Developing a convolutional neural network for classifying tumor images using Inception v3
DOI:
https://doi.org/10.15587/1729-4061.2023.281227Keywords:
convolutional neural network, deep learning, classification, Inception architecture, brain tumorAbstract
Deep learning algorithms rely on digital pathology to classify tissue tumors, where the whole tissue slides are digitized and imaged. The produced multi-resolution whole slide images (MWSIs) are with high resolution that may range from about 100,000 to 200,000 pixels. MWSIs are often stored in a multi-resolution configuration to simplify the processing of images, navigation, and efficient exposition. This work develops a network for classifying MWSIs that require high memory employing a deep neural Inception-v3 architecture. This work employs the MWSIs from Camelyon16, which is around 451 GB in size of Challenge dataset from two independent sources including 400 MWSIs as a total of lymph nodes. The training dataset contains 111 MWSIs of tumor tissue and lymph nodes and 159 WSIs of normal lymph nodes. The developed model uses sample-based processing to train extensive MWSIs employing the MATLAB platform. The model introduces transfer learning techniques with an Inception-v3-based architecture to categorize separate samples as a tumor or normal. Therefore, the main aim here is to achieve two-classes binary segmentation containing normal and tumor. This includes creating a new fully connected layer for the Inception-v3 architecture with two classes and compensating new layers instead of the original final fully-connected layers. The results obtained demonstrated that the heatmap visualization can recognize the boundary coordinates of ground truth as sketchy Region Of Interest (ROI), where the green boundary represents the normal regions and the tumor area with red boundaries. The proposed Inception v3 Convolutional Neural Network (CNN) architecture can achieve more than 92.8 % accuracy for such MWSIs dataset to categorize brain tumors into normal and tumor tissue
References
- Abdelaziz Ismael, S. A., Mohammed, A., Hefny, H. (2020). An enhanced deep learning approach for brain cancer MRI images classification using residual networks. Artificial Intelligence in Medicine, 102, 101779. doi: https://doi.org/10.1016/j.artmed.2019.101779
- Sfayyih, A. H., Sabry, A. H., Jameel, S. M., Sulaiman, N., Raafat, S. M., Humaidi, A. J., Kubaiaisi, Y. M. A. (2023). Acoustic-Based Deep Learning Architectures for Lung Disease Diagnosis: A Comprehensive Overview. Diagnostics, 13 (10), 1748. doi: https://doi.org/10.3390/diagnostics13101748
- Abd-Ellah, M. K., Awad, A. I., Khalaf, A. A. M., Hamed, H. F. A. (2018). Two-phase multi-model automatic brain tumour diagnosis system from magnetic resonance images using convolutional neural networks. EURASIP Journal on Image and Video Processing, 2018 (1). doi: https://doi.org/10.1186/s13640-018-0332-4
- Abd El Kader, I., Xu, G., Shuai, Z., Saminu, S., Javaid, I., Salim Ahmad, I. (2021). Differential Deep Convolutional Neural Network Model for Brain Tumor Classification. Brain Sciences, 11 (3), 352. doi: https://doi.org/10.3390/brainsci11030352
