Comparison evaluation of unet-based models with noise augmentation for breast cancer segmentation on ultrasound image
DOI:
https://doi.org/10.15587/1729-4061.2023.289044Keywords:
breast cancer, ultrasound imaging, deep learning, UNet, image segmentation, augmentationAbstract
This paper investigates the application of convolutional neural networks (CNNs), particularly the UNet model architecture, to improve the accuracy of breast cancer tumor segmentation in ultrasound images. Accurate identification of breast cancer is essential for effective patient treatment. However, ultrasound images, often contain noise and artifacts, which can complicate the task of tumor segmentation. Therefore, to highlight the most robust architecture, modifications were made to the original set, including the addition of noise and fuzziness. In this study, a comparative study of five different variants of UNet models (UNet, Attention UNet, UNet++, Dense Inception UNet and Residual UNet) was conducted on a diverse set of ultrasound images with different breast tumors. Using consistent training methods and techniques of augmentation and adding noise to the data, an improvement in segmentation accuracy was highlighted when using the Dense Inception UNet architecture. The results have potential practical applications in the field of medical diagnosis and can assist medical professionals in automatic tumor segmentation in breast cancer ultrasound images. This study highlights the improvement of segmentation accuracy by introducing dense induction into the UNet architecture. Importantly, the Dice coefficient, a key segmentation metric, improved markedly, increasing from 0.973 to 0.976 after data augmentation. The results of the study offer promise to the medical community by offering a more accurate and reliable approach to segmenting breast cancer lesions on ultrasound images. The findings can be implemented in clinical practice to assist radiologists in early cancer diagnosis.
References
- World Health Organization. Available at: https://www.who.int/
- Dunenova, G. A., Kalmataeva, Z. A., Kaidarova, D. R., Shatkovskaya, O. V., Zhylkaidarova, A. Z., Marchenko, E. A.,Glushkova, N. E. (2023). Breast cancer epidemiology in Kazakhstan for the period 2012-2021. Science & Healthcare, 25 (2). doi: https://doi.org/10.34689/SH.2023.25.2.018
- Igissinov, N., Toguzbayeva, A., Turdaliyeva, B., Igissinova, G., Bilyalova, Z., Akpolatova, G. et al. (2020). Breast Cancer in Megapolises of Kazakhstan: Epidemiological Assessment of Incidence and Mortality. Iranian Journal of Public Health. doi: https://doi.org/10.18502/ijph.v48i7.2948
- Barba, D., León-Sosa, A., Lugo, P., Suquillo, D., Torres, F., Surre, F. et al. (2021). Breast cancer, screening and diagnostic tools: All you need to know. Critical Reviews in Oncology/Hematology, 157, 103174. doi: https://doi.org/10.1016/j.critrevonc.2020.103174
- Ginsburg, O., Yip, C., Brooks, A., Cabanes, A., Caleffi, M., Dunstan Yataco, J. A. et al. (2020). Breast cancer early detection: A phased approach to implementation. Cancer, 126 (S10), 2379–2393. doi: https://doi.org/10.1002/cncr.32887
- Esteva, A., Chou, K., Yeung, S., Naik, N., Madani, A., Mottaghi, A. et al. (2021). Deep learning-enabled medical computer vision. Npj Digital Medicine, 4 (1). doi: https://doi.org/10.1038/s41746-020-00376-2
- Dhahri, H., Al Maghayreh, E., Mahmood, A., Elkilani, W., Faisal Nagi, M. (2019). Automated Breast Cancer Diagnosis Based on Machine Learning Algorithms. Journal of Healthcare Engineering, 2019, 1–11. doi: https://doi.org/10.1155/2019/4253641
