Determination of the number of clusters of normalized vegetation indices using the k-means algorithm
DOI:
https://doi.org/10.15587/1729-4061.2023.290129Keywords:
NVDI, vegetation index, cluster analysis, k-means algorithm, remote sensing dataAbstract
The process of clustering of normalized vegetation indices in five regions with a total area of 2565 hectares of the North Kazakhstan region was studied. A methodological approach to organizing the clustering process is proposed using the vegetation indices NDVI, MSAVI, ReCI, NDWI and NDRE, taking into account individual characteristics in the three main phases of spring wheat development
As a result of the research, vegetation indices were grouped into 3 classes using the k-means clustering method. The first cluster contained vegetation indices whose maximum values occupied about 33.98% of the total area of the study area. It was found that NDVImax located in the first cluster was positively correlated with soil-corrected vegetation indices MSAVI and crop moisture indicators NDMI (R2=0.92). The second cluster is characterized by minimum values of NDVImax coefficients at the germination, tillering and ripening phases (from 0.53 to 0.55). The lowest values of vegetation indices occupied 35.9 % in the germination phase, 37.9 % in the tillering phase, and 40.1 % of the field from the total area. The third cluster is characterized by average values of vegetation indices in all three phases. A correlation matrix was also constructed to assess the closeness of the relationship between actual yield and NDVI vegetation indices. The maximum coefficient was obtained at the germination phase, R=0.94 with a minimum significance coefficient p=0.018.
The approach used in this study can be useful in the analysis of satellite data, as it can improve the sensitivity of the constellation procedure. From a practical point of view, the results obtained make it possible to assess the condition of agricultural crops in the early stages of the growing season, which makes it possible to improve their productivity based on the results of cluster analysis
References
- Mutanga, O., Masenyama, A., Sibanda, M. (2023). Spectral saturation in the remote sensing of high-density vegetation traits: A systematic review of progress, challenges, and prospects. ISPRS Journal of Photogrammetry and Remote Sensing, 198, 297–309. doi: https://doi.org/10.1016/j.isprsjprs.2023.03.010
- Xue, J., Su, B. (2017). Significant Remote Sensing Vegetation Indices: A Review of Developments and Applications. Journal of Sensors, 2017, 1–17. doi: https://doi.org/10.1155/2017/1353691
- Xiao, X., Braswell, B., Zhang, Q., Boles, S., Frolking, S., Moore, B. (2003). Sensitivity of vegetation indices to atmospheric aerosols: continental-scale observations in Northern Asia. Remote Sensing of Environment, 84 (3), 385–392. doi: https://doi.org/10.1016/s0034-4257(02)00129-3
- Mimenbayeva, A., Zhukabayeva, T. (2020). A review of free resources for processing and analyzing geospatial data. Proceedings of the 6th International Conference on Engineering & MIS 2020. doi: https://doi.org/10.1145/3410352.3410800
- Liu, D., Yang, F., Liu, S. (2021). Estimating wheat fractional vegetation cover using a density peak k-means algorithm based on hyperspectral image data. Journal of Integrative Agriculture, 20 (11), 2880–2891. doi: https://doi.org/10.1016/s2095-3119(20)63556-0
- Komarov, A. A., Kirsanov, A. D., Malashin, S. N. (2021). Comparative characteristics of various vegetation indices (vi) when the vegetation cover state of forage grasses assessing. Izvestya of Saint-Petersburg State Agrarian University, 63 (2), 18–29. doi: https://doi.org/10.24412/2078-1318-2021-2-18-29
- Somvanshi, S. S., Kumari, M. (2020). Comparative analysis of different vegetation indices with respect to atmospheric particulate pollution using sentinel data. Applied Computing and Geosciences, 7, 100032. doi: https://doi.org/10.1016/j.acags.2020.100032
