Developing a model of association rules with machine learning in determining user habits on social media
DOI:
https://doi.org/10.15587/1729-4061.2024.305116Keywords:
habits, behavior, text data, associations, machine learning, social mediaAbstract
The object of the research is the habits of social media users. The problem in this research is that there is a lot of data spread across social media platforms ranging from image data, text data to audio data, making it difficult to identify starting from user behavior patterns, user interest in certain things and user habits. This research aims to explore scattered text data as text data has not been analyzed deeply in terms of word structure, thus concealing a lot of information and making it difficult to conduct analysis to determine user patterns and behavior on social media. The results obtained from this research are in the form of analysis and models that can identify and understand user patterns and behavior on social media using association rules and machine learning approaches. In applying the association technique, an a priori algorithm is used, which in the process determines all text data into item sets so that it can identify the habits of social media users and in the machine learning process there is a model formation process so that the results can be compared. There are several stages in the process of determining user habits, such as cleaning data, changing unstructured data to structured, then going through the rule association stage using an a priori algorithm applied to social media data so that the relationship can be seen between words. After that, machine learning is applied so that comparisons occur in seeing the relationships between words. This is new research in producing a model to identify user behavior using association rules and machine learning so that it can be used to map positive, negative or neutral user behavior
References
- El Rahman, S. A., AlOtaibi, F. A., AlShehri, W. A. (2019). Sentiment Analysis of Twitter Data. 2019 International Conference on Computer and Information Sciences (ICCIS). https://doi.org/10.1109/iccisci.2019.8716464
- Kumar, S., Khan, M. B., Hasanat, M. H. A., Saudagar, A. K. J., AlTameem, A., AlKhathami, M. (2022). An Anomaly Detection Framework for Twitter Data. Applied Sciences, 12 (21), 11059. https://doi.org/10.3390/app122111059
- Lubis, A. R., Prayudani, S., Lubis, M., Nugroho, O. (2022). Sentiment Analysis on Online Learning During the Covid-19 Pandemic Based on Opinions on Twitter using KNN Method. 2022 1st International Conference on Information System & Information Technology (ICISIT). https://doi.org/10.1109/icisit54091.2022.9872926
- Lubis, A. R., Nasution, M. K. M., Sitompul, O. S., Zamzami, E. M. (2023). A new approach to achieve the users’ habitual opportunities on social media. IAES International Journal of Artificial Intelligence (IJ-AI), 12 (1), 41. https://doi.org/10.11591/ijai.v12.i1.pp41-47
- Thakur, N. (2022). Twitter Big Data as a Resource for Exoskeleton Research: A Large-Scale Dataset of about 140,000 Tweets from 2017–2022 and 100 Research Questions. Analytics, 1 (2), 72–97. https://doi.org/10.3390/analytics1020007
- Schmidt, S., Zorenböhmer, C., Arifi, D., Resch, B. (2023). Polarity-Based Sentiment Analysis of Georeferenced Tweets Related to the 2022 Twitter Acquisition. Information, 14 (2), 71. https://doi.org/10.3390/info14020071