- MI-STA 2022 Conference Proceeding. (2022). 2022 IEEE 2nd International Maghreb Meeting of the Conference on Sciences and Techniques of Automatic Control and Computer Engineering (MI-STA). doi: https://doi.org/10.1109/mi-sta54861.2022.9837515
- Kuttan, G. O., Elayidom, M. S. (2023). Review on Computer Aided Breast Cancer Detection and Diagnosis using Machine Learning Methods on Mammogram Image. Current Medical Imaging Formerly Current Medical Imaging Reviews, 19 (12). doi: https://doi.org/10.2174/1573405619666230213093639
- Liu, Z., Wang, S., Dong, D., Wei, J., Fang, C., Zhou, X. et al. (2019). The Applications of Radiomics in Precision Diagnosis and Treatment of Oncology: Opportunities and Challenges. Theranostics, 9 (5), 1303–1322. doi: https://doi.org/10.7150/thno.30309
- Ronneberger, O., Fischer, P., Brox, T. (2015). U-Net: Convolutional Networks for Biomedical Image Segmentation. Medical Image Computing and Computer-Assisted Intervention – MICCAI 2015, 234–241. doi: https://doi.org/10.1007/978-3-319-24574-4_28
- Zhou, Z., Rahman Siddiquee, M. M., Tajbakhsh, N., Liang, J. (2018). UNet++: A Nested U-Net Architecture for Medical Image Segmentation. Lecture Notes in Computer Science, 3–11. doi: https://doi.org/10.1007/978-3-030-00889-5_1
- Oktay, O., Schlemper, J., Folgoc, L. L., Lee, M., Heinrich, M., Misawa, K. et al. (2018). Attention u-net: Learning where to look for the pancreas. arXiv. doi: https://doi.org/10.48550/arXiv.1804.03999
- Zhang, Z., Liu, Q., Wang, Y. (2018). Road Extraction by Deep Residual U-Net. IEEE Geoscience and Remote Sensing Letters, 15 (5), 749–753. doi: https://doi.org/10.1109/lgrs.2018.2802944
- Targ, S., Almeida, D., Lyman, K. (2016). Resnet in resnet: Generalizing residual architectures. arXiv. doi: https://doi.org/10.48550/arXiv.1603.08029
- Zhang, Z., Wu, C., Coleman, S., Kerr, D. (2020). DENSE-INception U-net for medical image segmentation. Computer Methods and Programs in Biomedicine, 192, 105395. doi: https://doi.org/10.1016/j.cmpb.2020.105395
- Zhao, Y., Lai, Z., Shen, L., Kong, H. (2022). Breast Lesions Segmentation using Dual-level UNet (DL-UNet). 2022 IEEE 35th International Symposium on Computer-Based Medical Systems (CBMS). doi: https://doi.org/10.1109/cbms55023.2022.00067
- Honghan, Z., Liu, D. C., Jingyan, L., Liu, P., Yin, H., Peng, Y. (2021). RMS-SE-UNet: A Segmentation Method for Tumors in Breast Ultrasound Images. 2021 IEEE 6th International Conference on Computer and Communication Systems (ICCCS). doi: https://doi.org/10.1109/icccs52626.2021.9449302
- Negi, A., Raj, A. N. J., Nersisson, R., Zhuang, Z., Murugappan, M. (2020). RDA-UNET-WGAN: An Accurate Breast Ultrasound Lesion Segmentation Using Wasserstein Generative Adversarial Networks. Arabian Journal for Science and Engineering, 45 (8), 6399–6410. doi: https://doi.org/10.1007/s13369-020-04480-z
- Chavan, T., Prajapati, K., JV, K. R. (2022). InvUNET: Involuted UNET for Breast Tumor Segmentation from Ultrasound. Lecture Notes in Computer Science, 283–290. doi: https://doi.org/10.1007/978-3-031-09342-5_27
- Chen, G., Li, L., Dai, Y., Zhang, J., Yap, M. H. (2023). AAU-Net: An Adaptive Attention U-Net for Breast Lesions Segmentation in Ultrasound Images. IEEE Transactions on Medical Imaging, 42 (5), 1289–1300. doi: https://doi.org/10.1109/tmi.2022.3226268
- Agarap, A. F. (2018). Deep Learning using Rectified Linear Units (ReLU). arXiv. doi: https://doi.org/10.48550/arXiv.1803.08375
