Improving the technology for constructing a software tool to determine the similarity of raster graphic images
DOI:
https://doi.org/10.15587/1729-4061.2024.298744Keywords:
raster graphics, image similarity, software, linguistic variables, hash code, algorithmAbstract
The object of research is the process of searching and analyzing images of raster graphics. In the context of this work, the problem of the lack of an effective and fast procedure for determining the similarity of images was solved.
The technology for improving the construction of a software tool for determining the similarity of raster graphics images by devising a procedure for determining the similarity of images using a hash code that corresponds to all variants of the image, regardless of size and aspect ratio, is reported. The image features of raster graphics were systematized.
A procedure for determining the similarity of images using a hash code is proposed. This procedure makes it possible to process all possible variants of the image, regardless of the size and aspect ratio. The resulting indicator of the proposed procedure is the value of the hash codes.
It is proposed to use the mathematical apparatus of fuzzy logic by introducing linguistic variables to estimate the similarity index. A comparison of the numerical values of similarity, obtained on the basis of the use of information systems, and the linguistic values revealed in the survey process was carried out. Threshold values were obtained that make it possible to assess the degree of similarity of the images.
Based on the proposed algorithm, a prototype of the information system for determining the similarity of images of raster graphics has been designed. As a result of the calculation of the numerical characteristics of the improvement of the technology of constructing a software tool for determining the similarity of images of raster graphics, the value of the precision indicators was approximately 0.89 and the completeness was 0.8. The advantage of the proposed technology for determining the similarity of images over known analog technologies is illustrated by the amount of RAM of the developed software, which is 210 MB
References
- Sun, W.-T., Lin, Y.-J. (2020). Systematic Review and Discussion on Final Artwork of Prepress Design. Education and Awareness of Sustainability. https://doi.org/10.1142/9789811228001_0175
- Cho, S., Byun, H. (2012). Dynamic curve color model for image matting. Pattern Recognition Letters, 33 (7), 920–933. https://doi.org/10.1016/j.patrec.2011.04.012
- Gu, C., Lu, X., Zhang, C. (2022). Example-based color transfer with Gaussian mixture modeling. Pattern Recognition, 129, 108716. https://doi.org/10.1016/j.patcog.2022.108716
- Cahyadi, T., Susanto, A., Riyono, D. (2021). Control of packaging print quality with an integrated production flow system in prepress. KREATOR, 2 (1). https://doi.org/10.46961/kreator.v2i1.283
- Al’boschiy, O., Dorokhov, O., Hrabovskyi, Y., Naumenko, M. (2022). Automated balancing method of vector Illustration and its software implementation. Bulletin of the Transilvania University of Brasov. Series III: Mathematics and Computer Science, 177–192. https://doi.org/10.31926/but.mif.2022.2.64.1.12
- Buehring, J., Bishop, P. C. (2020). Foresight and Design: New Support for Strategic Decision Making. She Ji: The Journal of Design, Economics, and Innovation, 6 (3), 408–432. https://doi.org/10.1016/j.sheji.2020.07.002