- Ntayagabiri, J. P., Ndikumagenge, J., Ndayisaba, L., Philippe, B. K. (2023). Study on the Development and Implementation of Different Big Data Clustering Methods. Open Journal of Applied Sciences, 13 (07), 1163–1177. doi: https://doi.org/10.4236/ojapps.2023.137092
- Tlebaldinova, A. S., Ponkina, Ye. V., Mansurova, M. Ye., Ixanov, S. Sh. (2021). Using satellite images to assess the state of arable fields on the example of the East Kazakhstan region. Bulletin of the National Engineering Academy of the Republic of Kazakhstan, 82 (4), 179–186. doi: https://doi.org/10.47533/2020.1606-146x.128
- Pandey, S., Khanna, P. (2014). A hierarchical clustering approach for image datasets. 2014 9th International Conference on Industrial and Information Systems (ICIIS). doi: https://doi.org/10.1109/iciinfs.2014.7036504
- Prasad, M., Thota, S. (2023). Buddy System Based Alpha Numeric Weight Based Clustering Algorithm with User Threshold. doi: https://doi.org/10.20944/preprints202308.1676.v1
- Khudov, H., Makoveichuk, O., Komarov, V., Khudov, V., Khizhnyak, I., Bashynskyi, V. et al. (2023). Determination of the number of clusters on images from space optic-electronic observation systems using the k-means algorithm. Eastern-European Journal of Enterprise Technologies, 3 (9 (123)), 60–69. doi: https://doi.org/10.15587/1729-4061.2023.282374
- Vandana, B., Kumar, S. S. (2019). Hybrid K Mean Clustering Algorithm for Crop Production Analysis in Agriculture. Special Issue, 9 (2S), 9–13. doi: https://doi.org/10.35940/ijitee.b1002.1292s19
- Umarani, R., Tamilarasi, P. (2019). Data analysis of crop yield prediction using k-means clustering algorithm. Journal of Emerging Technologies and Innovative Research, 6 (4), 535–538. Available at: https://www.jetir.org/papers/JETIR1904582.pdf
- Marino, S., Alvino, A. (2021). Vegetation Indices Data Clustering for Dynamic Monitoring and Classification of Wheat Yield Crop Traits. Remote Sensing, 13 (4), 541. doi: https://doi.org/10.3390/rs13040541
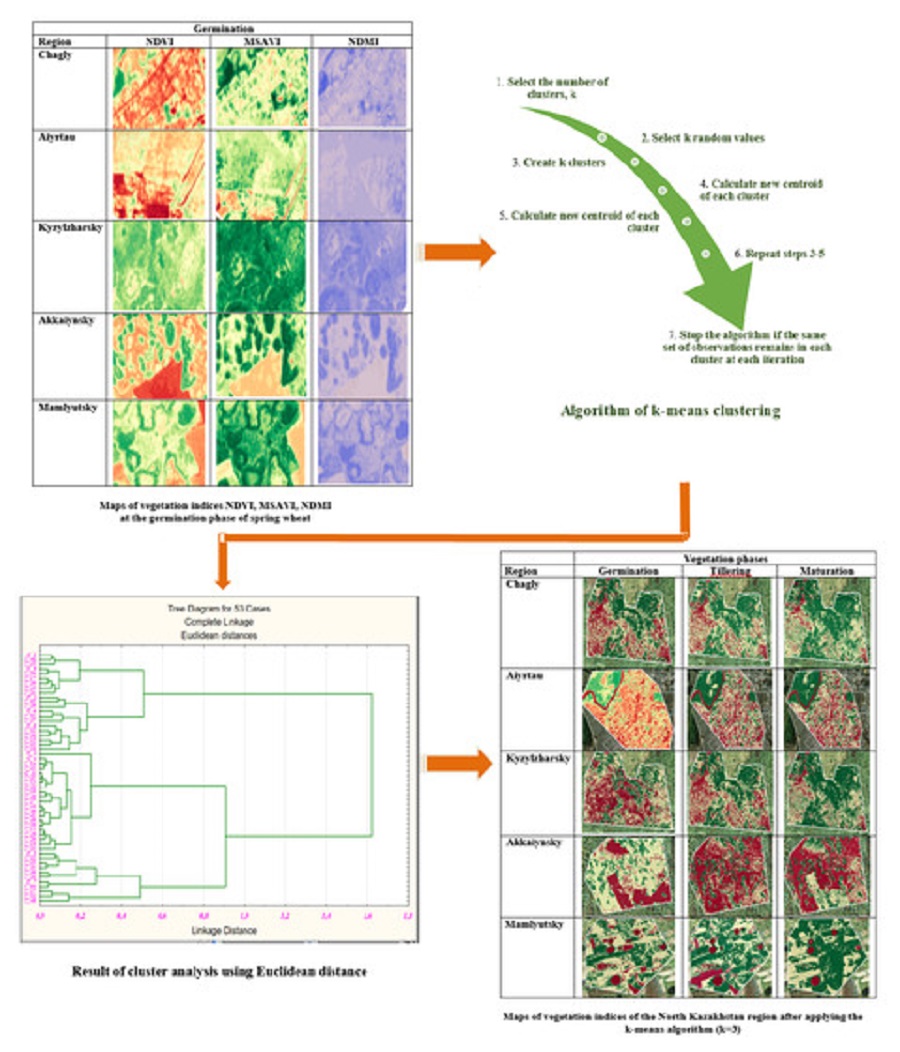
Downloads
Published
How to Cite
Issue
Section
License
Copyright (c) 2023 Aigul Mimenbayeva, Samat Artykbayev, Raya Suleimenova, Gulnar Abdygalikova, Akgul Naizagarayeva, Aisulu Ismailova

This work is licensed under a Creative Commons Attribution 4.0 International License.
The consolidation and conditions for the transfer of copyright (identification of authorship) is carried out in the License Agreement. In particular, the authors reserve the right to the authorship of their manuscript and transfer the first publication of this work to the journal under the terms of the Creative Commons CC BY license. At the same time, they have the right to conclude on their own additional agreements concerning the non-exclusive distribution of the work in the form in which it was published by this journal, but provided that the link to the first publication of the article in this journal is preserved.
A license agreement is a document in which the author warrants that he/she owns all copyright for the work (manuscript, article, etc.).
The authors, signing the License Agreement with TECHNOLOGY CENTER PC, have all rights to the further use of their work, provided that they link to our edition in which the work was published.
According to the terms of the License Agreement, the Publisher TECHNOLOGY CENTER PC does not take away your copyrights and receives permission from the authors to use and dissemination of the publication through the world's scientific resources (own electronic resources, scientometric databases, repositories, libraries, etc.).
In the absence of a signed License Agreement or in the absence of this agreement of identifiers allowing to identify the identity of the author, the editors have no right to work with the manuscript.
It is important to remember that there is another type of agreement between authors and publishers – when copyright is transferred from the authors to the publisher. In this case, the authors lose ownership of their work and may not use it in any way.