Statistical processing of a small sample of raw data using artificial orthogonalisation technology
DOI:
https://doi.org/10.15587/1729-4061.2023.282130Keywords:
statistical data processing, small sample, artificial orthogonalization, triaxial assignment problemAbstract
This paper addresses the task to devise a statistical estimation procedure in an event where the volume of the array of initial data used in processing is insufficient to correctly determine the parameters of the response function. The object of research is the technology of statistical processing of a small sample of data. The subject of the study is the methods of statistical estimation under conditions of a small sample of initial data. The main direction is to devise a special procedure for statistical processing of a small sample of initial data, which provides a correct statistical estimation of the parameters of the response function. The method for solving the problem is the selection of the most representative orthogonal replica-like subplan from the plan of a complete factorial experiment obtained by artificially orthogonalizing the results of a passive experiment. The necessity and expediency of the proposed procedure is a consequence of the unpredictability and uneven distribution of points in the phase space of coordinates. The result of the implementation of the corresponding procedure is a truncated orthogonal plan of the full factorial experiment, which provides the possibility of independent estimation of all coefficients of the regression polynomial describing the response function. Under conditions of a severe shortage of the number of measurements, the procedure makes it possible to isolate a representative orthogonal replica from the resulting plan of a complete factorial experiment. Using this subplan of the full factorial experiment plan makes it possible to evaluate all the coefficients of the regression polynomial that describes the desired response function. The corresponding computational procedure is based on solving the triaxial Boolean assignment problem
References
- Shoba, K. (2019). Multiple diskriminant analysis. Arlington: Inst. for statistics Education, 112.
- Aouati, M. (2017). Improvement of accuracy of parametric classification in the space of N×2 factors-attributes on the basis of preliminary obtained linear discriminant function. EUREKA: Physics and Engineering, 3, 55–68. doi: https://doi.org/10.21303/2461-4262.2017.00362
- Luna-Romera, J. M., Martínez-Ballesteros, M., García-Gutiérrez, J., Riquelme, J. C. (2019). External clustering validity index based on chi-squared statistical test. Information Sciences, 487, 1–17. doi: https://doi.org/10.1016/j.ins.2019.02.046
- Everitt, B. S., Landau, S., Leese, M., Stahl, D. (2011). Cluster Analysis. Wiley. doi: https://doi.org/10.1002/9780470977811
- Aouati, M. (2018). Improving the accuracy of classifying rules for controlling the processes of deculfuration and dephosphorization of Fe-C melt. Technology Audit and Production Reserves, 2 (3 (46)), 10–18. doi: https://doi.org/10.15587/2312-8372.2019.169696
- Mourad, A. (2017). Parametric identification in the problem of determining the quality of dusulfusation and deposphoration processes of Fe-C alloy. Technology Audit and Production Reserves, 2 (1 (34)), 9–15. doi: https://doi.org/10.15587/2312-8372.2017.99130
- Uotermen, D. (1989). Rukovodstvo po ekspertnym sistemam. Moscow: Mir, 388.
- Dzhekson, P. (2014). Vvedenie v ekspertnye sistemy. Moscow: Vil'yams, 624.
- Dzharratano, D., Rayli, G. (2016). Ekspertnye sistemy. Moscow: Vil'yams, 1152.
- Gavrilova, T. A., Khoroshevskiy, V. F. (2011). Bazy znaniy intellektual'nykh sistem. Sankt-Peterburg: Piter, 384.
- Oimoen, S. (2019). Classical Designs: Full Factorial Designs. STAT Center of Excellence, 26. Available at: https://www.afit.edu/stat/statcoe_files/Classical%20Designs-Full%20Factorial%20Designs_Final.pdf
- Montgomery, D. (2013). Design and analysis of experiments. Wiley.
- Burman, L. E., Reed, W. R., Alm, J. (2010). A Call for Replication Studies. Public Finance Review, 38 (6), 787–793. doi: https://doi.org/10.1177/1091142110385210
- Hari, R. (2022). Replication study.
- Narayan, C., Das, M. (2009). Design and analysis of Experiments. New of Experiments. Wiley, 53–76.
- Kullback, S. (1959). Information Theory and statistics. Willey.
- Seraya, O. V., Demin, D. A. (2012). Lineynyy regressionnyy analiz maloy vyborki nechetkikh iskhodnykh dannykh. Problemy upravleniya i informatiki, 4, 129–142.
