Features of the coefficient of variation of parameters of the gas environment in fire in the premises
DOI:
https://doi.org/10.15587/1729-4061.2023.293279Keywords:
ignition of material, gas environment, dangerous parameters, measure of sample coefficient of variationAbstract
The object of the study is the selective coefficient of variation of dangerous parameters of the gas environment during the ignition of materials. The measure of the sample coefficient of variation of an arbitrary hazardous parameter of the gas environment observed at an arbitrary time interval is substantiated. The representativeness error of the measure of the sampling coefficient of variation, which depends on the value of the measure and the sample size, was determined. The measure allows you to numerically determine its value for an arbitrary observation interval. The difference in the measure at the intervals corresponding to the reliable absence and occurrence of ignition allows to detect the occurrence of ignition of the material. According to the results of laboratory studies, the measures of the sample coefficient of variation for carbon monoxide concentration, smoke density, and temperature of the gas medium in the laboratory chamber at intervals of absence and appearance of ignition of alcohol, paper, wood, and textiles were determined. It was established that the dangerous parameters of the gas environment at the intervals of absence and presence of ignition are characterized by different values of the increase in the measure of the sample coefficient of variation. For example, it is determined that the ignition of alcohol causes the maximum increase in the measure for carbon monoxide concentration from 0.135 to 0.441, for smoke density from 0.629 to 0.805, and for temperature from 0.001 to 0.115. When paper catches fire, the measure for carbon monoxide concentration and temperature increases from 0.0026 to 0.140 and from 0.0019 to 0.05, respectively. When burning wood, the measure for carbon monoxide concentration and temperature increases from 0.0072 to 0.177 and from 0.0067 to 0.016, respectively. The obtained results, provided that the hazardous parameters of the gas environment in the premises are measured and the sample coefficient of variation is calculated in practice, make it possible to use them in the creation of early fire detection systems
References
- Iatsyshyn, A. V., Ivaschenko, T. G., Matvieieva, I. V., Zakharchenko, J. V., Lahoiko, A. M. (2023). Development of recommendations for improving the radiation monitoring system of Ukraine. IOP Conference Series: Earth and Environmental Science, 1254 (1), 012109. doi: https://doi.org/10.1088/1755-1315/1254/1/012109
- Barannik, V., Babenko, Y., Kulitsa, O., Barannik, V., Khimenko, A., Matviichuk-Yudina, O. (2020). Significant Microsegment Transformants Encoding Method to Increase the Availability of Video Information Resource. 2020 IEEE 2nd International Conference on Advanced Trends in Information Theory (ATIT). doi: https://doi.org/10.1109/atit50783.2020.9349256
- Sadkovyi, V., Andronov, V., Semkiv, O., Kovalov, A., Rybka, E., Otrosh, Yu. et al.; Sadkovyi, V., Rybka, E., Otrosh, Yu. (Eds.) (2021). Fire resistance of reinforced concrete and steel structures. Kharkiv: РС ТЕСHNOLOGY СЕNTЕR, 180. doi: https://doi.org/10.15587/978-617-7319-43-5
- Otrosh, Y., Rybka, Y., Danilin, O., Zhuravskyi, M. (2019). Assessment of the technical state and the possibility of its control for the further safe operation of building structures of mining facilities. E3S Web of Conferences, 123, 01012. doi: https://doi.org/10.1051/e3sconf/201912301012
- Pospelov, B., Kovrehin, V., Rybka, E., Krainiukov, O., Petukhova, O., Butenko, T. et al. (2020). Development of a method for detecting dangerous states of polluted atmospheric air based on the current recurrence of the combined risk. Eastern-European Journal of Enterprise Technologies, 5 (9 (107)), 49–56. doi: https://doi.org/10.15587/1729-4061.2020.213892
- Center of Fire Statistics (2022). World Fire Statistics of CTIF, 27.