- Liang, X., Wang, X., Lei, Z., Liao, S., Li, S. Z. (2017). Soft-Margin Softmax for Deep Classification. Lecture Notes in Computer Science, 413–421. doi: https://doi.org/10.1007/978-3-319-70096-0_43
- Guerrero-Pena, F. A., Marrero Fernandez, P. D., Ing Ren, T., Yui, M., Rothenberg, E., Cunha, A. (2018). Multiclass Weighted Loss for Instance Segmentation of Cluttered Cells. 2018 25th IEEE International Conference on Image Processing (ICIP). doi: https://doi.org/10.1109/icip.2018.8451187
- Schlemper, J., Oktay, O., Schaap, M., Heinrich, M., Kainz, B., Glocker, B., Rueckert, D. (2019). Attention gated networks: Learning to leverage salient regions in medical images. Medical Image Analysis, 53, 197–207. doi: https://doi.org/10.1016/j.media.2019.01.012
- Khanna, A., Londhe, N. D., Gupta, S., Semwal, A. (2020). A deep Residual U-Net convolutional neural network for automated lung segmentation in computed tomography images. Biocybernetics and Biomedical Engineering, 40 (3), 1314–1327. doi: https://doi.org/10.1016/j.bbe.2020.07.007
- He, K., Zhang, X., Ren, S., Sun, J. (2016). Deep Residual Learning for Image Recognition. 2016 IEEE Conference on Computer Vision and Pattern Recognition (CVPR). doi: https://doi.org/10.1109/cvpr.2016.90
- Chicco, D., Jurman, G. (2020). The advantages of the Matthews correlation coefficient (MCC) over F1 score and accuracy in binary classification evaluation. BMC Genomics, 21 (1). doi: https://doi.org/10.1186/s12864-019-6413-7
- Shamir, R. R., Duchin, Y., Kim, J., Sapiro, G., Harel, N. (2018). Continuous Dice Coefficient: a Method for Evaluating Probabilistic Segmentations. doi: https://doi.org/10.1101/306977
- Derczynski, L. (2016). Complementarity, F-score, and NLP Evaluation. Proceedings of the Tenth International Conference on Language Resources and Evaluation (LREC'16). Portorož, 261–266. Available at: https://aclanthology.org/L16-1040/
- Eelbode, T., Bertels, J., Berman, M., Vandermeulen, D., Maes, F., Bisschops, R., Blaschko, M. B. (2020). Optimization for Medical Image Segmentation: Theory and Practice When Evaluating With Dice Score or Jaccard Index. IEEE Transactions on Medical Imaging, 39 (11), 3679–3690. doi: https://doi.org/10.1109/tmi.2020.3002417
- Cheng, B., Girshick, R., Dollar, P., Berg, A. C., Kirillov, A. (2021). Boundary IoU: Improving Object-Centric Image Segmentation Evaluation. 2021 IEEE/CVF Conference on Computer Vision and Pattern Recognition (CVPR). doi: https://doi.org/10.1109/cvpr46437.2021.01508
- Al-Dhabyani, W., Gomaa, M., Khaled, H., Fahmy, A. (2020). Dataset of breast ultrasound images. Data in Brief, 28, 104863. doi: https://doi.org/10.1016/j.dib.2019.104863
- Al-Dhabyani, W., Gomaa, M., Khaled, H., Fahmy, A. (2019). Deep Learning Approaches for Data Augmentation and Classification of Breast Masses using Ultrasound Images. International Journal of Advanced Computer Science and Applications, 10 (5). doi: https://doi.org/10.14569/ijacsa.2019.0100579
- Akkus, Z., Cai, J., Boonrod, A., Zeinoddini, A., Weston, A. D., Philbrick, K. A., Erickson, B. J. (2019). A Survey of Deep-Learning Applications in Ultrasound: Artificial Intelligence–Powered Ultrasound for Improving Clinical Workflow. Journal of the American College of Radiology, 16 (9), 1318–1328. doi: https://doi.org/10.1016/j.jacr.2019.06.004
- Ilesanmi, A. E., Chaumrattanakul, U., Makhanov, S. S. (2021). A method for segmentation of tumors in breast ultrasound images using the variant enhanced deep learning. Biocybernetics and Biomedical Engineering, 41 (2), 802–818. doi: https://doi.org/10.1016/j.bbe.2021.05.007