- Firdaniza, F., Ruchjana, B., Chaerani, D., Radianti, J. (2021). Information Diffusion Model in Twitter: A Systematic Literature Review. Information, 13 (1), 13. https://doi.org/10.3390/info13010013
- Kwon, J.-H., Kim, S., Lee, Y.-K., Ryu, K. (2021). Characteristics of Social Media Content and Their Effects on Restaurant Patrons. Sustainability, 13 (2), 907. https://doi.org/10.3390/su13020907
- Wibowo, A., Chen, S.-C., Wiangin, U., Ma, Y., Ruangkanjanases, A. (2020). Customer Behavior as an Outcome of Social Media Marketing: The Role of Social Media Marketing Activity and Customer Experience. Sustainability, 13 (1), 189. https://doi.org/10.3390/su13010189
- Ali Hakami, N., Hosni Mahmoud, H. A. (2022). The Prediction of Consumer Behavior from Social Media Activities. Behavioral Sciences, 12 (8), 284. https://doi.org/10.3390/bs12080284
- Gupta, V., Jung, K., Yoo, S.-C. (2020). Exploring the Power of Multimodal Features for Predicting the Popularity of Social Media Image in a Tourist Destination. Multimodal Technologies and Interaction, 4 (3), 64. https://doi.org/10.3390/mti4030064
- Khafaga, D. S., Alharbi, A. H., Mohamed, I., Hosny, K. M. (2022). An Integrated Classification and Association Rule Technique for Early-Stage Diabetes Risk Prediction. Healthcare, 10 (10), 2070. https://doi.org/10.3390/healthcare10102070
- Orama, J. A., Borràs, J., Moreno, A. (2021). Combining Cluster-Based Profiling Based on Social Media Features and Association Rule Mining for Personalised Recommendations of Touristic Activities. Applied Sciences, 11 (14), 6512. https://doi.org/10.3390/app11146512
- Lee, S., Cha, Y., Han, S., Hyun, C. (2019). Application of Association Rule Mining and Social Network Analysis for Understanding Causality of Construction Defects. Sustainability, 11 (3), 618. https://doi.org/10.3390/su11030618
- Kruse, R., Lokukatagoda, T., Alkhushayni, S. (2022). A framework for association rule learning with social media networks. IOP SciNotes, 3 (1), 015001. https://doi.org/10.1088/2633-1357/abe9be
- Diaz-Garcia, J. A., Ruiz, M. D., Martin-Bautista, M. J. (2022). A survey on the use of association rules mining techniques in textual social media. Artificial Intelligence Review, 56 (2), 1175–1200. https://doi.org/10.1007/s10462-022-10196-3
- Shazad, B., Ullah khan, H., Rehman, Z., Farooq, M., Mahmood, A., Mehmood, I. et al. (2019). Finding Temporal Influential Users in Social Media Using Association Rule Learning. Intelligent Automation and Soft Computing. https://doi.org/10.31209/2019.100000130
- Zhang, J., Marino, C., Canale, N., Charrier, L., Lazzeri, G., Nardone, P., Vieno, A. (2022). The Effect of Problematic Social Media Use on Happiness among Adolescents: The Mediating Role of Lifestyle Habits. International Journal of Environmental Research and Public Health, 19 (5), 2576. https://doi.org/10.3390/ijerph19052576
- Zingla, M. A., Ettaleb, M., Latiri, C. C., Slimani, Y. (2014). INEX2014: Tweet Contextualization Using Association Rules between Terms. Working Notes for CLEF 2014 Conference, 574–584. Available at: https://ceur-ws.org/Vol-1180/CLEF2014wn-Inex-ZinglaEt2014.pdf