- Houssein, E. H., Emam, M. M., Ali, A. A., Suganthan, P. N. (2021). Deep and machine learning techniques for medical imaging-based breast cancer: A comprehensive review. Expert Systems with Applications, 167, 114161. doi: https://doi.org/10.1016/j.eswa.2020.114161
- Zhen, S., Cheng, M., Tao, Y., Wang, Y., Juengpanich, S., Jiang, Z. et al. (2020). Deep Learning for Accurate Diagnosis of Liver Tumor Based on Magnetic Resonance Imaging and Clinical Data. Frontiers in Oncology, 10. doi: https://doi.org/10.3389/fonc.2020.00680
- Alqudah, A. M. (2019). Brain Tumor Classification Using Deep Learning Technique - A Comparison between Cropped, Uncropped, and Segmented Lesion Images with Different Sizes. International Journal of Advanced Trends in Computer Science and Engineering, 8 (6), 3684–3691. doi: https://doi.org/10.30534/ijatcse/2019/155862019
- Wang, H., Zhou, Z., Li, Y., Chen, Z., Lu, P., Wang, W. et al. (2017). Comparison of machine learning methods for classifying mediastinal lymph node metastasis of non-small cell lung cancer from 18F-FDG PET/CT images. EJNMMI Research, 7 (1). doi: https://doi.org/10.1186/s13550-017-0260-9
- Hoseini, F., Shahbahrami, A., Bayat, P. (2018). An Efficient Implementation of Deep Convolutional Neural Networks for MRI Segmentation. Journal of Digital Imaging, 31 (5), 738–747. doi: https://doi.org/10.1007/s10278-018-0062-2
- Wei, J. W., Tafe, L. J., Linnik, Y. A., Vaickus, L. J., Tomita, N., Hassanpour, S. (2019). Pathologist-level classification of histologic patterns on resected lung adenocarcinoma slides with deep neural networks. Scientific Reports, 9 (1). doi: https://doi.org/10.1038/s41598-019-40041-7
- Mehrotra, R., Ansari, M. A., Agrawal, R., Anand, R. S. (2020). A Transfer Learning approach for AI-based classification of brain tumors. Machine Learning with Applications, 2, 100003. doi: https://doi.org/10.1016/j.mlwa.2020.100003
- Advanced Guide to Inception v3. Cloud TPU. Google Cloud. Available at: https://cloud.google.com/tpu/docs/inception-v3-advanced
- Litjens, G., Bandi, P., Ehteshami Bejnordi, B., Geessink, O., Balkenhol, M., Bult, P. et al. (2018). 1399 H&E-stained sentinel lymph node sections of breast cancer patients: the CAMELYON dataset. GigaScience, 7 (6). doi: https://doi.org/10.1093/gigascience/giy065
- Szegedy, C., Vanhoucke, V., Ioffe, S., Shlens, J., Wojna, Z. (2016). Rethinking the Inception Architecture for Computer Vision. 2016 IEEE Conference on Computer Vision and Pattern Recognition (CVPR). doi: https://doi.org/10.1109/cvpr.2016.308
- ImageNet. Available at:https://www.image-net.org/
- Raharjo, B., Farida, N., Subekti, P., Herlina, S., Doddy, H., Rahim, R. (2021). Optimization forecasting using back-propagation algorithm. Journal of Applied Engineering Science, 19 (4), 1083–1089. doi: https://doi.org/10.5937/jaes0-30175
- Negassi, M., Suarez-Ibarrola, R., Hein, S., Miernik, A., Reiterer, A. (2020). Application of artificial neural networks for automated analysis of cystoscopic images: a review of the current status and future prospects. World Journal of Urology, 38 (10), 2349–2358. doi: https://doi.org/10.1007/s00345-019-03059-0
- Zhuang, Z., Ding, W., Zhuang, S., Joseph Raj, A. N., Wang, J., Zhou, W., Wei, C. (2021). Tumor classification in automated breast ultrasound (ABUS) based on a modified extracting feature network. Computerized Medical Imaging and Graphics, 90, 101925. doi: https://doi.org/10.1016/j.compmedimag.2021.101925
