Застосування машинного навчання для покращення зображення текстурного типу
DOI:
https://doi.org/10.15587/1729-4061.2023.275984Ключові слова:
обробка зображень, SRGAN, ERSGAN, VGG19, MobileNet2V, ResNet152V2, машинне навчання, надроздільна здатністьАнотація
Робота присвячена методам машинного навчання, орієнтованим на покращення зображень текстурного типу, а саме на поліпшення об'єктів на зображеннях. Метою дослідження є розробка алгоритмів покращення зображень та визначення точності розглянутих моделей для покращення даного типу зображень. Незважаючи на те, що використовувані в даний час системи цифрової обробки зображень зазвичай забезпечують високу якість зображень, зовнішні фактори або навіть системні обмеження можуть привести до того, що зображення в багатьох областях науки матимуть низьку якість та роздільну здатність. Тому розглядаються порогові значення для обробки зображень у певній галузі науки.
Першим етапом обробки зображень є покращення зображення. Питання обробки зображень сигналів залишаються у центрі уваги різних фахівців. В даний час, поряд з розвитком інформаційних технологій, одним із актуальних завдань є автоматичне покращення зображень, що використовуються в будь-якій галузі науки. Зображення аналізувалися як об'єкти: державні номерні знаки автомобілів, особи, ділянки поля на супутникових знімках.
У даній роботі ми пропонуємо використовувати моделі генеративно-змагальної мережі надроздільної здатності (SRGAN), розширених генеративно-змагальних мереж надроздільної здатності (ERSGAN). Для цього був проведений експеримент, метою якого було перенавчання навченої моделі ESRGAN з трьома різними архітектурами згорткової нейронної мережі, тобто VGG19, MobileNet2V, ResNet152V2 для додавання втрат сприйняття (за пікселями), а також додавання різкості до прогнозування тестового зображення та порівняння продуктивності кожної перенавченої моделі. В результаті дослідження використання згорткових нейронних мереж для покращення зображення показало високу точність, тобто в середньому ESRGAN+MobileNetV2 – 91 %, ESRGAN+VGG19 – 86 %, ESRGAN+ResNet152V2 – 96 %
Спонсор дослідження
- For providing data on agricultural crops of Northern Kazakhstan in the preparation of this article, the author expresses gratitude to the Scientific and Production Center of Grain Farming named after A. I. Barayev.
Посилання
- Yessenova, M., Abdikerimova, G., Adilova, A., Yerzhanova, A., Kakabayev, N., Ayazbaev, T. et al. (2022). Identification of factors that negatively affect the growth of agricultural crops by methods of orthogonal transformations. Eastern-European Journal of Enterprise Technologies, 3 (2 (117)), 39–47. doi: https://doi.org/10.15587/1729-4061.2022.257431
- Yessenova, M., Abdikerimova, G., Baitemirova, N., Mukhamedrakhimova, G., Mukhamedrakhimov, K., Sattybaeva, Z. et al. (2022). The applicability of informative textural features for the detection of factors negatively influencing the growth of wheat on aerial images. Eastern-European Journal of Enterprise Technologies, 4 (2 (118)), 51–58. doi: https://doi.org/10.15587/1729-4061.2022.263433
