Devising an approach for the automated restoration of shipmaster’s navigational qualification parameters under risk conditions
DOI:
https://doi.org/10.15587/1729-4061.2024.296955Keywords:
cognitive automation, qualification parameters, navigation risks, sea transport, human factorAbstract
The object of this study is the safety control system of ship management, by identifying and restoring the qualification parameters of shipmasters in critical situations.
The task solved in the study is the timely determination of an insufficient level of qualification for the performance of certain operations in controlling the movement of the ship, by applying a formal-logical model of detecting the intuitive actions of the operator-shipmaster and gradually restoring his/her qualification parameters using the devised method.
The stages of development and the formal-logical structure of the model and method in terms of cognitive automation were described in detail as the study results. It was possible to ensure early detection of risks when controlling the movement of the ship in 56 % of cases, during a laboratory experiment on simulators, which in 24 % of cases turned out to be particularly dangerous.
The interpretation of the results involved algorithmizing complex and formalized data on the actions of operators and the application of the method of restoring their qualification parameters, which allowed a comprehensive approach to safety management.
The distinguishing features of the findings were to predict the level of danger by simulating maritime operations with input navigational and individual conditions. This made it possible to improve the effectiveness of operations to 89 %, reduce the phenomenon of loss of control over the course to 32 %, reduce critical situations to 7 % and the cost of resources.
The scope and conditions of practical use involve a comprehensive assessment of external and internal influences on the level of danger, delay in decision-making by operators, as well as sailing conditions. The simulation results could be used to devise strategies for planning maneuvers, predicting risks, and developing maritime security systems
References
- Mahbub Tusher, H., Haque Munim, Z., Hussain, S., Nazir, S. (2023). An automated machine learning approach for early identification of at-risk maritime students. AHFE International. https://doi.org/10.54941/ahfe1003150
- Engel, C., Elshan, E., Ebel, P., Leimeister, J. M. (2023). Stairway to heaven or highway to hell: A model for assessing cognitive automation use cases. Journal of Information Technology. https://doi.org/10.1177/02683962231185599
- Miller, M., Holley, S. (2023). Deficits in Cognitive Resilience of Commercial Pilots: The Case For a CRM Tenet on Computer Information and Automation in Digital Flight Operations. AHFE International. https://doi.org/10.54941/ahfe1003921
- Khatun, M., Wagner, F., Jung, R., Glaß, M. (2023). Identification of Interface Related Factors Between Safety Management System and Cybersecurity Management System for Highly Automated Driving Vehicles. Proceedings of the 25th International Conference on Enterprise Information Systems. https://doi.org/10.5220/0011708700003467
- Gaitanidou, E., Loukea, M., Alertas, I., Spanidis, P., Bekiaris, E. (2023). E-Learning Training Tool for Automated Transport Systems. Advances in Intelligent Traffic and Transportation Systems. https://doi.org/10.3233/atde230004
- Chang, Z., He, X., Fan, H., Guan, W., He, L. (2023). Leverage Bayesian Network and Fault Tree Method on Risk Assessment of LNG Maritime Transport Shipping Routes: Application to the China–Australia Route. Journal of Marine Science and Engineering, 11 (9), 1722. https://doi.org/10.3390/jmse11091722