- Chernukha, A., Teslenko, A., Kovalov, P., Bezuglov, O. (2020). Mathematical Modeling of Fire-Proof Efficiency of Coatings Based on Silicate Composition. Materials Science Forum, 1006, 70–75. doi: https://doi.org/10.4028/www.scientific.net/msf.1006.70
- Pospelov, B., Rybka, E., Meleshchenko, R., Krainiukov, O., Biryukov, I., Butenko, T. et al. (2021). Short-term fire forecast based on air state gain recurrence and zero-order brown model. Eastern-European Journal of Enterprise Technologies, 3 (10 (111)), 27–33. doi: https://doi.org/10.15587/1729-4061.2021.233606
- Pospelov, B., Rybka, E., Krainiukov, O., Yashchenko, O., Bezuhla, Y., Bielai, S. et al. (2021). Short-term forecast of fire in the premises based on modification of the Brown’s zero-order model. Eastern-European Journal of Enterprise Technologies, 4 (10 (112)), 52–58. doi: https://doi.org/10.15587/1729-4061.2021.238555
- Cheng, C., Sun, F., Zhou, X. (2011). One fire detection method using neural networks. Tsinghua Science and Technology, 16 (1), 31–35. doi: https://doi.org/10.1016/s1007-0214(11)70005-0
- Ding, Q., Peng, Z., Liu, T., Tong, Q. (2014). Multi-Sensor Building Fire Alarm System with Information Fusion Technology Based on D-S Evidence Theory. Algorithms, 7 (4), 523–537. doi: https://doi.org/10.3390/a7040523
- Wu, Y., Harada, T. (2004). Study on the Burning Behaviour of Plantation Wood. Scientia Silvae Sinicae, 40 (2), 131–136. doi: https://doi.org/10.11707/j.1001-7488.20040223
- Ji, J., Yang, L., Fan, W. (2003). Experimental Study on Effects of Burning Behaviours of Materials Caused by External Heat Radiation. Journal of Combustion Science and Technology, 9, 139.
- Peng, X., Liu, S., Lu, G. (2005). Experimental Analysis on Heat Release Rate of Materials. Journal of Chongqing University, 28, 122.
- Heskestad, G., Newman, J. S. (1992). Fire detection using cross-correlations of sensor signals. Fire Safety Journal, 18 (4), 355–374. doi: https://doi.org/10.1016/0379-7112(92)90024-7
- Gottuk, D. T., Wright, M. T., Wong, J. T., Pham, H. V., Rose-Pehrsson, S. L., Hart, S. et al. (2002). Prototype Early Warning Fire Detection Systems: Test Series 4 Results. NRL/MR/6180-02-8602. Naval Research Laboratory.
- Pospelov, B., Rybka, E., Togobytska, V., Meleshchenko, R., Danchenko, Y., Butenko, T. et al. (2019). Construction of the method for semi-adaptive threshold scaling transformation when computing recurrent plots. Eastern-European Journal of Enterprise Technologies, 4 (10 (100)), 22–29. doi: https://doi.org/10.15587/1729-4061.2019.176579
- Pospelov, B., Andronov, V., Rybka, E., Krainiukov, O., Karpets, K., Pirohov, O. et al. (2019). Development of the correlation method for operative detection of recurrent states. Eastern-European Journal of Enterprise Technologies, 6 (4 (102)), 39–46. doi: https://doi.org/10.15587/1729-4061.2019.187252
- Sadkovyi, V., Pospelov, B., Andronov, V., Rybka, E., Krainiukov, O., Rud, A. et al. (2020). Construction of a method for detecting arbitrary hazard pollutants in the atmospheric air based on the structural function of the current pollutant concentrations. Eastern-European Journal of Enterprise Technologies, 6 (10 (108)), 14–22. doi: https://doi.org/10.15587/1729-4061.2020.218714
- Pospelov, B., Rybka, E., Meleshchenko, R., Krainiukov, O., Harbuz, S., Bezuhla, Y. et al. (2020). Use of uncertainty function for identification of hazardous states of atmospheric pollution vector. Eastern-European Journal of Enterprise Technologies, 2 (10 (104)), 6–12. doi: https://doi.org/10.15587/1729-4061.2020.200140
- Pospelov, B., Rybka, E., Savchenko, A., Dashkovska, O., Harbuz, S., Naden, E. et al. (2022). Peculiarities of amplitude spectra of the third order for the early detection of indoor fires. Eastern-European Journal of Enterprise Technologies, 5 (10 (119)), 49–56. doi: https://doi.org/10.15587/1729-4061.2022.265781
- Pospelov, B., Andronov, V., Rybka, E., Chubko, L., Bezuhla, Y., Gordiichuk, S. et al. (2023). Revealing the peculiarities of average bicoherence of frequencies in the spectra of dangerous parameters of the gas environment during fire. Eastern-European Journal of Enterprise Technologies, 1 (10 (121)), 46–54. doi: https://doi.org/10.15587/1729-4061.2023.272949
- Du, L., Liu, H., Bao, Z. (2005). Radar HRRP target recognition based on higher order spectra. IEEE Transactions on Signal Processing, 53 (7), 2359–2368. doi: https://doi.org/10.1109/tsp.2005.849161
- Hayashi, K., Mukai, N., Sawa, T. (2014). Simultaneous bicoherence analysis of occipital and frontal electroencephalograms in awake and anesthetized subjects. Clinical Neurophysiology, 125 (1), 194–201. doi: https://doi.org/10.1016/j.clinph.2013.06.024
- Pospelov, B., Rybka, E., Polkovnychenko, D., Myskovets, I., Bezuhla, Y., Butenko, T. et al. (2023). Comparison of bicoherence on the ensemble of realizations and a selective evaluation of the bispectrum of the dynamics of dangerous parameters of the gas medium during fire. Eastern-European Journal of Enterprise Technologies, 2 (10 (122)), 14–21. doi: https://doi.org/10.15587/1729-4061.2023.276779
- Forkman, J. (2006). Statistical inference for the coefficient of variation in normally distributed data. Centre of biostatistics. Report 2.
