Залежність ефективності нейромереж для розпізнавання людського голосу в залежності від мови
DOI:
https://doi.org/10.15587/1729-4061.2024.298687Ключові слова:
штучний інтелект, нейронні мережі, ЗНМ, РНМ, БШП, детектор голосової активності, розпізнавання людського голосу, ефективність навчання, мовні особливості, точність розпізнаванняАнотація
У цьому дослідженні розглядається ефективність архітектур нейронних мереж (багатошаровий персептрон БШП, згорткова нейронна мережа ЗНМ, рекурентна нейронна мережа РНМ) для розпізнавання голосу людини, з акцентом на казахську мову. Розглядаються проблеми, пов’язані з мовою, відмінністю мовців, впливом архітектури мережі на точність розпізнавання. Методологія включає тривале навчання та тестування, вивчення точності розпізнавання різними мовами та різні набори даних про носіїв мови. Використовуючи порівняльний аналіз, це дослідження оцінює продуктивність трьох архітектур, які навчаються виключно казахською мовою. Тестування включало висловлювання казахською та іншими мовами, у той час як кількість носіїв змінювалася, щоб оцінити його вплив на точність розпізнавання.
Під час дослідження результати показали, що нейронні мережі ЗНМ більш ефективні в розпізнаванні людського голосу, ніж РНМ і БШП. Також виявилося, що ЗНМ має більш високу точність розпізнавання людського голосу казахською мовою, як для невеликої, так і для великої кількості дикторів. Наприклад, для 20 мовців помилка розпізнавання російською склала 21,86 %, а казахською – 10,6 %. Подібна тенденція спостерігалася для 80 носіїв мови: 16,2 % росіян і 8,3 % казахів. Можна також стверджувати, що вивчення однієї мови не гарантує високої точності розпізнавання іншими мовами. Тому точність розпізнавання людського голосу нейронними мережами істотно залежить від мови, якою ведеться навчання.
Крім того, це дослідження підкреслює важливість різних наборів даних про спікерів для досягнення оптимальних результатів. Ці знання мають вирішальне значення для просування в розробці надійних систем розпізнавання людського голосу, які можуть точно ідентифікувати різні людські голоси в різних мовних контекстах
Посилання
- Mihalache, S., Burileanu, D. (2022). Using Voice Activity Detection and Deep Neural Networks with Hybrid Speech Feature Extraction for Deceptive Speech Detection. Sensors, 22 (3), 1228. https://doi.org/10.3390/s22031228
- Lee, Y., Min, J., Han, D. K., Ko, H. (2020). Spectro-Temporal Attention-Based Voice Activity Detection. IEEE Signal Processing Letters, 27, 131–135. https://doi.org/10.1109/lsp.2019.2959917
- Sofer, A., Chazan, S. E. (2022). CNN self-attention voice activity detector. arXiv. https://doi.org/10.48550/arXiv.2203.02944
- Zhang, X.-L., Xu, M. (2022). AUC optimization for deep learning-based voice activity detection. EURASIP Journal on Audio, Speech, and Music Processing, 2022 (1). https://doi.org/10.1186/s13636-022-00260-9
- Jia, F., Majumdar, S., Ginsburg, B. (2021). MarbleNet: Deep 1D Time-Channel Separable Convolutional Neural Network for Voice Activity Detection. ICASSP 2021 - 2021 IEEE International Conference on Acoustics, Speech and Signal Processing (ICASSP). https://doi.org/10.1109/icassp39728.2021.9414470
- Heo, Y., Lee, S. (2023). Supervised Contrastive Learning for Voice Activity Detection. Electronics, 12 (3), 705. https://doi.org/10.3390/electronics12030705
- Faghani, M., Rezaee-Dehsorkh, H., Ravanshad, N., Aminzadeh, H. (2023). Ultra-Low-Power Voice Activity Detection System Using Level-Crossing Sampling. Electronics, 12 (4), 795. https://doi.org/10.3390/electronics12040795
- Lee, G. W., Kim, H. K. (2020). Multi-Task Learning U-Net for Single-Channel Speech Enhancement and Mask-Based Voice Activity Detection. Applied Sciences, 10 (9), 3230. https://doi.org/10.3390/app10093230