- Yessenova, M., Abdikerimova, G., Ayazbaev, T., Murzabekova, G., Ismailova, A., Beldeubayeva, Z. et al. (2023). The effectiveness of methods and algorithms for detecting and isolating factors that negatively affect the growth of crops. International Journal of Electrical and Computer Engineering (IJECE), 13 (2), 1669. doi: https://doi.org/10.11591/ijece.v13i2.pp1669-1679
- Yerzhanova, A., Kassymova, A., Abdikerimova, G., Abdimomynova, M., Tashenova, Z., Nurlybaeva, E. (2021). Analysis of the spectral properties of wheat growth in different vegetation periods. Eastern-European Journal of Enterprise Technologies, 6 (2 (114)), 96–102. doi: https://doi.org/10.15587/1729-4061.2021.249278
- Phiri, D., Simwanda, M., Salekin, S., Nyirenda, V., Murayama, Y., Ranagalage, M. (2020). Sentinel-2 Data for Land Cover/Use Mapping: A Review. Remote Sensing, 12 (14), 2291. doi: https://doi.org/10.3390/rs12142291
- Wang, X., Yu, K., Wu, S., Gu, J., Liu, Y., Dong, C. et al. (2019). ESRGAN: Enhanced Super-Resolution Generative Adversarial Networks. Computer Vision – ECCV 2018 Workshops, 63–79. doi: https://doi.org/10.1007/978-3-030-11021-5_5
- Wang, X., Xie, L., Dong, C., Shan, Y. (2021). Real-ESRGAN: Training Real-World Blind Super-Resolution with Pure Synthetic Data. 2021 IEEE/CVF International Conference on Computer Vision Workshops (ICCVW). doi: https://doi.org/10.1109/iccvw54120.2021.00217
- Clabaut, É., Lemelin, M., Germain, M., Bouroubi, Y., St-Pierre, T. (2021). Model Specialization for the Use of ESRGAN on Satellite and Airborne Imagery. Remote Sensing, 13 (20), 4044. doi: https://doi.org/10.3390/rs13204044
- Zouch, W., Sagga, D., Echtioui, A., Khemakhem, R., Ghorbel, M., Mhiri, C., Hamida, A. B. (2022). Detection of COVID-19 from CT and Chest X-ray Images Using Deep Learning Models. Annals of Biomedical Engineering, 50 (7), 825–835. doi: https://doi.org/10.1007/s10439-022-02958-5
- Yamashita, K., Markov, K. (2020). Medical Image Enhancement Using Super Resolution Methods. Computational Science – ICCS 2020, 496–508. doi: https://doi.org/10.1007/978-3-030-50426-7_37
- Dou, X., Li, C., Shi, Q., Liu, M. (2020). Super-Resolution for Hyperspectral Remote Sensing Images Based on the 3D Attention-SRGAN Network. Remote Sensing, 12 (7), 1204. doi: https://doi.org/10.3390/rs12071204
- Zhou, S., Yu, L., Jin, M. (2022). Texture transformer super-resolution for low-dose computed tomography. Biomedical Physics & Engineering Express, 8 (6), 065024. doi: https://doi.org/10.1088/2057-1976/ac9da7
- Kang, X., Liu, L., Ma, H. (2021). ESR-GAN: Environmental Signal Reconstruction Learning With Generative Adversarial Network. IEEE Internet of Things Journal, 8 (1), 636–646. doi: https://doi.org/10.1109/jiot.2020.3018621