- Múller-Plath, G., Lehleitner, J., Maier, J., Silva-Lóbling, J., Zhang, H., Zhang, X., Zhou, S. (2023). How Does Maritime Situation Awareness Depend on Navigation Automation and Mental Workload? A Sea Simulator Experiment. TransNav, the International Journal on Marine Navigation and Safety of Sea Transportation, 17 (4), 963–969. https://doi.org/10.12716/1001.17.04.23
- Engel, C., Ebel, P., Leimeister, J. M. (2022). Cognitive automation. Electronic Markets, 32 (1), 339–350. https://doi.org/10.1007/s12525-021-00519-7
- Brcko, T., Androjna, A., Srše, J., Boć, R. (2021). Vessel Multi-Parametric Collision Avoidance Decision Model: Fuzzy Approach. Journal of Marine Science and Engineering, 9 (1), 49. https://doi.org/10.3390/jmse9010049
- Cao, Q., Wang, B., Dong, G., Hu, K., Yang, H. (2022). Operator Optimization Oriented Person Re-Identification. Journal of Physics: Conference Series, 2284 (1), 012019. https://doi.org/10.1088/1742-6596/2284/1/012019
- Grasmeier, M., Tadić, T. (2023). Enhancing Maritime Safety Training Through Active Learning: The Theoretical Framework and Prototype Development of the Virtual Training Vessel. Available at: https://www.researchgate.net/publication/376167960_Enhancing_Maritime_Safety_Training_Through_Active_Learning_The_Theoretical_Framework_and_Prototype_Development_of_the_Virtual_Training_Vessel
- Wishart, J., Zhao, J., Woodard, B., O’Malley, G., Guo, H., Rahimi, S., Swaminathan, S. (2023). A Proposed Safety Case Framework for Automated Vehicle Safety Evaluation. 2023 IEEE International Automated Vehicle Validation Conference (IAVVC). https://doi.org/10.1109/iavvc57316.2023.10328077
- Danish, A., Veena, D., Samad, D. A., Faiza, J., Hira, K., Tayyaba, S..(2023). Enhancing Home Automation through Brain-Computer Interface Technology. Xi'an Shiyou Daxue Xuebao (Ziran Kexue Ban)/Journal of Xi'an Shiyou University, 19 (12), 217–223. Available at: https://www.researchgate.net/publication/376272436_Enhancing_Home_Automation_through_Brain-Computer_Interface_Technology
- Griffiths, T. L., Callaway, F., Chang, M. B., Grant, E., Krueger, P. M., Lieder, F. (2019). Doing more with less: meta-reasoning and meta-learning in humans and machines. Current Opinion in Behavioral Sciences, 29, 24–30. https://doi.org/10.1016/j.cobeha.2019.01.005
- Kelly, A. (2017). Think Twice: Review of Thinking, Fast and Slow by Daniel Kahneman (2011). Numeracy, 10 (2). https://doi.org/10.5038/1936-4660.10.2.15
- Prokopchuk, Y. (2022). Intuition: The Experience of Formal Research. Dnipro: PSACEA Press, 724. https://doi.org/10.13140/RG.2.2.12215.39841
- Popovych, I., Halian, I., Lialiuk, G., Chopyk, R., Karpenko, Ye., Melnyk, Yu. (2022). Research of young female handball players’ self-actualizing mental states. Journal of Physical Education and Sport, 22 (7), 1599–1607. https://doi.org/10.7752/jpes.2022.07201
- Popovych, I., Plokhikh, V., Hrys, A., Pavliuk, M., Nosov, P., Zinchenko, S. (2023). Operationalization of footballers’ emotional intelligence in the dimensions of motivational orientation: analysis based on the basic positions. Journal of Physical Education and Sport, 23 (3), 772–781. https://doi.org/10.7752/jpes.2023.03095
- Blynova, O., Derevianko, S., Ivanova, O., Popovych, I., Estay Sepúlveda, J. G. (2022). Demanda profesional de trabajadores migrantes potenciales. Revista Notas Históricas Y Geográficas, 88–106. Available at: https://www.revistanotashistoricasygeograficas.cl/index.php/nhyg/article/view/435
- Solovey, O., Ben, A., Dudchenko, S., Nosov, P. (2020). Development of control model for loading operations on heavy lift vessels based on inverse algorithm. Eastern-European Journal of Enterprise Technologies, 5 (2 (107)), 48–56. https://doi.org/10.15587/1729-4061.2020.214856