- Arslan, O., Engin, E. Z. (2019). Noise Robust Voice Activity Detection Based on Multi-Layer Feed-Forward Neural Network. Electrica, 19 (2), 91–100. https://doi.org/10.26650/electrica.2019.18042
- Oh, Y. R., Park, K., Park, J. G. (2020). Online Speech Recognition Using Multichannel Parallel Acoustic Score Computation and Deep Neural Network (DNN)- Based Voice-Activity Detector. Applied Sciences, 10 (12), 4091. https://doi.org/10.3390/app10124091
- Sehgal, A., Kehtarnavaz, N. (2018). A Convolutional Neural Network Smartphone App for Real-Time Voice Activity Detection. IEEE Access, 6, 9017–9026. https://doi.org/10.1109/access.2018.2800728
- Mukherjee, H., Obaidullah, Sk. Md., Santosh, K. C., Phadikar, S., Roy, K. (2018). Line spectral frequency-based features and extreme learning machine for voice activity detection from audio signal. International Journal of Speech Technology, 21 (4), 753–760. https://doi.org/10.1007/s10772-018-9525-6
- Ali, Z., Talha, M. (2018). Innovative Method for Unsupervised Voice Activity Detection and Classification of Audio Segments. IEEE Access, 6, 15494–15504. https://doi.org/10.1109/access.2018.2805845
- Jung, Y., Kim, Y., Choi, Y., Kim, H. (2018). Joint Learning Using Denoising Variational Autoencoders for Voice Activity Detection. Interspeech 2018. https://doi.org/10.21437/interspeech.2018-1151
- Yoshimura, T., Hayashi, T., Takeda, K., Watanabe, S. (2020). End-to-End Automatic Speech Recognition Integrated with CTC-Based Voice Activity Detection. ICASSP 2020 - 2020 IEEE International Conference on Acoustics, Speech and Signal Processing (ICASSP). https://doi.org/10.1109/icassp40776.2020.9054358
- Bredin, H., Laurent, A. (2021). End-To-End Speaker Segmentation for Overlap-Aware Resegmentation. Interspeech 2021. https://doi.org/10.21437/interspeech.2021-560
- Lavechin, M., Gill, M.-P., Bousbib, R., Bredin, H., Garcia-Perera, L. P. (2020). End-to-End Domain-Adversarial Voice Activity Detection. Interspeech 2020. https://doi.org/10.21437/interspeech.2020-2285
- Cornell, S., Omologo, M., Squartini, S., Vincent, E. (2020). Detecting and Counting Overlapping Speakers in Distant Speech Scenarios. Interspeech 2020. https://doi.org/10.21437/interspeech.2020-2671
- Tan, X., Zhang, X.-L. (2021). Speech Enhancement Aided End-To-End Multi-Task Learning for Voice Activity Detection. ICASSP 2021 - 2021 IEEE International Conference on Acoustics, Speech and Signal Processing (ICASSP). https://doi.org/10.1109/icassp39728.2021.9414445
- Varzandeh, R., Adiloglu, K., Doclo, S., Hohmann, V. (2020). Exploiting Periodicity Features for Joint Detection and DOA Estimation of Speech Sources Using Convolutional Neural Networks. ICASSP 2020 - 2020 IEEE International Conference on Acoustics, Speech and Signal Processing (ICASSP). https://doi.org/10.1109/icassp40776.2020.9054754
- Medetov, B., Kulakayeva, A., Zhetpisbayeva, A., Albanbay, N., Kabduali, T. (2023). Identifying the regularities of the signal detection method using the Kalman filter. Eastern-European Journal of Enterprise Technologies, 5 (9 (125)), 26–34. https://doi.org/10.15587/1729-4061.2023.289472
- Mussakhojayeva, S., Khassanov, Y., Atakan Varol, H. (2022). KSC2: An Industrial-Scale Open-Source Kazakh Speech Corpus. Interspeech 2022. https://doi.org/10.21437/interspeech.2022-421
- Mussakhojayeva, S., Khassanov, Y., Atakan Varol, H. (2021). A Study of Multilingual End-to-End Speech Recognition for Kazakh, Russian, and English. Lecture Notes in Computer Science, 448–459. https://doi.org/10.1007/978-3-030-87802-3_41
- Mussakhojayeva, S., Dauletbek, K., Yeshpanov, R., Varol, H. A. (2023). Multilingual Speech Recognition for Turkic Languages. Information, 14 (2), 74. https://doi.org/10.3390/info14020074