- Jaworek-Korjakowska, J., Kleczek, P., Gorgon, M. (2019). Melanoma Thickness Prediction Based on Convolutional Neural Network With VGG-19 Model Transfer Learning. 2019 IEEE/CVF Conference on Computer Vision and Pattern Recognition Workshops (CVPRW). doi: https://doi.org/10.1109/cvprw.2019.00333
- Pan, H., Pang, Z., Wang, Y., Wang, Y., Chen, L. (2020). A New Image Recognition and Classification Method Combining Transfer Learning Algorithm and MobileNet Model for Welding Defects. IEEE Access, 8, 119951–119960. doi: https://doi.org/10.1109/access.2020.3005450
- Alrashedy, H. H. N., Almansour, A. F., Ibrahim, D. M., Hammoudeh, M. A. A. (2022). BrainGAN: Brain MRI Image Generation and Classification Framework Using GAN Architectures and CNN Models. Sensors, 22 (11), 4297. doi: https://doi.org/10.3390/s22114297
- Zhang, W., Liu, Y., Dong, C., Qiao, Y. (2019). RankSRGAN: Generative Adversarial Networks With Ranker for Image Super-Resolution. 2019 IEEE/CVF International Conference on Computer Vision (ICCV). doi: https://doi.org/10.1109/iccv.2019.00319
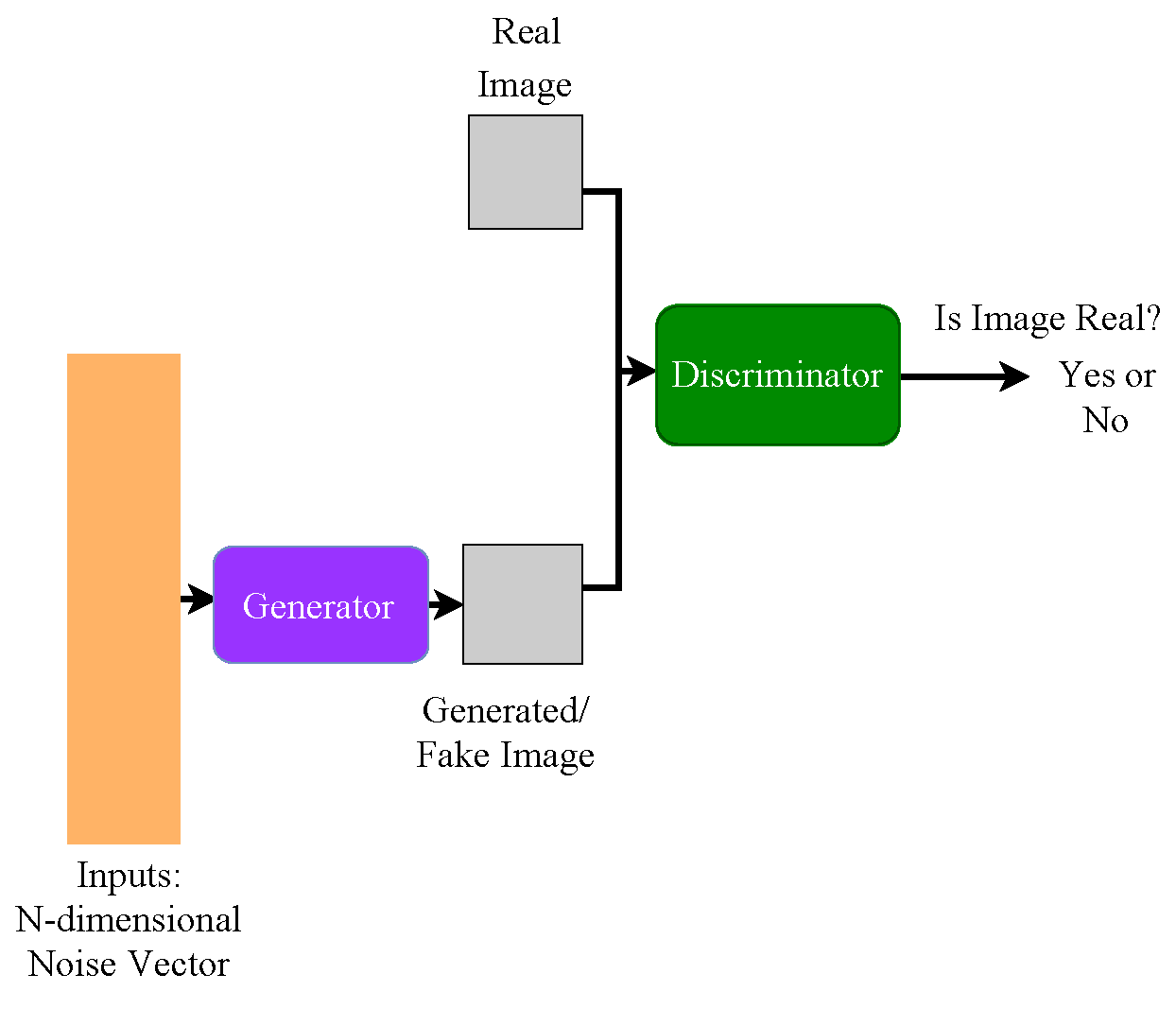
##submission.downloads##
Опубліковано
Як цитувати
Номер
Розділ
Ліцензія
Авторське право (c) 2023 Jamalbek Tussupov, Kairat Kozhabai, Aigulim Bayegizova, Leila Kassenova, Zhanat Manbetova, Natalya Glazyrina, Bersugir Mukhamedi, Miras Yeginbayev

Ця робота ліцензується відповідно до Creative Commons Attribution 4.0 International License.
Закріплення та умови передачі авторських прав (ідентифікація авторства) здійснюється у Ліцензійному договорі. Зокрема, автори залишають за собою право на авторство свого рукопису та передають журналу право першої публікації цієї роботи на умовах ліцензії Creative Commons CC BY. При цьому вони мають право укладати самостійно додаткові угоди, що стосуються неексклюзивного поширення роботи у тому вигляді, в якому вона була опублікована цим журналом, але за умови збереження посилання на першу публікацію статті в цьому журналі.
Ліцензійний договір – це документ, в якому автор гарантує, що володіє усіма авторськими правами на твір (рукопис, статтю, тощо).
Автори, підписуючи Ліцензійний договір з ПП «ТЕХНОЛОГІЧНИЙ ЦЕНТР», мають усі права на подальше використання свого твору за умови посилання на наше видання, в якому твір опублікований. Відповідно до умов Ліцензійного договору, Видавець ПП «ТЕХНОЛОГІЧНИЙ ЦЕНТР» не забирає ваші авторські права та отримує від авторів дозвіл на використання та розповсюдження публікації через світові наукові ресурси (власні електронні ресурси, наукометричні бази даних, репозитарії, бібліотеки тощо).
За відсутності підписаного Ліцензійного договору або за відсутністю вказаних в цьому договорі ідентифікаторів, що дають змогу ідентифікувати особу автора, редакція не має права працювати з рукописом.
Важливо пам’ятати, що існує і інший тип угоди між авторами та видавцями – коли авторські права передаються від авторів до видавця. В такому разі автори втрачають права власності на свій твір та не можуть його використовувати в будь-який спосіб.