The dependence of the effectiveness of neural networks for recognizing human voice on language
DOI:
https://doi.org/10.15587/1729-4061.2024.298687Keywords:
Artificial intelligence, neural networks, CNN, RNN, MLP, voice activity detector, human voice recognition, the effectiveness of training, language specifics, recognition accuracyAbstract
This study examines the effectiveness of neural network architectures (multilayer perceptron MLP, convolutional neural network CNN, recurrent neural network RNN) for human voice recognition, with an emphasis on the Kazakh language. Problems related to language, the difference between speakers, and the influence of network architecture on recognition accuracy are considered. The methodology includes extensive training and testing, studying the accuracy of recognition in different languages, and different sets of data on speakers. Using a comparative analysis, this study evaluates the performance of three architectures trained exclusively in the Kazakh language. The testing included statements in Kazakhs and other languages, while the number of speakers varied to assess its impact on recognition accuracy.
During the study, the results showed that CNN neural networks are more effective in recognizing human voice than RNN and MLP. Also, it was found that the CNN has a higher accuracy in recognizing the human voice in the Kazakh language, both for a small and for a large number of announcers. For example, for 20 speakers, the recognition error in Russian was 21.86 %, whereas in Kazakhs it was 10.6 %. A similar trend was observed for 80 speakers: 16.2 % Russians and 8.3 % Kazakhs. It can also be argued that learning one language does not guarantee high recognition accuracy in other languages. Therefore, the accuracy of human voice recognition by neural networks depends significantly on the language in which training is conducted.
In addition, this study highlights the importance of different sets of speaker data to achieve optimal results. This knowledge is crucial for advancing the development of reliable human voice recognition systems that can accurately identify different human voices in different language contexts
References
- Mihalache, S., Burileanu, D. (2022). Using Voice Activity Detection and Deep Neural Networks with Hybrid Speech Feature Extraction for Deceptive Speech Detection. Sensors, 22 (3), 1228. https://doi.org/10.3390/s22031228
- Lee, Y., Min, J., Han, D. K., Ko, H. (2020). Spectro-Temporal Attention-Based Voice Activity Detection. IEEE Signal Processing Letters, 27, 131–135. https://doi.org/10.1109/lsp.2019.2959917
- Sofer, A., Chazan, S. E. (2022). CNN self-attention voice activity detector. arXiv. https://doi.org/10.48550/arXiv.2203.02944
- Zhang, X.-L., Xu, M. (2022). AUC optimization for deep learning-based voice activity detection. EURASIP Journal on Audio, Speech, and Music Processing, 2022 (1). https://doi.org/10.1186/s13636-022-00260-9
- Jia, F., Majumdar, S., Ginsburg, B. (2021). MarbleNet: Deep 1D Time-Channel Separable Convolutional Neural Network for Voice Activity Detection. ICASSP 2021 - 2021 IEEE International Conference on Acoustics, Speech and Signal Processing (ICASSP). https://doi.org/10.1109/icassp39728.2021.9414470
- Heo, Y., Lee, S. (2023). Supervised Contrastive Learning for Voice Activity Detection. Electronics, 12 (3), 705. https://doi.org/10.3390/electronics12030705
- Faghani, M., Rezaee-Dehsorkh, H., Ravanshad, N., Aminzadeh, H. (2023). Ultra-Low-Power Voice Activity Detection System Using Level-Crossing Sampling. Electronics, 12 (4), 795. https://doi.org/10.3390/electronics12040795
- Lee, G. W., Kim, H. K. (2020). Multi-Task Learning U-Net for Single-Channel Speech Enhancement and Mask-Based Voice Activity Detection. Applied Sciences, 10 (9), 3230. https://doi.org/10.3390/app10093230