- Nosov, P., Zinchenko, S., Ben, A., Prokopchuk, Y., Mamenko, P., Popovych, I. et al. (2021). Navigation safety control system development through navigator action prediction by data mining means. Eastern-European Journal of Enterprise Technologies, 2 (9 (110)), 55–68. https://doi.org/10.15587/1729-4061.2021.229237
- Nosov, P., Zinchenko, S., Plokhikh, V., Popovych, I., Prokopchuk, Y., Makarchuk, D. et al. (2021). Development and experimental study of analyzer to enhance maritime safety. Eastern-European Journal of Enterprise Technologies, 4 (3 (112)), 27–35. https://doi.org/10.15587/1729-4061.2021.239093
- Nosov, P., Koretsky, O., Zinchenko, S., Prokopchuk, Y., Gritsuk, I., Sokol, I., Kyrychenko, K. (2023). Devising an approach to safety management of vessel control through the identification of navigator’s state. Eastern-European Journal of Enterprise Technologies, 4 (3 (124)), 19–32. https://doi.org/10.15587/1729-4061.2023.286156
- Plokhikh, V. V. (2023). Relationship between coping behavior and students’ perceptions of the passage of time. Insight: the psychological dimensions of society, 9, 72–93. Available at: https://insight.journal.kspu.edu/index.php/insight/article/view/124/93
- Kalenchuk, V. O., Fedchuk, O. V., Mykhaylyuk, V. P. (2023). Relationship between corporate culture and psychological safety of training and educational space for young female athletes. Insight: the psychological dimensions of society, 9, 113–129. Available at: https://insight.journal.kspu.edu/index.php/insight/article/view/127/95
- Fan, S., Ji, T., Gordon, W., Rickard, B. (2013). Forecasting Baltic Dirty Tanker Index by Applying Wavelet Neural Networks. Journal of Transportation Technologies, 03 (01), 68–87. https://doi.org/10.4236/jtts.2013.31008
- Zhuravlova, L. P., Lytvynchuk, A. I., Grechukha, I. A., Bedny, I. S. (2023). Subclinical personal correlates of psychological safety. Insight: the psychological dimensions of society, 9, 94–111. Available at: https://insight.journal.kspu.edu/index.php/insight/article/view/125/94
- Popovych, I., Hulias, I., Serbin, I., Piletska, L., Mashchak, S., Zahrai, L. (2023). Psychological content parameters of attention in the structure of time perspective of young female athletes: comparative analysis. Journal of Physical Education and Sport, 23 (1), 152–161. https://doi.org/10.7752/jpes.2023.01019
- Serhii, Z., Oleh, T., Pavlo, N., Ihor, P., Kostiantyn, K. (2022). Pivot Point position determination and its use for manoeuvring a vessel. Ships and Offshore Structures, 18 (3), 358–364. https://doi.org/10.1080/17445302.2022.2052480
- Wei, H., Wei, X., Guo, J., Zhou, J. (2022). Application of PID in Position Control of Multi-Articulated Robotic Arms in Rock Drilling Trolleys. Mechatronics and Automation Technology. https://doi.org/10.3233/atde221159
- Abougarair, A. J., Aburakhis, M. K. I., Edardar, M. M. (2022). Adaptive Neural Networks Based Robust Output Feedback Controllers for Nonlinear Systems. International Journal of Robotics and Control Systems, 2 (1), 37–56. https://doi.org/10.31763/ijrcs.v2i1.523
- Yu, Y., Adu, K., Tashi, N., Anokye, P., Wang, X., Ayidzoe, M. A. (2020). RMAF: Relu-Memristor-Like Activation Function for Deep Learning. IEEE Access, 8, 72727–72741. https://doi.org/10.1109/access.2020.2987829
- Kamran, Shah, F. A., Aly, W. H. F., Aksoy, H., Alotaibi, F. M., Mahariq, I. (2022). Numerical Inverse Laplace Transform Methods for Advection-Diffusion Problems. Symmetry, 14 (12), 2544. https://doi.org/10.3390/sym14122544
- Shutovskyi, A. (2023). Some applied aspects of the Dirac delta function. Ukrainian Mathematical Bulletin, 20 (3), 442–453. https://doi.org/10.37069/1810-3200-2023-20-3-7