- Alanazi, M. F., Ali, M. U., Hussain, S. J., Zafar, A., Mohatram, M., Irfan, M., AlRuwaili, R. et al. (2022). Brain Tumor/Mass Classification Framework Using Magnetic-Resonance-Imaging-Based Isolated and Developed Transfer Deep-Learning Model. Sensors, 22 (1), 372. doi: https://doi.org/10.3390/s22010372
- Mahmood, T., Li, J., Pei, Y., Akhtar, F., Rehman, M. U., Wasti, S. H. (2022). Breast lesions classifications of mammographic images using a deep convolutional neural network-based approach. PLOS ONE, 17 (1), e0263126. doi: https://doi.org/10.1371/journal.pone.0263126
- Asif, S., Yi, W., Ain, Q. U., Hou, J., Yi, T., Si, J. (2022). Improving Effectiveness of Different Deep Transfer Learning-Based Models for Detecting Brain Tumors From MR Images. IEEE Access, 10, 34716–34730. doi: https://doi.org/10.1109/access.2022.3153306
- Jwaid, W. M., Al-Husseini, Z. S. M., Sabry, A. H. (2021). Development of brain tumor segmentation of magnetic resonance imaging (MRI) using U-Net deep learning. Eastern-European Journal of Enterprise Technologies, 4 (9 (112)), 23–31. doi: https://doi.org/10.15587/1729-4061.2021.238957
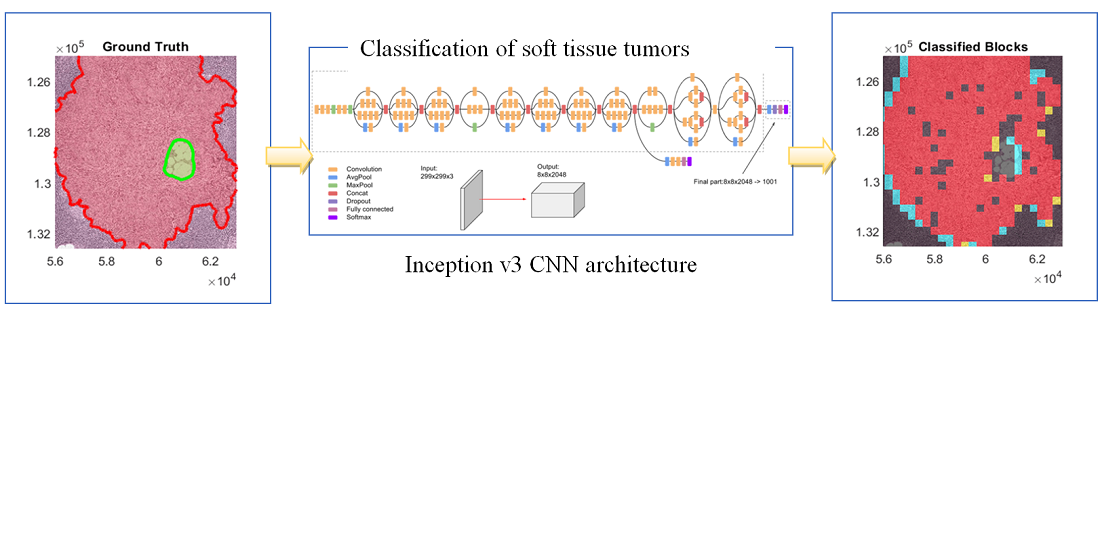
Downloads
Published
How to Cite
Issue
Section
License
Copyright (c) 2023 Ali A. Mahmood, Sadeer Sadeq, Yaser Issam Aljanabi, Ahmad H. Sabry

This work is licensed under a Creative Commons Attribution 4.0 International License.
The consolidation and conditions for the transfer of copyright (identification of authorship) is carried out in the License Agreement. In particular, the authors reserve the right to the authorship of their manuscript and transfer the first publication of this work to the journal under the terms of the Creative Commons CC BY license. At the same time, they have the right to conclude on their own additional agreements concerning the non-exclusive distribution of the work in the form in which it was published by this journal, but provided that the link to the first publication of the article in this journal is preserved.
A license agreement is a document in which the author warrants that he/she owns all copyright for the work (manuscript, article, etc.).
The authors, signing the License Agreement with TECHNOLOGY CENTER PC, have all rights to the further use of their work, provided that they link to our edition in which the work was published.
According to the terms of the License Agreement, the Publisher TECHNOLOGY CENTER PC does not take away your copyrights and receives permission from the authors to use and dissemination of the publication through the world's scientific resources (own electronic resources, scientometric databases, repositories, libraries, etc.).
In the absence of a signed License Agreement or in the absence of this agreement of identifiers allowing to identify the identity of the author, the editors have no right to work with the manuscript.
It is important to remember that there is another type of agreement between authors and publishers – when copyright is transferred from the authors to the publisher. In this case, the authors lose ownership of their work and may not use it in any way.