Розробка моделі використання "розумного будинку" іт-фахівцями: роль машинного навчання
DOI:
https://doi.org/10.15587/1729-4061.2022.265657Ключові слова:
РСАДПЗ, Інтернет речей, відносна перевага, розумний будинок, модель прийняття технологійАнотація
Моделі використання "розумного будинку" переважають у розвинених країнах, тоді як в країнах, що розвиваються вони все ще відсутні. У контексті "розумного будинку" широко використовується модель прийняття технологій (МПТ), інші теорії прийняття технологій маловивчені. Метою даного дослідження є вивчення досвіду використання "розумного будинку" фахівцями з інформаційних технологій (ІТ) в Раді співробітництва арабських держав Перської затоки (РСАДПЗ). У роботі використовуються теорії існування і показано позитивний вплив відносної переваги, зручності, доступності та вартості на намір використовувати "розумний будинок". Крім того, висловлено припущення, що намір використовувати, а також сприятливі умови безпосередньо впливають на фактичне використання "розумного будинку". В якості посередника між наміром використовувати і фактичним використанням були запропоновані знання з машинного навчання. Дані були зібрані у ІТ-фахівців РСАДПЗ за допомогою цілеспрямованої вибірки. Аналіз проводився методом аналізу моментних структур (АМОС). Результати показали, що зручність, доступність та відносна перевага мають позитивний вплив, тоді як вартість негативно впливає на намір використовувати "розумний будинок". Намір використовувати і сприятливі умови позитивно вплинули на фактичне використання. Знання в області машинного навчання мали позитивний вплив на намір використовувати і фактичне використання. Особам, що приймають рішення, рекомендується розширити переваги використання "розумного будинку" на основі Інтернету речей та створити індивідуальний план, що дозволяє використовувати "розумний будинок" на всіх рівнях. Знання в області машинного навчання мають велике значення для використання "розумного будинку". В цьому відношенні, у розширенні використання "розумного будинку" важливу роль відіграють спеціалізовані курси.
Посилання
- Marikyan, D., Papagiannidis, S., Alamanos, E. (2019). A systematic review of the smart home literature: A user perspective. Technological Forecasting and Social Change, 138, 139–154. doi: https://doi.org/10.1016/j.techfore.2018.08.015
- Sovacool, B. K., Furszyfer Del Rio, D. D. (2020). Smart home technologies in Europe: A critical review of concepts, benefits, risks and policies. Renewable and Sustainable Energy Reviews, 120, 109663. doi: https://doi.org/10.1016/j.rser.2019.109663
- Almusaylim, Z. A., Zaman, N. (2018). A review on smart home present state and challenges: linked to context-awareness internet of things (IoT). Wireless Networks, 25 (6), 3193–3204. doi: https://doi.org/10.1007/s11276-018-1712-5
- Awad, S. R., Sharef, B. T., Salih, A. M., Malallah, F. L. (2022). Deep learning-based iraqi banknotes classification system for blind people. Eastern-European Journal of Enterprise Technologies, 1 (2 (115)), 31–38. doi: https://doi.org/10.15587/1729-4061.2022.248642
- Liang, T., Zeng, B., Liu, J., Ye, L., Zou, C. (2018). An Unsupervised User Behavior Prediction Algorithm Based on Machine Learning and Neural Network For Smart Home. IEEE Access, 6, 49237–49247. doi: https://doi.org/10.1109/access.2018.2868984
