Development of decision-making technique based on sentiment analysis of crowdsourcing data in medical social media resources
DOI:
https://doi.org/10.15587/1729-4061.2023.289989Keywords:
medical social media, decision-making, patient opinions, clinic activity, sentiment analysisAbstract
The object of the study is the decision-making modeling in the context of medical social media to increase the clinics’ effectiveness. The problem is to classify the patient reviews collected in the patient-clinic segment of the medical social media and to identify the situation related to the clinics’ activity by revealing the criteria characterizing the clinics’ activity out of the opinions.
The proposed technique refers to lexicon-based sentiment analysis of opinions, the classification based on Valence Aware Dictionary and Sentiment Reasoner (VADER), the verification of the results accuracy with Multinomial Naive Bayes and Support Vector Machine, the manual sentiment analysis of opinions to detect criteria and the classification of opinions according to each criterion.
Using this technique, out of 442587 patient reviews obtained from database cms_hospital_satisfaction_2020 of the Kaggle company generated on the basis of crowdsourcing of patient reviews on medical social media, 218914 patient reviews are classified as positive, 190360 – as neutral, and 33313 – as negative. The results accuracy is verified, and the clinics are rated by the «positive» opinions. 6 new criteria characterizing the clinics’ activity are discovered, and the identification of the situation related to the clinics’ activity based on the comparison of «positive» and «negative» opinions according to each criterion is presented.
The possibility of using the results obtained from the identification to increase the clinics’ efficiency in making decisions is shown.
The results obtained in this study can be used to improve the clinics’ performance according to public opinion. This opportunity involves the crowdsourcing of opinions about the clinic in the medical social media environment and the collection of opinions in a structured way.
References
- Emin, M. (2018). A Qualitative Study on the Reasons for Social Media Addiction. European Journal of Educational Research, 7 (4), 861–865. doi: https://doi.org/10.12973/eu-jer.7.4.861
- Yusifov, F., Alguliyev, R., Aliguliyev, R. (2018). Role of Social Networks in E-government: Risks and Security Threats. Online Journal of Communication and Media Technologies, 8 (4). doi: https://doi.org/10.12973/ojcmt/3957
- Salikhova, N. R., Grigoryeva, O. V., Semenova-Poliakh, G. G., Salikhova, A. B., Smirnikova, O. V., Sopun, S. M. (2023). Communication tools and social media usage: Assessing self-perceived communication competence. Online Journal of Communication and Media Technologies, 13 (4), e202343. doi: https://doi.org/10.30935/ojcmt/13453
- Zaw, H. T. (2018). The Impact of Social Media on Cultural Adaptation Process: Study on Chinese Government Scholarship Students. Advances in Journalism and Communication, 06 (03), 75–89. doi: https://doi.org/10.4236/ajc.2018.63007
- Swan, M. (2009). Emerging Patient-Driven Health Care Models: An Examination of Health Social Networks, Consumer Personalized Medicine and Quantified Self-Tracking. International Journal of Environmental Research and Public Health, 6 (2), 492–525. doi: https://doi.org/10.3390/ijerph6020492
- Cesare, N., Grant, C., Hawkins, J. B., Brownstein, J. S., Nsoesie, E. O. (2017). Demographics in Social Media Data for Public Health Research: Does it matter? Bloomberg Data for Good Exchange Conference. doi: https://doi.org/10.48550/arXiv.1710.11048
- Renuka, D. K., Jeetha, B. R. (2017). Dynamic and reliable intelligent data mining technique on social media drug related posts. 2017 IEEE International Conference on Power, Control, Signals and Instrumentation Engineering (ICPCSI). doi: https://doi.org/10.1109/icpcsi.2017.8392022