- Domin, D., Sira, O., Raskin, L. (2021). Artificial orthogonalization of a passive experiment for a small sample of fuzzy data for constructing regression equations. Available at: https://ingraph.org/en/products/212
- Raskin, L. G. (1976). Analiz slozhnykh sistem i elementy teorii optimal'nogo upravleniya. Moscow: Sov. Radio, 344.
- Domin, D. (2013). Artificial orthogonalization in searching of optimal control of technological processes under uncertainty conditions. Eastern-European Journal of Enterprise Technologies, 5 (9(65)), 45–53. doi: https://doi.org/10.15587/1729-4061.2013.18452
- Adler, Yu. P., Markova, E. V., Granovskiy, Yu. V. (1971). Planirovanie eksperimenta pri poiske optimal'nykh usloviy. Moscow: Nauka, 282.
- Domin, D., Sira, O., Raskin, L. (2021). Technology for constructing regression equations for a small sample of passive experiment data. Available at: https://ingraph.org/en/products/211
- Raskin, L. G. (1982). Mnogoindeksnye zadachi lineynogo programmirovaniya. Moscow: Radio i svyaz', 246.
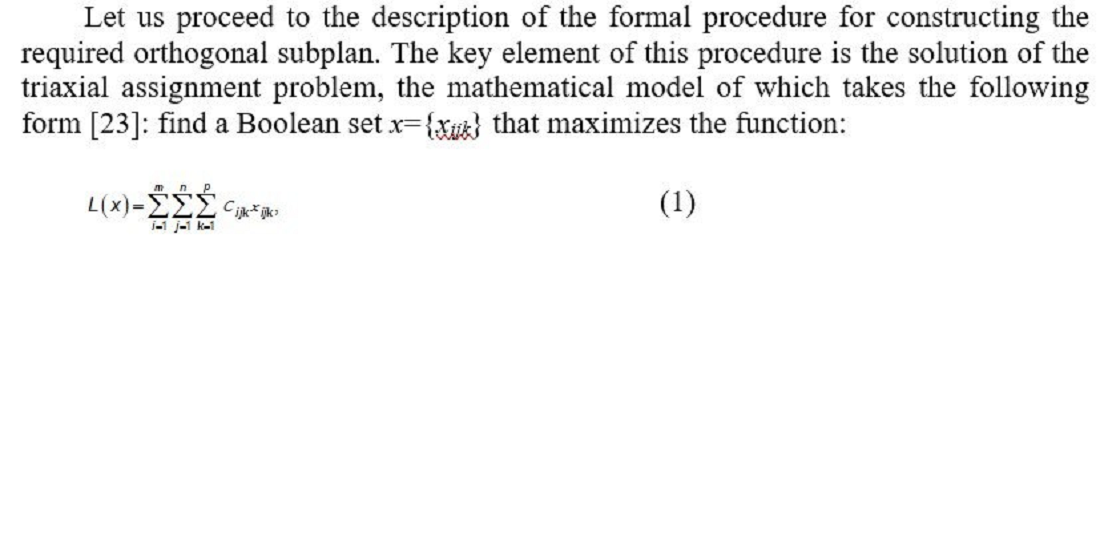
Downloads
Published
How to Cite
Issue
Section
License
Copyright (c) 2023 Lev Raskin, Larysa Sukhomlyn, Viacheslav Karpenko, Dmytro Sokolov

This work is licensed under a Creative Commons Attribution 4.0 International License.
The consolidation and conditions for the transfer of copyright (identification of authorship) is carried out in the License Agreement. In particular, the authors reserve the right to the authorship of their manuscript and transfer the first publication of this work to the journal under the terms of the Creative Commons CC BY license. At the same time, they have the right to conclude on their own additional agreements concerning the non-exclusive distribution of the work in the form in which it was published by this journal, but provided that the link to the first publication of the article in this journal is preserved.
A license agreement is a document in which the author warrants that he/she owns all copyright for the work (manuscript, article, etc.).
The authors, signing the License Agreement with TECHNOLOGY CENTER PC, have all rights to the further use of their work, provided that they link to our edition in which the work was published.
According to the terms of the License Agreement, the Publisher TECHNOLOGY CENTER PC does not take away your copyrights and receives permission from the authors to use and dissemination of the publication through the world's scientific resources (own electronic resources, scientometric databases, repositories, libraries, etc.).
In the absence of a signed License Agreement or in the absence of this agreement of identifiers allowing to identify the identity of the author, the editors have no right to work with the manuscript.
It is important to remember that there is another type of agreement between authors and publishers – when copyright is transferred from the authors to the publisher. In this case, the authors lose ownership of their work and may not use it in any way.