- Hargreaves, T., Wilson, C., Hauxwell-Baldwin, R. (2017). Learning to live in a smart home. Building Research & Information, 46 (1), 127–139. doi: https://doi.org/10.1080/09613218.2017.1286882
- Maleki, M., Manshouri, N., Kayikcioglu, T. (2021). Brain-computer Interface Systems for Smart Homes - A Review Study. Recent Advances in Electrical & Electronic Engineering (Formerly Recent Patents on Electrical & Electronic Engineering), 14 (2), 144–155. doi: https://doi.org/10.2174/2352096513999200727175948
- Miandashti, F. J., Izadi, M., Shirehjini, A. A. N., Shirmohammadi, S. (2020). An Empirical Approach to Modeling User-System Interaction Conflicts in Smart Homes. IEEE Transactions on Human-Machine Systems, 50 (6), 573–583. doi: https://doi.org/10.1109/thms.2020.3017784
- Ousghir, S., Daoud, M. (2022). Exploratory study on innovation management in startups, an attempt to design it through the business model. Eastern-European Journal of Enterprise Technologies, 1 (13 (115)), 20–26. doi: https://doi.org/10.15587/1729-4061.2022.251845
- Marufuzzaman, M., Tumbraegel, T., Rahman, L. F., Sidek, L. M. (2021). A machine learning approach to predict the activity of smart home inhabitant. Journal of Ambient Intelligence and Smart Environments, 13 (4), 271–283. doi: https://doi.org/10.3233/ais-210604
- Machorro-Cano, I., Alor-Hernández, G., Paredes-Valverde, M. A., Rodríguez-Mazahua, L., Sánchez-Cervantes, J. L., Olmedo-Aguirre, J. O. (2020). HEMS-IoT: A Big Data and Machine Learning-Based Smart Home System for Energy Saving. Energies, 13 (5), 1097. doi: https://doi.org/10.3390/en13051097
- Babichenko, A., Kravchenko, Y., Babichenko, J., Lysachenko, I., Krasnikov, I., Velma, V. (2022). Design of an intelligent system to control the technological system of ammonia production secondary condensation. Eastern-European Journal of Enterprise Technologies, 1 (2 (115)), 105–115. doi: https://doi.org/10.15587/1729-4061.2022.252383
- Khudov, H., Makoveichuk, O., Misiuk, D., Pievtsov, H., Khizhnyak, I., Solomonenko, Y. et. al. (2022). Devising a method for processing the image of a vehicle’s license plate when shooting with a smartphone camera. Eastern-European Journal of Enterprise Technologies, 1 (2 (115)), 6–21. doi: https://doi.org/10.15587/1729-4061.2022.252310
- Galchonkov, O., Nevrev, A., Shevchuk, B., Baranov, N. (2022). Determination of the influence of the choice of the pruning procedure parameters on the learning quality of a multilayer perceptron. Eastern-European Journal of Enterprise Technologies, 1 (9 (115)), 75–83. doi: https://doi.org/10.15587/1729-4061.2022.253103
- Dong, X., Chang, Y., Wang, Y., Yan, J. (2017). Understanding usage of Internet of Things (IOT) systems in China. Information Technology & People, 30 (1), 117–138. doi: https://doi.org/10.1108/itp-11-2015-0272
- Park, E., Cho, Y., Han, J., Kwon, S. J. (2017). Comprehensive Approaches to User Acceptance of Internet of Things in a Smart Home Environment. IEEE Internet of Things Journal, 4 (6), 2342–2350. doi: https://doi.org/10.1109/jiot.2017.2750765