- Arslan, O., Engin, E. Z. (2019). Noise Robust Voice Activity Detection Based on Multi-Layer Feed-Forward Neural Network. Electrica, 19 (2), 91–100. https://doi.org/10.26650/electrica.2019.18042
- Oh, Y. R., Park, K., Park, J. G. (2020). Online Speech Recognition Using Multichannel Parallel Acoustic Score Computation and Deep Neural Network (DNN)- Based Voice-Activity Detector. Applied Sciences, 10 (12), 4091. https://doi.org/10.3390/app10124091
- Sehgal, A., Kehtarnavaz, N. (2018). A Convolutional Neural Network Smartphone App for Real-Time Voice Activity Detection. IEEE Access, 6, 9017–9026. https://doi.org/10.1109/access.2018.2800728
- Mukherjee, H., Obaidullah, Sk. Md., Santosh, K. C., Phadikar, S., Roy, K. (2018). Line spectral frequency-based features and extreme learning machine for voice activity detection from audio signal. International Journal of Speech Technology, 21 (4), 753–760. https://doi.org/10.1007/s10772-018-9525-6
- Ali, Z., Talha, M. (2018). Innovative Method for Unsupervised Voice Activity Detection and Classification of Audio Segments. IEEE Access, 6, 15494–15504. https://doi.org/10.1109/access.2018.2805845
- Jung, Y., Kim, Y., Choi, Y., Kim, H. (2018). Joint Learning Using Denoising Variational Autoencoders for Voice Activity Detection. Interspeech 2018. https://doi.org/10.21437/interspeech.2018-1151
- Yoshimura, T., Hayashi, T., Takeda, K., Watanabe, S. (2020). End-to-End Automatic Speech Recognition Integrated with CTC-Based Voice Activity Detection. ICASSP 2020 - 2020 IEEE International Conference on Acoustics, Speech and Signal Processing (ICASSP). https://doi.org/10.1109/icassp40776.2020.9054358
- Bredin, H., Laurent, A. (2021). End-To-End Speaker Segmentation for Overlap-Aware Resegmentation. Interspeech 2021. https://doi.org/10.21437/interspeech.2021-560
- Lavechin, M., Gill, M.-P., Bousbib, R., Bredin, H., Garcia-Perera, L. P. (2020). End-to-End Domain-Adversarial Voice Activity Detection. Interspeech 2020. https://doi.org/10.21437/interspeech.2020-2285
- Cornell, S., Omologo, M., Squartini, S., Vincent, E. (2020). Detecting and Counting Overlapping Speakers in Distant Speech Scenarios. Interspeech 2020. https://doi.org/10.21437/interspeech.2020-2671
- Tan, X., Zhang, X.-L. (2021). Speech Enhancement Aided End-To-End Multi-Task Learning for Voice Activity Detection. ICASSP 2021 - 2021 IEEE International Conference on Acoustics, Speech and Signal Processing (ICASSP). https://doi.org/10.1109/icassp39728.2021.9414445
- Varzandeh, R., Adiloglu, K., Doclo, S., Hohmann, V. (2020). Exploiting Periodicity Features for Joint Detection and DOA Estimation of Speech Sources Using Convolutional Neural Networks. ICASSP 2020 - 2020 IEEE International Conference on Acoustics, Speech and Signal Processing (ICASSP). https://doi.org/10.1109/icassp40776.2020.9054754
- Medetov, B., Kulakayeva, A., Zhetpisbayeva, A., Albanbay, N., Kabduali, T. (2023). Identifying the regularities of the signal detection method using the Kalman filter. Eastern-European Journal of Enterprise Technologies, 5 (9 (125)), 26–34. https://doi.org/10.15587/1729-4061.2023.289472
- Mussakhojayeva, S., Khassanov, Y., Atakan Varol, H. (2022). KSC2: An Industrial-Scale Open-Source Kazakh Speech Corpus. Interspeech 2022. https://doi.org/10.21437/interspeech.2022-421
- Mussakhojayeva, S., Khassanov, Y., Atakan Varol, H. (2021). A Study of Multilingual End-to-End Speech Recognition for Kazakh, Russian, and English. Lecture Notes in Computer Science, 448–459. https://doi.org/10.1007/978-3-030-87802-3_41
- Mussakhojayeva, S., Dauletbek, K., Yeshpanov, R., Varol, H. A. (2023). Multilingual Speech Recognition for Turkic Languages. Information, 14 (2), 74. https://doi.org/10.3390/info14020074