- Roudný, P., Držková, M. (2020). Use of prepress automation in the Czech Republic and examples of automated processing for selected prepress tasks. Proceedings - The Tenth International Symposium GRID 2020. https://doi.org/10.24867/grid-2020-p72
- Yuan, L., Wang, T., Zhang, X., Tay, F. E., Jie, Z., Liu, W., Feng, J. (2020). Central Similarity Quantization for Efficient Image and Video Retrieval. 2020 IEEE/CVF Conference on Computer Vision and Pattern Recognition (CVPR). https://doi.org/10.1109/cvpr42600.2020.00315
- Hrabovskyi, Y., Kots, H., Veretelnyk, K. (2023). Development of a methodology for creating an on-line layout assistant for electronic publications for mobile devices. EUREKA: Physics and Engineering, 5, 164–176. https://doi.org/10.21303/2461-4262.2023.003105
- Vallez, C., Kucharavy, A., Dolamic, L. (2022). Needle in a Haystack, Fast: Benchmarking Image Perceptual Similarity Metrics At Scale. arXiv. https://doi.org/10.48550/arXiv.2206.00282
- Hrabovskyi, Y. (2023). How to Enhance the Management and Quality of Electronic Publications? Materials Research Proceedings. https://doi.org/10.21741/9781644902691-50
- A fragment of the Python program code of a prototype information system for determining the similarity of images. Available at: https://drive.google.com/file/d/17C1gE5CwJipYZ3rzr3thx1dGYKfbgVOB/view
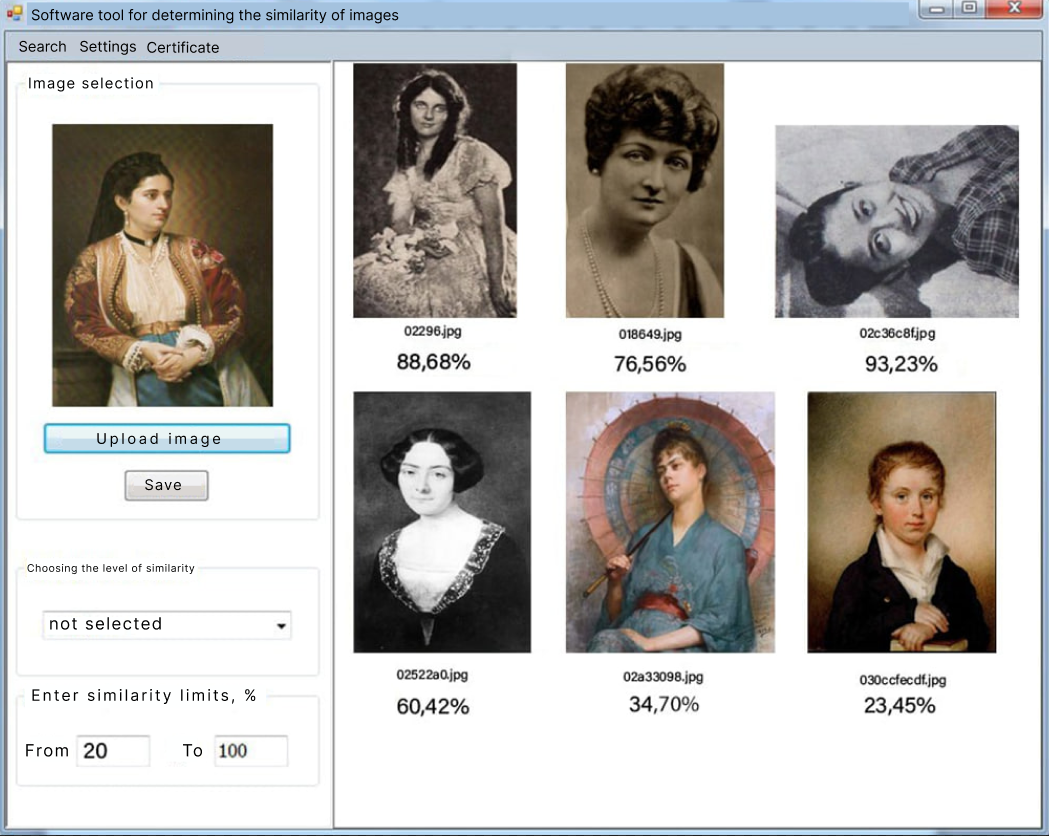
Downloads
Published
How to Cite
Issue
Section
License
Copyright (c) 2024 Yevhen Hrabovskyi, Dmytro Bondarenko, Igor Kobzev

This work is licensed under a Creative Commons Attribution 4.0 International License.
The consolidation and conditions for the transfer of copyright (identification of authorship) is carried out in the License Agreement. In particular, the authors reserve the right to the authorship of their manuscript and transfer the first publication of this work to the journal under the terms of the Creative Commons CC BY license. At the same time, they have the right to conclude on their own additional agreements concerning the non-exclusive distribution of the work in the form in which it was published by this journal, but provided that the link to the first publication of the article in this journal is preserved.
A license agreement is a document in which the author warrants that he/she owns all copyright for the work (manuscript, article, etc.).
The authors, signing the License Agreement with TECHNOLOGY CENTER PC, have all rights to the further use of their work, provided that they link to our edition in which the work was published.
According to the terms of the License Agreement, the Publisher TECHNOLOGY CENTER PC does not take away your copyrights and receives permission from the authors to use and dissemination of the publication through the world's scientific resources (own electronic resources, scientometric databases, repositories, libraries, etc.).
In the absence of a signed License Agreement or in the absence of this agreement of identifiers allowing to identify the identity of the author, the editors have no right to work with the manuscript.
It is important to remember that there is another type of agreement between authors and publishers – when copyright is transferred from the authors to the publisher. In this case, the authors lose ownership of their work and may not use it in any way.