- Mital, M., Chang, V., Choudhary, P., Papa, A., Pani, A. K. (2018). Adoption of Internet of Things in India: A test of competing models using a structured equation modeling approach. Technological Forecasting and Social Change, 136, 339–346. doi: https://doi.org/10.1016/j.techfore.2017.03.001
- de Boer, P. S., van Deursen, A. J. A. M., van Rompay, T. J. L. (2019). Accepting the Internet-of-Things in our homes: The role of user skills. Telematics and Informatics, 36, 147–156. doi: https://doi.org/10.1016/j.tele.2018.12.004
- Nakashydze, L., Gil’orme, T. (2015). Energy security assessment when introducing renewable energy technologies. Eastern-European Journal of Enterprise Technologies, 4 (8 (76)), 54–59. doi: https://doi.org/10.15587/1729-4061.2015.46577
- Pronoza, P., Kuzenko, T., Sablina, N. (2022). Implementation of strategic tools in the process of financial security management of industrial enterprises in Ukraine. Eastern-European Journal of Enterprise Technologies, 2 (13 (116)), 15–23. doi: https://doi.org/10.15587/1729-4061.2022.254234
- Poltorak, A., Khrystenko, O., Sukhorukova, A., Moroz, T., Sharin, O. (2022). Development of an integrated approach to assessing the impact of innovative development on the level of financial security of households. Eastern-European Journal of Enterprise Technologies, 1 (13 (115)), 103–112. doi: https://doi.org/10.15587/1729-4061.2022.253062
- Shachak, A., Kuziemsky, C., Petersen, C. (2019). Beyond TAM and UTAUT: Future directions for HIT implementation research. Journal of Biomedical Informatics, 100, 103315. doi: https://doi.org/10.1016/j.jbi.2019.103315
- Hong, A., Nam, C., Kim, S. (2020). What will be the possible barriers to consumers’ adoption of smart home services? Telecommunications Policy, 44 (2), 101867. doi: https://doi.org/10.1016/j.telpol.2019.101867
- Arthanat, S., Chang, H., Wilcox, J. (2020). Determinants of information communication and smart home automation technology adoption for aging-in-place. Journal of Enabling Technologies, 14 (2), 73–86. doi: https://doi.org/10.1108/jet-11-2019-0050
- Balta-Ozkan, N., Davidson, R., Bicket, M., Whitmarsh, L. (2013). Social barriers to the adoption of smart homes. Energy Policy, 63, 363–374. doi: https://doi.org/10.1016/j.enpol.2013.08.043
- Pal, D., Funilkul, S., Charoenkitkarn, N., Kanthamanon, P. (2018). Internet-of-Things and Smart Homes for Elderly Healthcare: An End User Perspective. IEEE Access, 6, 10483–10496. doi: https://doi.org/10.1109/access.2018.2808472
- Cvitić, I., Peraković, D., Periša, M., Gupta, B. (2021). Ensemble machine learning approach for classification of IoT devices in smart home. International Journal of Machine Learning and Cybernetics, 12 (11), 3179–3202. doi: https://doi.org/10.1007/s13042-020-01241-0
- Lytvyn, V., Vysotska, V., Demchuk, A., Demkiv, I., Ukhanska, O., Hladun, V. et. al. (2019). Design of the architecture of an intelligent system for distributing commercial content in the internet space based on SEO-technologies, neural networks, and Machine Learning. Eastern-European Journal of Enterprise Technologies, 2 (2 (98)), 15–34. doi: https://doi.org/10.15587/1729-4061.2019.164441