- Yadav, S., Ekbal, A., Saha, S., Bhattacharyya, P. (2018). Medical Sentiment Analysis using Social Media: Towards building a Patient Assisted System. Proceedings of the Eleventh International Conference on Language Resources and Evaluation (LREC 2018). Miyazaki, 2790–2797. Available at: https://aclanthology.org/L18-1442.pdf
- Zhang, M., Zhang, M., Ge, C., Liu, Q., Wang, J., Wei, J., Zhu, K. Q. (2019). Automatic discovery of adverse reactions through Chinese social media. Data Mining and Knowledge Discovery, 33 (4), 848–870. doi: https://doi.org/10.1007/s10618-018-00610-2
- Wang, C., Han, L., Stein, G., Day, S., Bien-Gund, C., Mathews, A. et al. (2020). Crowdsourcing in health and medical research: a systematic review. Infectious Diseases of Poverty, 9 (1). doi: https://doi.org/10.1186/s40249-020-0622-9
- Tucker, J. D., Day, S., Tang, W., Bayus, B. (2019). Crowdsourcing in medical research: concepts and applications. PeerJ, 7, e6762. doi: https://doi.org/10.7717/peerj.6762
- Mammadova, M., Jabrayilova, Z. (2019). Electronic medicine: formation and scientific-theoretical problems. Baku: "Information Technologies" publishing house, 319. Available at: https://ict.az/uploads/files/E-medicine-monograph-IIT-ANAS.pdf
- Medina-Aguerrebere, P., Medina, E., Gonzalez-Pacanowski, T. (2023). Branding cancer research institutions through social media platforms. Online Journal of Communication and Media Technologies, 13 (2), e202313. doi: https://doi.org/10.30935/ojcmt/12955
- Swan, M. (2012). Crowdsourced Health Research Studies: An Important Emerging Complement to Clinical Trials in the Public Health Research Ecosystem. Journal of Medical Internet Research, 14 (2), e46. doi: https://doi.org/10.2196/jmir.1988
- Mammadova, M. H., Jabrayilova, Z. G., Isayeva, A. M. (2019). Analysis of physician-patient relations segment of social media: opportunities and challenges. Problems of information society, 2, 41–50. doi: https://doi.org/10.25045/jpis.v10.i2.04
- Mammadovа, M., Jabrayilova, Z., Isayeva, A. (2020). Conceptual Approach to the Use of Information Acquired in Social Media for Medial Decisions. Online Journal of Communication and Media Technologies, 10 (2). doi: https://doi.org/10.29333/ojcmt/7877
- Mammadova, M., Jabrayilova, Z., Shikhaliyeva, N. (2022). Lexicon-based sentiment analysis of medical data. Technology Transfer: Fundamental Principles and Innovative Technical Solutions, 7–10. doi: https://doi.org/10.21303/2585-6847.2022.002671
- Simsek, A., Elciyar, K., Kizilhan, T. (2019). A Comparative Study on Social Media Addiction of High School and University Students. Contemporary Educational Technology, 10 (2), 106–119. doi: https://doi.org/10.30935/cet.554452
- Tunc-Aksan, A., Evin, S. (2019). Smartphone Addiction, Fear of Missing Out, and Perceived Competence as Predictors of Social Media Addiction of Adolescents. European Journal of Educational Research, 8 (2), 559–569. doi: https://doi.org/10.12973/eu-jer.8.2.559
- Recio Moreno, D., Gil Quintana, J., Romero Riaño, E. (2023). Impact and engagement of sport & fitness influencers: A challenge for health education media literacy. Online Journal of Communication and Media Technologies, 13 (3), e202334. doi: https://doi.org/10.30935/ojcmt/13309
- Lyu, H., Wang, J., Wu, W., Duong, V., Zhang, X., Dye, T. D., Luo, J. (2022). Social media study of public opinions on potential COVID-19 vaccines: informing dissent, disparities, and dissemination. Intelligent Medicine, 2 (1), 1–12. doi: https://doi.org/10.1016/j.imed.2021.08.001
- Sri, V. I. S. R., Niharika, Ch., Maneesh, K., Ismail, Dr. M. (2019). Sentiment Analysis of Patients’ Opinions in Healthcare using Lexicon-based Method. International Journal of Engineering and Advanced Technology, 9 (1), 6977–6981. doi: https://doi.org/10.35940/ijeat.a2141.109119
- Khan, M. T., Khalid, S. (2015). Sentiment Analysis for Health Care. International Journal of Privacy and Health Information Management, 3 (2), 78–91. doi: https://doi.org/10.4018/ijphim.2015070105