- Koukaras, P., Tjortjis, C., Rousidis, D. (2022). Mining association rules from COVID-19 related twitter data to discover word patterns, topics and inferences. Information Systems, 109, 102054. https://doi.org/10.1016/j.is.2022.102054
- Güven, Z. A., Diri, B., Çakaloğlu, T. (2018). Classification of TurkishTweet emotions by n- stage Latent Dirichlet Allocation. 2018 Electric Electronics, Computer Science, Biomedical Engineerings’ Meeting (EBBT). https://doi.org/10.1109/ebbt.2018.8391454
- Drias, Y., Drias, H. (2021). Sentiment Evolution Analysis and Association Rule Mining for COVID-19 Tweets. Journal of Digital Art & Humanities, 2 (2), 3–21. https://doi.org/10.33847/2712-8148.2.2_1
- Lian, S., Gao, J., Li, H. (2018). A Method of Mining Association Rules for Geographical Points of Interest. ISPRS International Journal of Geo-Information, 7 (4), 146. https://doi.org/10.3390/ijgi7040146
- Kusumaningrum, R., Wiedjayanto, M. I. A., Adhy, S., Suryono (2016). Classification of Indonesian news articles based on Latent Dirichlet Allocation. 2016 International Conference on Data and Software Engineering (ICoDSE). https://doi.org/10.1109/icodse.2016.7936106
- Ranjan, R., Kumar, S. S. (2022). User behaviour analysis using data analytics and machine learning to predict malicious user versus legitimate user. High-Confidence Computing, 2 (1), 100034. https://doi.org/10.1016/j.hcc.2021.100034
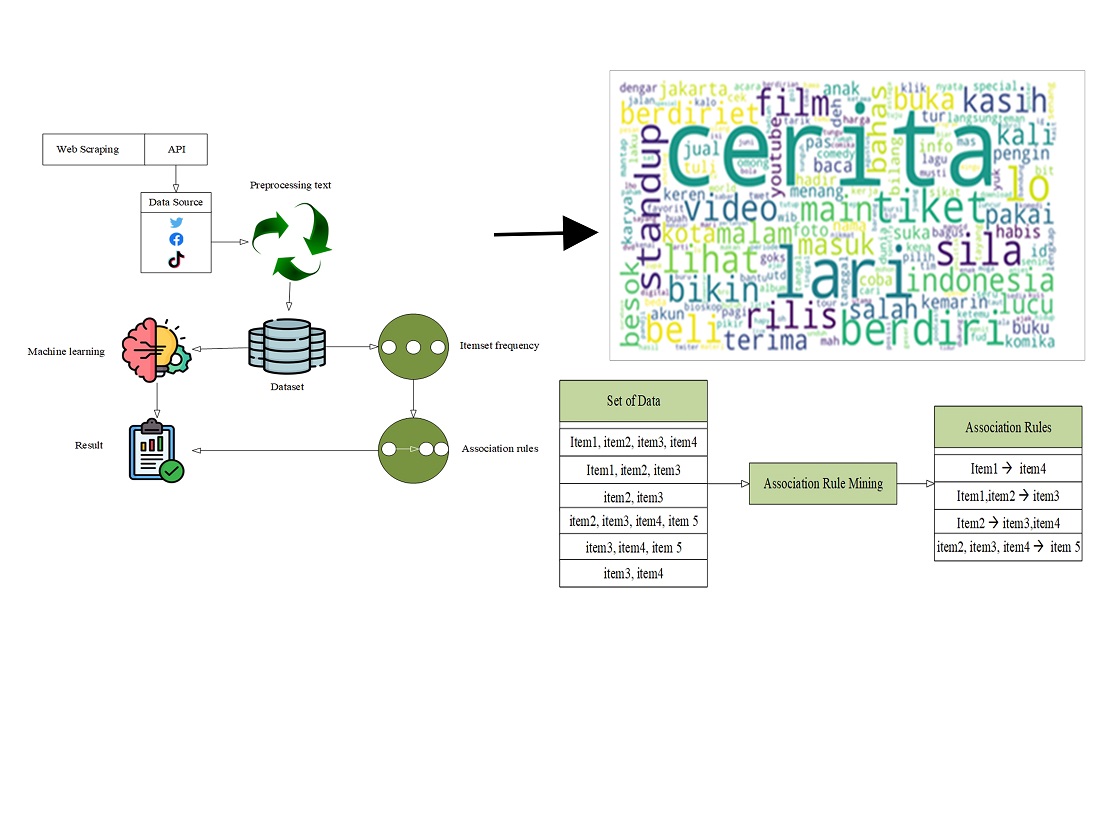
Downloads
Published
How to Cite
Issue
Section
License
Copyright (c) 2024 Antoni, Mahrani Arfah, Ferry Fachrizal, Okvi Nugroho

This work is licensed under a Creative Commons Attribution 4.0 International License.
The consolidation and conditions for the transfer of copyright (identification of authorship) is carried out in the License Agreement. In particular, the authors reserve the right to the authorship of their manuscript and transfer the first publication of this work to the journal under the terms of the Creative Commons CC BY license. At the same time, they have the right to conclude on their own additional agreements concerning the non-exclusive distribution of the work in the form in which it was published by this journal, but provided that the link to the first publication of the article in this journal is preserved.
A license agreement is a document in which the author warrants that he/she owns all copyright for the work (manuscript, article, etc.).
The authors, signing the License Agreement with TECHNOLOGY CENTER PC, have all rights to the further use of their work, provided that they link to our edition in which the work was published.
According to the terms of the License Agreement, the Publisher TECHNOLOGY CENTER PC does not take away your copyrights and receives permission from the authors to use and dissemination of the publication through the world's scientific resources (own electronic resources, scientometric databases, repositories, libraries, etc.).
In the absence of a signed License Agreement or in the absence of this agreement of identifiers allowing to identify the identity of the author, the editors have no right to work with the manuscript.
It is important to remember that there is another type of agreement between authors and publishers – when copyright is transferred from the authors to the publisher. In this case, the authors lose ownership of their work and may not use it in any way.