- Tsoy, A., Titlov, O., Granovskiy, A., Koretskiy, D., Vorobyova, O., Tsoy, D., Jamasheva, R. (2022). Improvement of refrigerating machine energy efficiency through radiative removal of condensation heat. Eastern-European Journal of Enterprise Technologies, 1 (8 (115)), 35–45. doi: https://doi.org/10.15587/1729-4061.2022.251834
- Huang, H., Yu, H. (2019). Compact and Fast Machine Learning Accelerator for IoT Devices. Springer, 149. doi: https://doi.org/10.1007/978-981-13-3323-1
- Aliiev, E., Paliy, A., Kis, V., Paliy, A., Petrov, R., Plyuta, L. et. al. (2022). Establishing the influence of technical and technological parameters of milking equipment on the efficiency of machine milking. Eastern-European Journal of Enterprise Technologies, 1 (1 (115)), 44–55. doi: https://doi.org/10.15587/1729-4061.2022.251172
- Nykyforov, А., Antoshchenkov, R., Halych, I., Kis, V., Polyansky, P., Koshulko, V. et. al. (2022). Construction of a regression model for assessing the efficiency of separation of lightweight seeds on vibratory machines involving measures to reduce the harmful influence of the aerodynamic factor. Eastern-European Journal of Enterprise Technologies, 2 (1 (116)), 24–34. doi: https://doi.org/10.15587/1729-4061.2022.253657
- Zahorulko, A., Zagorulko, A., Kasabova, K., Liashenko, B., Postadzhiev, A., Sashnova, M. (2022). Improving a tempering machine for confectionery masses. Eastern-European Journal of Enterprise Technologies, 2 (11 (116)), 6–11. doi: https://doi.org/10.15587/1729-4061.2022.254873
- Petrakov, Y., Korenkov, V., Myhovych, A. (2022). Technology for programming contour milling on a CNC machine. Eastern-European Journal of Enterprise Technologies, 2 (1 (116)), 55–61. doi: https://doi.org/10.15587/1729-4061.2022.255389
- Alarifi, A., Tolba, A. (2019). Optimizing the network energy of cloud assisted internet of things by using the adaptive neural learning approach in wireless sensor networks. Computers in Industry, 106, 133–141. doi: https://doi.org/10.1016/j.compind.2019.01.004
- Davis, F. D. (1989). Perceived Usefulness, Perceived Ease of Use, and User Acceptance of Information Technology. MIS Quarterly, 13 (3), 319. doi: https://doi.org/10.2307/249008
- Venkatesh, V., Morris, M. G., Davis, G. B., Davis, F. D. (2003). User Acceptance of Information Technology: Toward a Unified View. MIS Quarterly, 27 (3), 425. doi: https://doi.org/10.2307/30036540
- Shin, D.-H., Jin Park, Y. (2017). Understanding the Internet of Things ecosystem: multi-level analysis of users, society, and ecology. Digital Policy, Regulation and Governance, 19 (1), 77–100. doi: https://doi.org/10.1108/dprg-07-2016-0035
- Khayer, A., Talukder, Md. S., Bao, Y., Hossain, Md. N. (2020). Cloud computing adoption and its impact on SMEs’ performance for cloud supported operations: A dual-stage analytical approach. Technology in Society, 60, 101225. doi: https://doi.org/10.1016/j.techsoc.2019.101225
- Sivathanu, B. (2018). Adoption of internet of things (IOT) based wearables for healthcare of older adults – a behavioural reasoning theory (BRT) approach. Journal of Enabling Technologies, 12 (4), 169–185. doi: https://doi.org/10.1108/jet-12-2017-0048