- Almajalid, R., Shan, J., Du, Y., Zhang, M. (2018). Development of a Deep-Learning-Based Method for Breast Ultrasound Image Segmentation. 2018 17th IEEE International Conference on Machine Learning and Applications (ICMLA). doi: https://doi.org/10.1109/icmla.2018.00179
- Zhang, C., Bao, N., Sun, H., Li, H., Li, J., Qian, W., Zhou, S. (2022). A Deep Learning Image Data Augmentation Method for Single Tumor Segmentation. Frontiers in Oncology, 12. doi: https://doi.org/10.3389/fonc.2022.782988
- Ferreira, M. R., Torres, H. R., Oliveira, B., Gomes-Fonseca, J., Morais, P., Novais, P., Vilaca, J. L. (2022). Comparative Analysis of Current Deep Learning Networks for Breast Lesion Segmentation in Ultrasound Images. 2022 44th Annual International Conference of the IEEE Engineering in Medicine & Biology Society (EMBC). doi: https://doi.org/10.1109/embc48229.2022.9871091
- Mukasheva, A., Akanov, Z., Yedilkhan, D. (2021). Research of the Regression Analysis Methods for Predicting the Growth of Patients with Diabetes Mellitus. 2021 IEEE International Conference on Smart Information Systems and Technologies (SIST). doi: https://doi.org/10.1109/sist50301.2021.9465975
- Zhao, T., Dai, H. (2022). Breast Tumor Ultrasound Image Segmentation Method Based on Improved Residual U-Net Network. Computational Intelligence and Neuroscience, 2022, 1–9. doi: https://doi.org/10.1155/2022/3905998
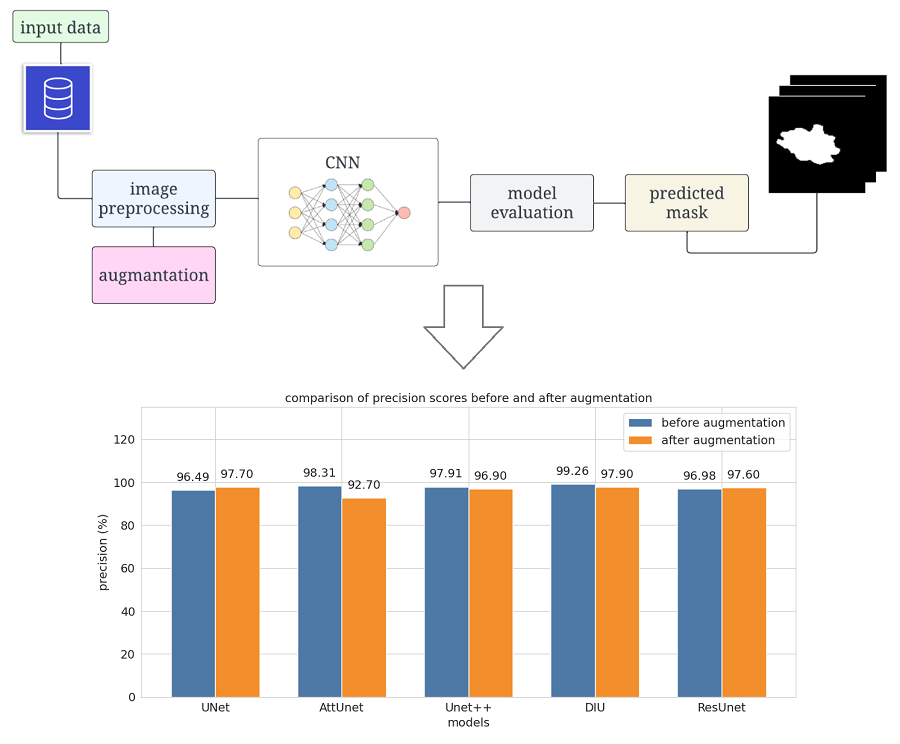
Downloads
Published
How to Cite
Issue
Section
License
Copyright (c) 2023 Assel Mukasheva, Dina Koishiyeva, Zhanna Suimenbayeva, Sabina Rakhmetulayeva, Aigerim Bolshibayeva, Gulnar Sadikova

This work is licensed under a Creative Commons Attribution 4.0 International License.
The consolidation and conditions for the transfer of copyright (identification of authorship) is carried out in the License Agreement. In particular, the authors reserve the right to the authorship of their manuscript and transfer the first publication of this work to the journal under the terms of the Creative Commons CC BY license. At the same time, they have the right to conclude on their own additional agreements concerning the non-exclusive distribution of the work in the form in which it was published by this journal, but provided that the link to the first publication of the article in this journal is preserved.
A license agreement is a document in which the author warrants that he/she owns all copyright for the work (manuscript, article, etc.).
The authors, signing the License Agreement with TECHNOLOGY CENTER PC, have all rights to the further use of their work, provided that they link to our edition in which the work was published.
According to the terms of the License Agreement, the Publisher TECHNOLOGY CENTER PC does not take away your copyrights and receives permission from the authors to use and dissemination of the publication through the world's scientific resources (own electronic resources, scientometric databases, repositories, libraries, etc.).
In the absence of a signed License Agreement or in the absence of this agreement of identifiers allowing to identify the identity of the author, the editors have no right to work with the manuscript.
It is important to remember that there is another type of agreement between authors and publishers – when copyright is transferred from the authors to the publisher. In this case, the authors lose ownership of their work and may not use it in any way.