцензія
Авторське право (c) 2022 Hennadii Khudov, Oleksandr Makoveichuk, Ihor Butko, Mykola Butko, Veronika Khudolei, Stanislav Kukhtyk

Ця робота ліцензується відповідно до Creative Commons Attribution 4.0 International License.
Закріплення та умови передачі авторських прав (ідентифікація авторства) здійснюється у Ліцензійному договорі. Зокрема, автори залишають за собою право на авторство свого рукопису та передають журналу право першої публікації цієї роботи на умовах ліцензії Creative Commons CC BY. При цьому вони мають право укладати самостійно додаткові угоди, що стосуються неексклюзивного поширення роботи у тому вигляді, в якому вона була опублікована цим журналом, але за умови збереження посилання на першу публікацію статті в цьому журналі.
Ліцензійний договір – це документ, в якому автор гарантує, що володіє усіма авторськими правами на твір (рукопис, статтю, тощо).
Автори, підписуючи Ліцензійний договір з ПП «ТЕХНОЛОГІЧНИЙ ЦЕНТР», мають усі права на подальше використання свого твору за умови посилання на наше видання, в якому твір опублікований. Відповідно до умов Ліцензійного договору, Видавець ПП «ТЕХНОЛОГІЧНИЙ ЦЕНТР» не забирає ваші авторські права та отримує від авторів дозвіл на використання та розповсюдження публікації через світові наукові ресурси (власні електронні ресурси, наукометричні бази даних, репозитарії, бібліотеки тощо).
За відсутності підписаного Ліцензійного договору або за відсутністю вказаних в цьому договорі ідентифікаторів, що дають змогу ідентифікувати особу автора, редакція не має права працювати з рукописом.
Важливо пам’ятати, що існує і інший тип угоди між авторами та видавцями – коли авторські права передаються від авторів до видавця. В такому разі автори втрачають права власності на свій твір та не можуть його використовувати в будь-який спосіб.