- Pinochet, L. H. C., Lopes, E. L., Srulzon, C. H. F., Onusic, L. M. (2018). The influence of the attributes of “Internet of Things” products on functional and emotional experiences of purchase intention. Innovation & Management Review, 15 (3), 303–320. doi: https://doi.org/10.1108/inmr-05-2018-0028
- de Oliveira, G. A. A., de Bettio, R. W., Freire, A. P. (2016). Accessibility of the smart home for users with visual disabilities: an evaluation of open source mobile applications for home automation. Proceedings of the 15th Brazilian Symposium on Human Factors in Computing Systems. doi: https://doi.org/10.1145/3033701.3033730
- Agustina, R., Suprianto, D., Ariyanto, R. (2021). Technology Acceptance Model Analysis of User Behavioral Intentions on IoT Smart Board Devices. 2021 1st Conference on Online Teaching for Mobile Education (OT4ME). doi: https://doi.org/10.1109/ot4me53559.2021.9638937
- Sivarethinamohan, R., Sujatha, S. (2021). Upskilling and Curating the Potentials of IoT Enabled Smart Cities: Use Cases and Implementation Strategies. Artificial Intelligence in Industrial Applications, 67–78. doi: https://doi.org/10.1007/978-3-030-85383-9_5
- Al-Momani, A. M., Mahmoud, M. A., Ahmad, M. S. (2018). Factors that Influence the Acceptance of Internet of Things Services by Customers of Telecommunication Companies in Jordan. Journal of Organizational and End User Computing, 30 (4), 51–63. doi: https://doi.org/10.4018/joeuc.2018100104
- Karahoca, A., Karahoca, D., Aksöz, M. (2017). Examining intention to adopt to internet of things in healthcare technology products. Kybernetes, 47 (4), 742–770. doi: https://doi.org/10.1108/k-02-2017-0045
- Shuhaiber, A., Mashal, I. (2019). Understanding users’ acceptance of smart homes. Technology in Society, 58, 101110. doi: https://doi.org/10.1016/j.techsoc.2019.01.003
- Solangi, Z. A., Solangi, Y. A., Aziz, M. S. Abd., Asadullah. (2017). An empirical study of Internet of Things (IoT) – Based healthcare acceptance in Pakistan: PILOT study. 2017 IEEE 3rd International Conference on Engineering Technologies and Social Sciences (ICETSS). doi: https://doi.org/10.1109/icetss.2017.8324135
- Sohn, K., Kwon, O. (2020). Technology acceptance theories and factors influencing artificial Intelligence-based intelligent products. Telematics and Informatics, 47, 101324. doi: https://doi.org/10.1016/j.tele.2019.101324
- Chakraborty, S., Khayer, N., Ahmed, T. (2020). Assessing Critical Factors Affecting the Mass Adoption of IoT in Bangladesh. International Conference on Mechanical, Industrial and Energy Engineering. Available at: https://www.researchgate.net/publication/347889988_Assessing_Critical_Factors_Affecting_the_Mass_Adoption_of_IoT_in_Bangladesh
- Roma, P., Monaro, M., Muzi, L., Colasanti, M., Ricci, E., Biondi, S. et. al. (2020). How to Improve Compliance with Protective Health Measures during the COVID-19 Outbreak: Testing a Moderated Mediation Model and Machine Learning Algorithms. International Journal of Environmental Research and Public Health, 17 (19), 7252. doi: https://doi.org/10.3390/ijerph17197252
- Hwang, J., Park, S., Kim, I. (2020). Understanding motivated consumer innovativeness in the context of a robotic restaurant: The moderating role of product knowledge. Journal of Hospitality and Tourism Management, 44, 272–282. doi: https://doi.org/10.1016/j.jhtm.2020.06.003