- Curto, J. D., Pinto, J. C. (2008). The coefficient of variation asymptotic distribution in the case of non-iid random variables. Journal of Applied Statistics, 36 (1), 21–32. doi: https://doi.org/10.1080/02664760802382491
- Abdi, H., Edelman, B., Valentin, D., Dowling, W. J. (2009). Experimental design and analysis for psychology. Oxford University Press. Available at: https://citeseerx.ist.psu.edu/document?repid=rep1&type=pdf&doi=e340ab80345010a0c6e458ebb3e1458345b0b489
- Ye, L., Gu, X., Wang, D., Vogel, R. M. (2021). An unbiased estimator of coefficient of variation of streamflow. Journal of Hydrology, 594, 125954. doi: https://doi.org/10.1016/j.jhydrol.2021.125954
- Yousef, A. (2020). Performance of Three-Stage Sequential Estimation of the Normal Inverse Coefficient of Variation Under Type II Error Probability: A Monte Carlo Simulation Study. Frontiers in Physics, 8. doi: https://doi.org/10.3389/fphy.2020.00071
- Polstiankin, R. M., Pospelov, B. B. (2015). Stochastic models of hazardous factors and parameters of a fire in the premises. Problemy pozharnoy bezopasnosti, 38, 130–135. Available at: http://nbuv.gov.ua/UJRN/Ppb_2015_38_24
- Pospelov, B., Andronov, V., Rybka, E., Skliarov, S. (2017). Research into dynamics of setting the threshold and a probability of ignition detection by selfadjusting fire detectors. Eastern-European Journal of Enterprise Technologies, 5 (9 (89)), 43–48. doi: https://doi.org/10.15587/1729-4061.2017.110092
- Dubinin, D., Cherkashyn, O., Maksymov, A., Beliuchenko, D., Hovalenkov, S., Shevchenko, S., Avetisyan, V. (2020). Investigation of the effect of carbon monoxide on people in case of fire in a building. Sigurnost, 62 (4), 347–357. doi: https://doi.org/10.31306/s.62.4.2
- Pasport. Spovishchuvach pozhezhnyi teplovyi tochkovyi. TPT-4. Arton. Available at: https://ua.arton.com.ua/files/passports/%D0%A2%D0%9F%D0%A2-4_UA.pdf
- Pasport. Spovishchuvach pozhezhnyi dymovyi tochkovyi optychnyi. SPD-3.2. Arton. Available at: https://ua.arton.com.ua/files/passports/spd-32_new_pas_ua.pdf
- Optical/Heat Multisensor Detector. Discovery. Available at: https://www.nsc-hellas.gr/pdf/APOLLO/discovery/B02704-00%20Discovery%20Multisensor%20Heat-%20Optical.pdf
- McGrattan K., Hostikka S., McDermott R., Floyd J., Weinschenk C., Overholt K. (2016). Fire dynamics simulator technical reference guide. Volume 3: Validation. National Institute of Standards and Technology. Available at: https://www.fse-italia.eu/PDF/ManualiFDS/FDS_Validation_Guide.pdf
- McGrattan, K., Hostikka, S., McDermott, R., Floyd, J., Weinschenk, C., Overholt, K. (2013). Fire Dynamics Simulator User’s Guide. National Institute of Standard and Technology. Available at: https://tsapps.nist.gov/publication/get_pdf.cfm?pub_id=913619
- Levin, B. R. (1989). Teoreticheskie osnovy statisticheskoy radiotehniki. Moscow: Radio i svyaz', 656.
- Gorban', I. I. (2011). Osobennosti zakona bol'shih chisel pri narusheniyah statisticheskoy ustoychivosti. Visti vyshchykh uchbovykh zakladiv. Radioelektronika, 54 (7), 31–42.