- Musaev, M., Mussakhojayeva, S., Khujayorov, I., Khassanov, Y., Ochilov, M., Atakan Varol, H. (2021). USC: An Open-Source Uzbek Speech Corpus and Initial Speech Recognition Experiments. Lecture Notes in Computer Science, 437–447. https://doi.org/10.1007/978-3-030-87802-3_40
- Ardila, R., Branson, M., Davis, K., Henretty, M., Kohler, M., Meyer, J. et al. (2020). Common voice: A massively-multilingualspeech corpus. arXiv. https://doi.org/10.48550/arXiv.1912.06670
- Medetov, B., Serikov, T., Tolegenova, A., Zhexebay, D., Yskak, A., Namazbayev, T., Albanbay, N. (2023). Development of a model for determining the necessary FPGA computing resource for placing a multilayer neural network on it. Eastern-European Journal of Enterprise Technologies, 4 (4 (124)), 34–45. https://doi.org/10.15587/1729-4061.2023.281731
- Aigul, K., Altay, A., Yevgeniya, D., Bekbolat, M., Zhadyra, O. (2022). Improvement of Signal Reception Reliability at Satellite Spectrum Monitoring System. IEEE Access, 10, 101399–101407. https://doi.org/10.1109/access.2022.3206953
- Aitmagambetov, A., Butuzov, Y., Butuzov, Y., Tikhvinskiy, V., Tikhvinskiy, V., Kulakayeva, A. et al. (2021). Energy budget and methods for determining coordinates for a radiomonitoring system based on a small spacecraft. Indonesian Journal of Electrical Engineering and Computer Science, 21 (2), 945. https://doi.org/10.11591/ijeecs.v21.i2.pp945-956
- Albanbay, N., Medetov, B., Zaks, M. A. (2021). Exponential distribution of lifetimes for transient bursting states in coupled noisy excitable systems. Chaos: An Interdisciplinary Journal of Nonlinear Science, 31 (9). https://doi.org/10.1063/5.0059102
- Albanbay, N., Medetov, B., Zaks, M. A. (2020). Statistics of Lifetimes for Transient Bursting States in Coupled Noisy Excitable Systems. Journal of Computational and Nonlinear Dynamics, 15 (12). https://doi.org/10.1115/1.4047867
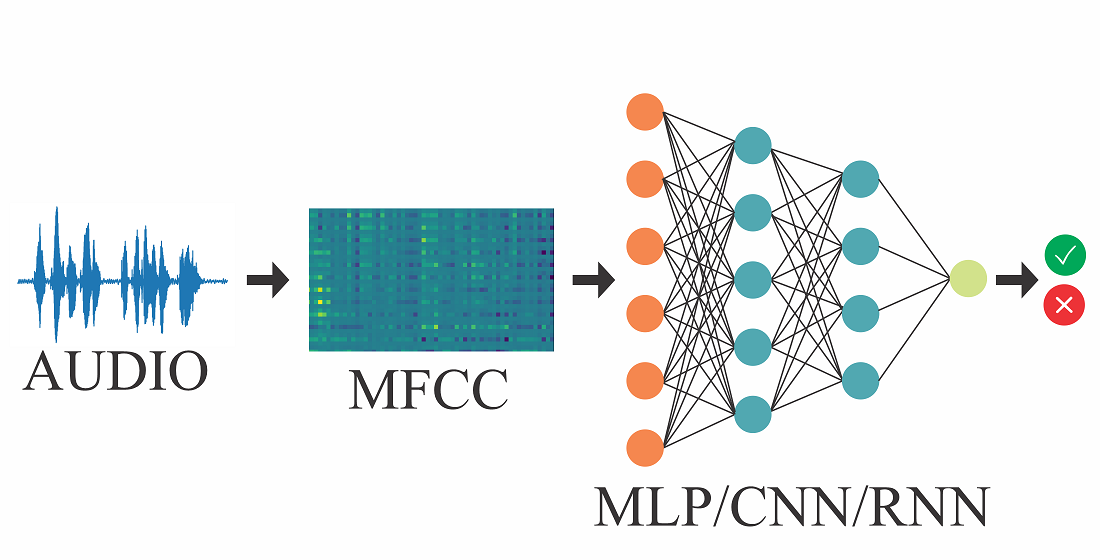
##submission.downloads##
Опубліковано
Як цитувати
Номер
Розділ
Ліцензія
Авторське право (c) 2024 Aigul Nurlankyzy, Ainur Akhmediyarova, Ainur Zhetpisbayeva, Timur Namazbayev, Asset Yskak, Nurdaulet Yerzhan, Bekbolat Medetov

Ця робота ліцензується відповідно до Creative Commons Attribution 4.0 International License.
Закріплення та умови передачі авторських прав (ідентифікація авторства) здійснюється у Ліцензійному договорі. Зокрема, автори залишають за собою право на авторство свого рукопису та передають журналу право першої публікації цієї роботи на умовах ліцензії Creative Commons CC BY. При цьому вони мають право укладати самостійно додаткові угоди, що стосуються неексклюзивного поширення роботи у тому вигляді, в якому вона була опублікована цим журналом, але за умови збереження посилання на першу публікацію статті в цьому журналі.
Ліцензійний договір – це документ, в якому автор гарантує, що володіє усіма авторськими правами на твір (рукопис, статтю, тощо).
Автори, підписуючи Ліцензійний договір з ПП «ТЕХНОЛОГІЧНИЙ ЦЕНТР», мають усі права на подальше використання свого твору за умови посилання на наше видання, в якому твір опублікований. Відповідно до умов Ліцензійного договору, Видавець ПП «ТЕХНОЛОГІЧНИЙ ЦЕНТР» не забирає ваші авторські права та отримує від авторів дозвіл на використання та розповсюдження публікації через світові наукові ресурси (власні електронні ресурси, наукометричні бази даних, репозитарії, бібліотеки тощо).
За відсутності підписаного Ліцензійного договору або за відсутністю вказаних в цьому договорі ідентифікаторів, що дають змогу ідентифікувати особу автора, редакція не має права працювати з рукописом.
Важливо пам’ятати, що існує і інший тип угоди між авторами та видавцями – коли авторські права передаються від авторів до видавця. В такому разі автори втрачають права власності на свій твір та не можуть його використовувати в будь-який спосіб.