- Rogers, E. (1995). Diffusion of innovations. Available at: https://web.stanford.edu/class/symbsys205/Diffusion%20of%20Innovations.htm
- Kayali, M., Alaaraj, S. (2020). Adoption of Cloud Based E-learning in Developing Countries: A Combination of DOI, TAM and UTAUT. International Journal of Contemporary Management and Information Technology, 1 (1), 1–7. Available at: https://ijcmit.com/wp-content/uploads/2020/11/Kayali-Alaaraj-2020-1d59ab79.pdf
- Lian, J.-W. (2015). Critical factors for cloud based e-invoice service adoption in Taiwan: An empirical study. International Journal of Information Management, 35 (1), 98–109. doi: https://doi.org/10.1016/j.ijinfomgt.2014.10.005
- Hair, J. F., Anderson, R. E., Babin, B. J., Black, W. C. (2010). Multivariate data analysis: A global perspective. Upper Saddle River (N.J.) : Pearson education, 800.
- Lowry, P. B., Gaskin, J. (2014). Partial Least Squares (PLS) Structural Equation Modeling (SEM) for Building and Testing Behavioral Causal Theory: When to Choose It and How to Use It. IEEE Transactions on Professional Communication, 57 (2), 123–146. doi: https://doi.org/10.1109/tpc.2014.2312452
- Awang, Z. (2014). A Handbook on Structural Equation Modeling for Academicians and Practitioner. Bandar Baru Bangi, kuala lumpur, Malaysia: MPWS Rich Resources.
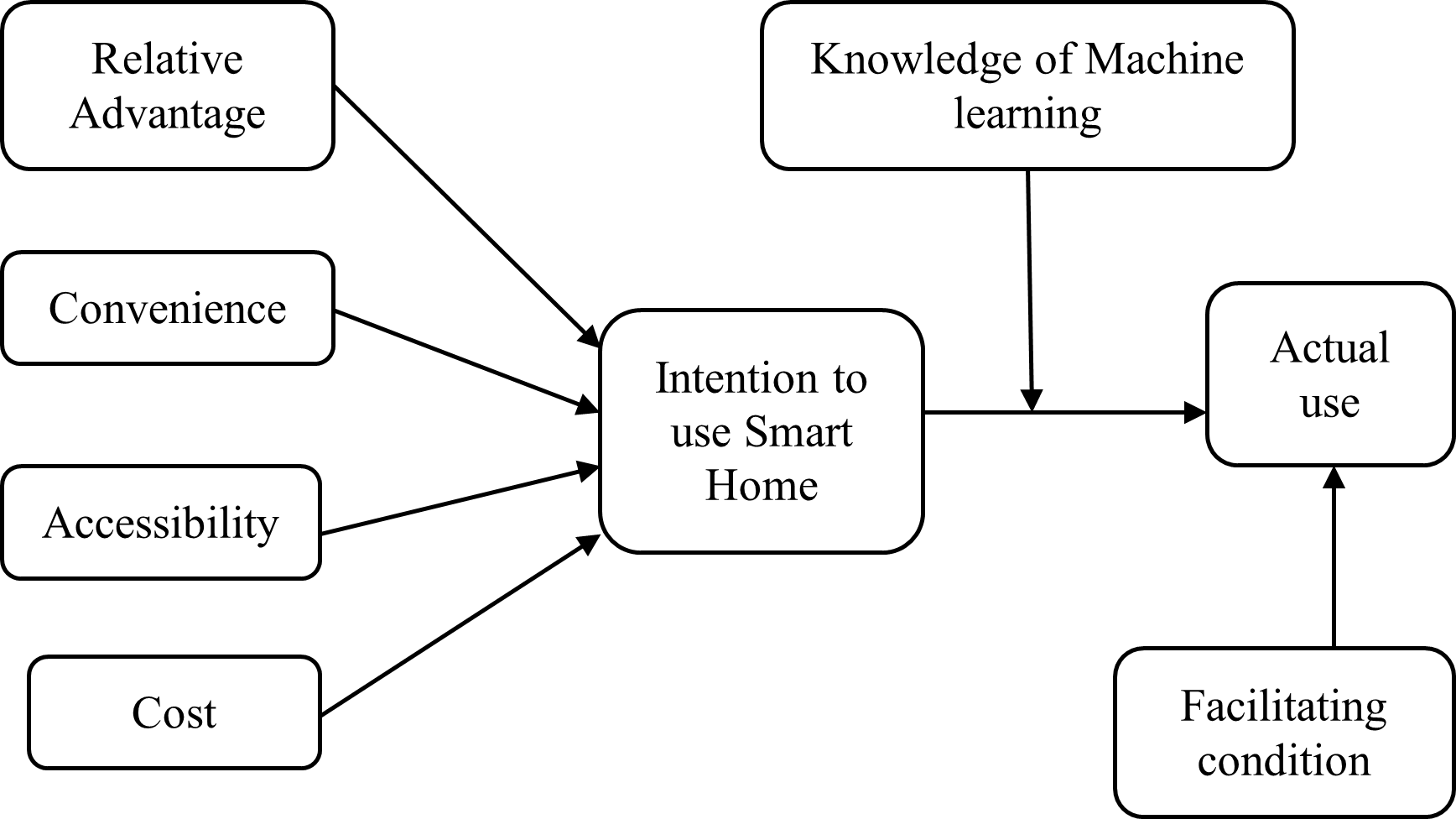
##submission.downloads##
Опубліковано
Як цитувати
Номер
Розділ
Ліцензія
Авторське право (c) 2022 Baraa Sharef

Ця робота ліцензується відповідно до Creative Commons Attribution 4.0 International License.
Закріплення та умови передачі авторських прав (ідентифікація авторства) здійснюється у Ліцензійному договорі. Зокрема, автори залишають за собою право на авторство свого рукопису та передають журналу право першої публікації цієї роботи на умовах ліцензії Creative Commons CC BY. При цьому вони мають право укладати самостійно додаткові угоди, що стосуються неексклюзивного поширення роботи у тому вигляді, в якому вона була опублікована цим журналом, але за умови збереження посилання на першу публікацію статті в цьому журналі.
Ліцензійний договір – це документ, в якому автор гарантує, що володіє усіма авторськими правами на твір (рукопис, статтю, тощо).
Автори, підписуючи Ліцензійний договір з ПП «ТЕХНОЛОГІЧНИЙ ЦЕНТР», мають усі права на подальше використання свого твору за умови посилання на наше видання, в якому твір опублікований. Відповідно до умов Ліцензійного договору, Видавець ПП «ТЕХНОЛОГІЧНИЙ ЦЕНТР» не забирає ваші авторські права та отримує від авторів дозвіл на використання та розповсюдження публікації через світові наукові ресурси (власні електронні ресурси, наукометричні бази даних, репозитарії, бібліотеки тощо).
За відсутності підписаного Ліцензійного договору або за відсутністю вказаних в цьому договорі ідентифікаторів, що дають змогу ідентифікувати особу автора, редакція не має права працювати з рукописом.
Важливо пам’ятати, що існує і інший тип угоди між авторами та видавцями – коли авторські права передаються від авторів до видавця. В такому разі автори втрачають права власності на свій твір та не можуть його використовувати в будь-який спосіб.