- Orlov, Yu. N., Osminin, K. P. (2008). Postroenie vyborochnoy funktsii raspredeleniya dlya prognozirovaniya nestatsionarnogo vremennogo ryada. Matematicheskoe modelirovanie, 20 (9), 23–33.
- Dragotti, P. L., Vetterli, M., Blu, T. (2007). Sampling Moments and Reconstructing Signals of Finite Rate of Innovation: Shannon Meets Strang–Fix. IEEE Transactions on Signal Processing, 55 (5), 1741–1757. doi: https://doi.org/10.1109/tsp.2006.890907
- Forkman, J. (2009). Estimator and Tests for Common Coefficients of Variation in Normal Distributions. Communications in Statistics - Theory and Methods, 38 (2), 233–251. doi: https://doi.org/10.1080/03610920802187448
- Pospelov, B., Andronov, V., Rybka, E., Samoilov, M., Krainiukov, O., Biryukov, I. et al. (2021). Development of the method of operational forecasting of fire in the premises of objects under real conditions. Eastern-European Journal of Enterprise Technologies, 2 (10 (110)), 43–50. doi: https://doi.org/10.15587/1729-4061.2021.226692
- Pospelov, B., Andronov, V., Rybka, E., Popov, V., Semkiv, O. (2018). Development of the method of frequencytemporal representation of fluctuations of gaseous medium parameters at fire. Eastern-European Journal of Enterprise Technologies, 2 (10 (92)), 44–49. doi: https://doi.org/10.15587/1729-4061.2018.125926
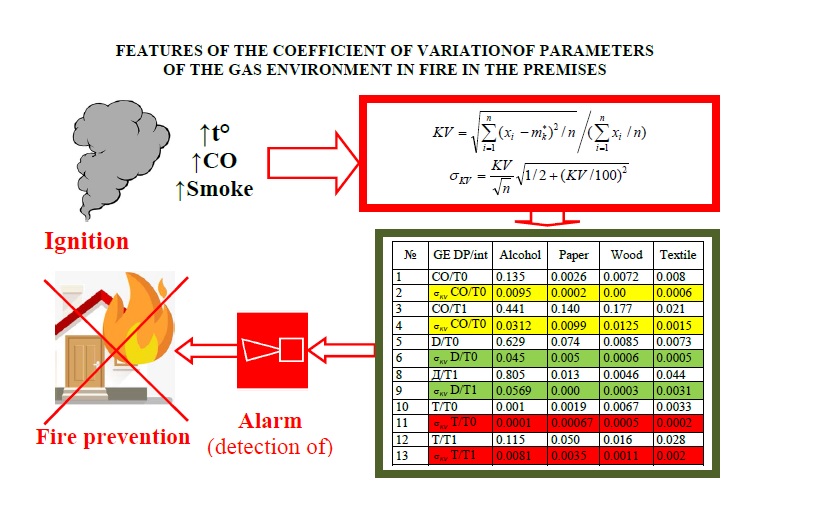
Downloads
Published
How to Cite
Issue
Section
License
Copyright (c) 2023 Boris Pospelov, Yuliia Bezuhla, Yurii Kozar, Olekcii Krainiukov, Larysa Chubko, Oleksandr Yashchenko, Olena Liashevska, Sergey Shcherbak, Pavlo Cherevko, Viacheslav Kurepin

This work is licensed under a Creative Commons Attribution 4.0 International License.
The consolidation and conditions for the transfer of copyright (identification of authorship) is carried out in the License Agreement. In particular, the authors reserve the right to the authorship of their manuscript and transfer the first publication of this work to the journal under the terms of the Creative Commons CC BY license. At the same time, they have the right to conclude on their own additional agreements concerning the non-exclusive distribution of the work in the form in which it was published by this journal, but provided that the link to the first publication of the article in this journal is preserved.
A license agreement is a document in which the author warrants that he/she owns all copyright for the work (manuscript, article, etc.).
The authors, signing the License Agreement with TECHNOLOGY CENTER PC, have all rights to the further use of their work, provided that they link to our edition in which the work was published.
According to the terms of the License Agreement, the Publisher TECHNOLOGY CENTER PC does not take away your copyrights and receives permission from the authors to use and dissemination of the publication through the world's scientific resources (own electronic resources, scientometric databases, repositories, libraries, etc.).
In the absence of a signed License Agreement or in the absence of this agreement of identifiers allowing to identify the identity of the author, the editors have no right to work with the manuscript.
It is important to remember that there is another type of agreement between authors and publishers – when copyright is transferred from the authors to the publisher. In this case, the authors lose ownership of their work and may not use it in any way.