- Musaev, M., Mussakhojayeva, S., Khujayorov, I., Khassanov, Y., Ochilov, M., Atakan Varol, H. (2021). USC: An Open-Source Uzbek Speech Corpus and Initial Speech Recognition Experiments. Lecture Notes in Computer Science, 437–447. https://doi.org/10.1007/978-3-030-87802-3_40
- Ardila, R., Branson, M., Davis, K., Henretty, M., Kohler, M., Meyer, J. et al. (2020). Common voice: A massively-multilingualspeech corpus. arXiv. https://doi.org/10.48550/arXiv.1912.06670
- Medetov, B., Serikov, T., Tolegenova, A., Zhexebay, D., Yskak, A., Namazbayev, T., Albanbay, N. (2023). Development of a model for determining the necessary FPGA computing resource for placing a multilayer neural network on it. Eastern-European Journal of Enterprise Technologies, 4 (4 (124)), 34–45. https://doi.org/10.15587/1729-4061.2023.281731
- Aigul, K., Altay, A., Yevgeniya, D., Bekbolat, M., Zhadyra, O. (2022). Improvement of Signal Reception Reliability at Satellite Spectrum Monitoring System. IEEE Access, 10, 101399–101407. https://doi.org/10.1109/access.2022.3206953
- Aitmagambetov, A., Butuzov, Y., Butuzov, Y., Tikhvinskiy, V., Tikhvinskiy, V., Kulakayeva, A. et al. (2021). Energy budget and methods for determining coordinates for a radiomonitoring system based on a small spacecraft. Indonesian Journal of Electrical Engineering and Computer Science, 21 (2), 945. https://doi.org/10.11591/ijeecs.v21.i2.pp945-956
- Albanbay, N., Medetov, B., Zaks, M. A. (2021). Exponential distribution of lifetimes for transient bursting states in coupled noisy excitable systems. Chaos: An Interdisciplinary Journal of Nonlinear Science, 31 (9). https://doi.org/10.1063/5.0059102
- Albanbay, N., Medetov, B., Zaks, M. A. (2020). Statistics of Lifetimes for Transient Bursting States in Coupled Noisy Excitable Systems. Journal of Computational and Nonlinear Dynamics, 15 (12). https://doi.org/10.1115/1.4047867
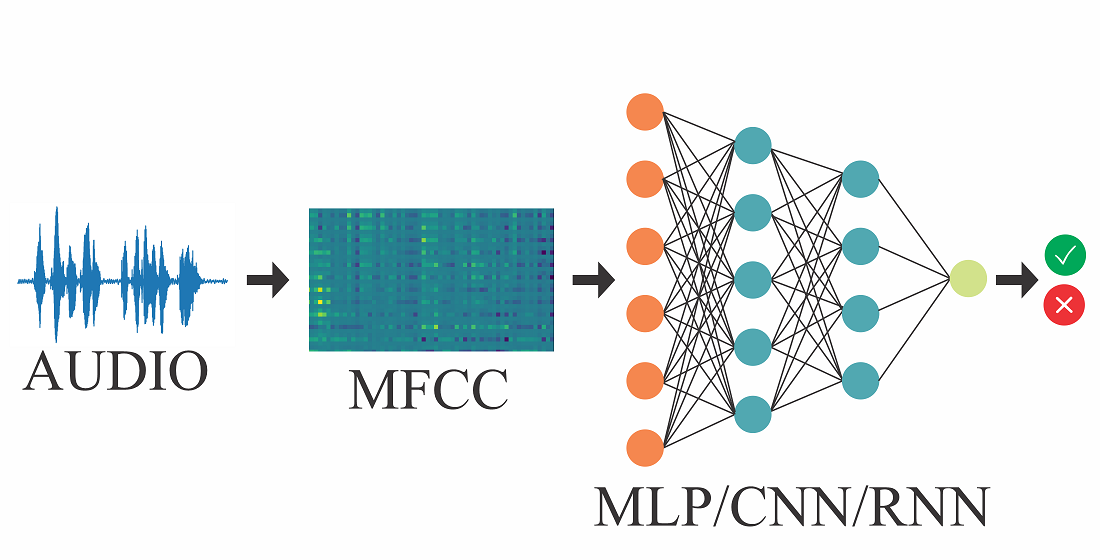
Downloads
Published
How to Cite
Issue
Section
License
Copyright (c) 2024 Aigul Nurlankyzy, Ainur Akhmediyarova, Ainur Zhetpisbayeva, Timur Namazbayev, Asset Yskak, Nurdaulet Yerzhan, Bekbolat Medetov

This work is licensed under a Creative Commons Attribution 4.0 International License.
The consolidation and conditions for the transfer of copyright (identification of authorship) is carried out in the License Agreement. In particular, the authors reserve the right to the authorship of their manuscript and transfer the first publication of this work to the journal under the terms of the Creative Commons CC BY license. At the same time, they have the right to conclude on their own additional agreements concerning the non-exclusive distribution of the work in the form in which it was published by this journal, but provided that the link to the first publication of the article in this journal is preserved.
A license agreement is a document in which the author warrants that he/she owns all copyright for the work (manuscript, article, etc.).
The authors, signing the License Agreement with TECHNOLOGY CENTER PC, have all rights to the further use of their work, provided that they link to our edition in which the work was published.
According to the terms of the License Agreement, the Publisher TECHNOLOGY CENTER PC does not take away your copyrights and receives permission from the authors to use and dissemination of the publication through the world's scientific resources (own electronic resources, scientometric databases, repositories, libraries, etc.).
In the absence of a signed License Agreement or in the absence of this agreement of identifiers allowing to identify the identity of the author, the editors have no right to work with the manuscript.
It is important to remember that there is another type of agreement between authors and publishers – when copyright is transferred from the authors to the publisher. In this case, the authors lose ownership of their work and may not use it in any way.