- Higaki, T., Hashimoto, H., Yoshioka, H. (2022). Investigation and Imitation of Human Captains’ Maneuver Using Inverse Reinforcement Learning. https://doi.org/10.21203/rs.3.rs-1844861/v1
- Ren, B., Guan, W., Zhou, Q., Wang, Z. (2023). EEG-Based Driver Fatigue Monitoring within a Human–Ship–Environment System: Implications for Ship Braking Safety. Sensors, 23 (10), 4644. https://doi.org/10.3390/s23104644
- Lyu, H., Yue, J., Zhang, W., Cheng, T., Yin, Y., Yang, X. et al. (2023). Fatigue Detection for Ship OOWs Based on Input Data Features, From the Perspective of Comparison With Vehicle Drivers: A Review. IEEE Sensors Journal, 23 (14), 15239–15252. https://doi.org/10.1109/jsen.2023.3281068
- Chen, X., Wei, C., Xin, Z., Zhao, J., Xian, J. (2023). Ship Detection under Low-Visibility Weather Interference via an Ensemble Generative Adversarial Network. Journal of Marine Science and Engineering, 11 (11), 2065. https://doi.org/10.3390/jmse11112065
- Biočić, T., Frančić, V., Hasanspahić, N., Maglić, L. (2023). The Analysis of the Deficiencies Resulting from Paris MoU PSC Inspections. Naše More, 70 (4), 228–238. https://doi.org/10.17818/nm/2023/4.5
- Wang, Z., Wu, Y., Chu, X., Liu, C., Zheng, M. (2023). Risk Identification Method for Ship Navigation in the Complex Waterways via Consideration of Ship Domain. Journal of Marine Science and Engineering, 11 (12), 2265. https://doi.org/10.3390/jmse11122265
- Zhang, D., Chu, X., Liu, C., He, Z., Zhang, P., Wu, W. (2024). A Review on Motion Prediction for Intelligent Ship Navigation. Journal of Marine Science and Engineering, 12 (1), 107. https://doi.org/10.3390/jmse12010107
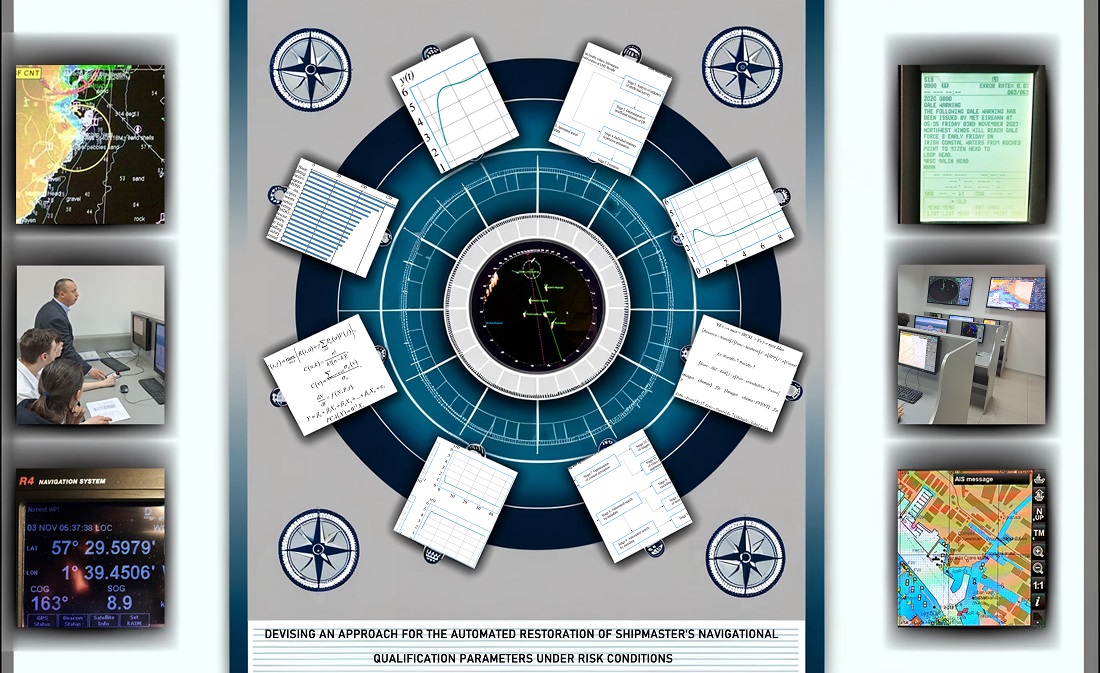
Downloads
Published
How to Cite
Issue
Section
License
Copyright (c) 2024 Victoria Ponomaryova, Pavlo Nosov, Andrii Ben, Ihor Popovych, Yurii Prokopchuk, Pavlo Mamenko, Sergiy Dudchenko, Eduard Appazov, Ihor Sokol

This work is licensed under a Creative Commons Attribution 4.0 International License.
The consolidation and conditions for the transfer of copyright (identification of authorship) is carried out in the License Agreement. In particular, the authors reserve the right to the authorship of their manuscript and transfer the first publication of this work to the journal under the terms of the Creative Commons CC BY license. At the same time, they have the right to conclude on their own additional agreements concerning the non-exclusive distribution of the work in the form in which it was published by this journal, but provided that the link to the first publication of the article in this journal is preserved.
A license agreement is a document in which the author warrants that he/she owns all copyright for the work (manuscript, article, etc.).
The authors, signing the License Agreement with TECHNOLOGY CENTER PC, have all rights to the further use of their work, provided that they link to our edition in which the work was published.
According to the terms of the License Agreement, the Publisher TECHNOLOGY CENTER PC does not take away your copyrights and receives permission from the authors to use and dissemination of the publication through the world's scientific resources (own electronic resources, scientometric databases, repositories, libraries, etc.).
In the absence of a signed License Agreement or in the absence of this agreement of identifiers allowing to identify the identity of the author, the editors have no right to work with the manuscript.
It is important to remember that there is another type of agreement between authors and publishers – when copyright is transferred from the authors to the publisher. In this case, the authors lose ownership of their work and may not use it in any way.