- Ilyasova, R. (2019). Fundamentals of Health Economics. Kazan: Kazan University Publishing House, 86. Available at: https://kpfu.ru/staff_files/F167974908/POSOBIE__KFU__Osnovy_EZ_Ilyasova_A.R._2019__1_.pdf
- Botha, Y. (2023). Determinants of social organizational credibility: Towards a formal conceptualization. Online Journal of Communication and Media Technologies, 13 (3), e202329. doi: https://doi.org/10.30935/ojcmt/13218
- Aattouchi, I., Elmendili, S., Elmendili, F. (2021). Sentiment Analysis of Health Care: Review. E3S Web of Conferences, 319, 01064. doi: https://doi.org/10.1051/e3sconf/202131901064
- Kausar, S., Huahu, X., Ahmad, W., Shabir, M. Y., Ahmad, W. (2020). A Sentiment Polarity Categorization Technique for Online Product Reviews. IEEE Access, 8, 3594–3605. doi: https://doi.org/10.1109/access.2019.2963020
- Hamdan, H., Bellot, P., Bechet, F. (2015). Sentiment Lexicon-Based Features for Sentiment Analysis in Short Text. Research in Computing Science, 90 (1), 217–226. doi: https://doi.org/10.1007/978-3-319-18117-2_9
- Baid, P., Gupta, A., Chaplot, N. (2017). Sentiment Analysis of Movie Reviews using Machine Learning Techniques. International Journal of Computer Applications, 179 (7), 45–49. doi: https://doi.org/10.5120/ijca2017916005
- Yadav, S., Shukla, S. (2016). Analysis of k-Fold Cross-Validation over Hold-Out Validation on Colossal Datasets for Quality Classification. 2016 IEEE 6th International Conference on Advanced Computing (IACC). doi: https://doi.org/10.1109/iacc.2016.25
- U.S. Hospital Customer Satisfaction 2016-2020. Available at: https://www.kaggle.com/datasets/abrambeyer/us-hospital-customer-satisfaction-20162020?select=cms_hospital_patient_satisfaction_2020.csv
- Prakash, P. R., Aruna, K. D. (2019). Business intelligence analytics using sentiment analysis-a survey. International Journal of Electrical and Computer Engineering (IJECE), 9 (1), 613. doi: https://doi.org/10.11591/ijece.v9i1.pp613-620
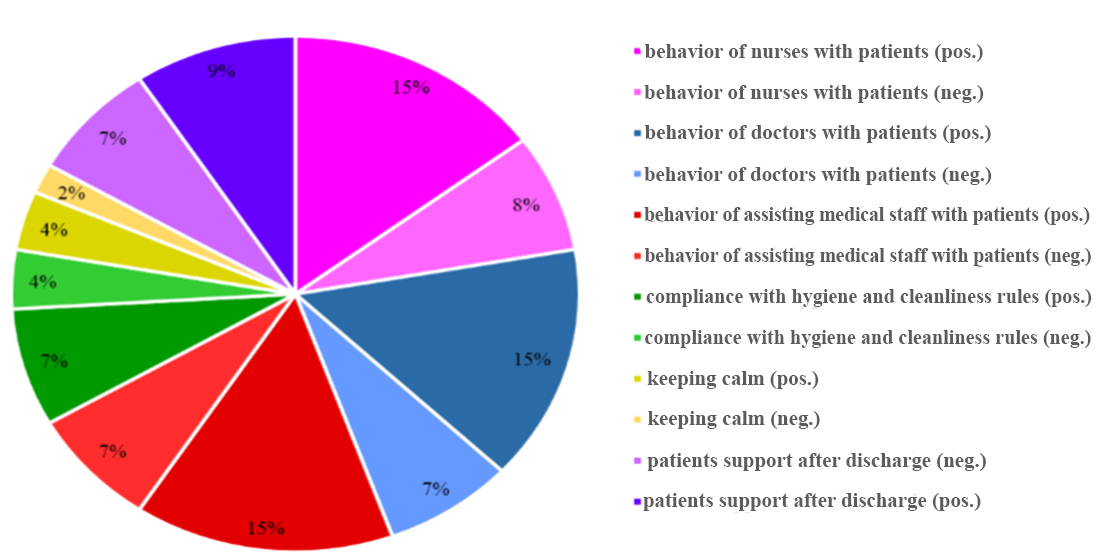
Downloads
Published
How to Cite
Issue
Section
License
Copyright (c) 2023 Masuma Mammadova, Zarifa Jabrayilova, Nargiz Shikhaliyeva

This work is licensed under a Creative Commons Attribution 4.0 International License.
The consolidation and conditions for the transfer of copyright (identification of authorship) is carried out in the License Agreement. In particular, the authors reserve the right to the authorship of their manuscript and transfer the first publication of this work to the journal under the terms of the Creative Commons CC BY license. At the same time, they have the right to conclude on their own additional agreements concerning the non-exclusive distribution of the work in the form in which it was published by this journal, but provided that the link to the first publication of the article in this journal is preserved.
A license agreement is a document in which the author warrants that he/she owns all copyright for the work (manuscript, article, etc.).
The authors, signing the License Agreement with TECHNOLOGY CENTER PC, have all rights to the further use of their work, provided that they link to our edition in which the work was published.
According to the terms of the License Agreement, the Publisher TECHNOLOGY CENTER PC does not take away your copyrights and receives permission from the authors to use and dissemination of the publication through the world's scientific resources (own electronic resources, scientometric databases, repositories, libraries, etc.).
In the absence of a signed License Agreement or in the absence of this agreement of identifiers allowing to identify the identity of the author, the editors have no right to work with the manuscript.
It is important to remember that there is another type of agreement between authors and publishers – when copyright is transferred from the authors to the publisher. In this case, the authors lose ownership of their work and may not use it in any way.