Визначення психо-емоційного стану спостереженого на основі аналізу відеоспостереження
DOI:
https://doi.org/10.15587/1729-4061.2024.296500Ключові слова:
комп’ютерний зір, фізична агресія, емоційна реакція, булінг, модельне навчання, нейронна мережаАнотація
У цій роботі розроблено систему визначення психоемоційного стану спостережуваних людей на основі аналізу відеоспостереження із застосуванням технології штучного інтелекту з використанням апаратно-програмних засобів, таких як PoseNet, PyTorch, SQLite, FastAPI та Flask. У багатьох сферах людської діяльності існує нагальна потреба в системі спостереження, яка могла б надійно функціонувати та виявляти підозрілу діяльність. Щоб вирішити цю проблему, у цій статті пропонується нова основа для системи спостереження в реальному часі, яка автоматично виявляє аномальну діяльність людини.
Система була протестована та перевірена в реальних умовах. Найкращі результати показали результати тестування програмних моделей штучного інтелекту (оцінка f1 зі значеннями 0,98–0,99). Середньозважене значення метрики f1-score становило 0,96, що є досить високим значенням. Використання PoseNet, реалізованого за допомогою PyTorch, дозволило нам точно визначити позу людини на відео та отримати інформацію про положення різних частин тіла. Особливість роботи полягає в розробці моделей штучного інтелекту для автоматичного виявлення можливої фізичної агресії на відео, в методах формування оптимального набору ознак для розробки моделей ШІ, які ідентифікують агресора та жертву булінгу.
Розроблена система має потенціал бути корисним інструментом у різних сферах, таких як психологія, медицина, безпека та інших, де важливо аналізувати емоційний стан людей на основі їх фізичних проявів. Отримані прикладні результати можуть бути використані в навчальних закладах та сферах, де необхідний відеоаналіз
Посилання
- Bauman, S. (2016). Do We Need More Measures of Bullying? Journal of Adolescent Health, 59 (5), 487–488. https://doi.org/10.1016/j.jadohealth.2016.08.021
- Al-Nawashi, M., Al-Hazaimeh, O. M., Saraee, M. (2016). A novel framework for intelligent surveillance system based on abnormal human activity detection in academic environments. Neural Computing and Applications, 28 (S1), 565–572. https://doi.org/10.1007/s00521-016-2363-z
- Seldin, M., Yanez, C. (2019). Student Reports of Bullying: Results from the 2017 School Crime Supplement to the National Crime Victimization Survey. Web Tables. NCES 2019–054. National Center for Education Statistics. Available at: https://nces.ed.gov/pubs2019/2019054.pdf
- McCarthy, R. J., Elson, M. (2018). A Conceptual Review of Lab-Based Aggression Paradigms. Collabra: Psychology, 4 (1). https://doi.org/10.1525/collabra.104
- Parrott, D. J., Zeichner, A. (2002). Effects of alcohol and trait anger on physical aggression in men. Journal of Studies on Alcohol, 63 (2), 196–204. https://doi.org/10.15288/jsa.2002.63.196
- Allen, J. J., Anderson, C. A. (2017). Aggression and Violence: Definitions and Distinctions. The Wiley Handbook of Violence and Aggression, 1–14. https://doi.org/10.1002/9781119057574.whbva001
- Zhou, P., Ding, Q., Luo, H., Hou, X. (2018). Violence detection in surveillance video using low-level features. PLOS ONE, 13 (10), e0203668. https://doi.org/10.1371/journal.pone.0203668
- Hassner, T., Itcher, Y., Kliper-Gross, O. (2012). Violent flows: Real-time detection of violent crowd behavior. 2012 IEEE Computer Society Conference on Computer Vision and Pattern Recognition Workshops. https://doi.org/10.1109/cvprw.2012.6239348
- Ullah, F. U. M., Ullah, A., Muhammad, K., Haq, I. U., Baik, S. W. (2019). Violence Detection Using Spatiotemporal Features with 3D Convolutional Neural Network. Sensors, 19 (11), 2472. https://doi.org/10.3390/s19112472
- Amirgaliyev, Y. N., Bukenova, I. N., Bukenov, G. S., Kenshimov, C. A. (2023). Software solutions for the recognition violent movements by video. Bulletin of East Kazakhstan Technical University, 2, 31–42.
- Peixoto, B. M., Avila, S., Dias, Z., Rocha, A. (2018). Breaking down violence. Proceedings of the 13th International Conference on Availability, Reliability and Security. https://doi.org/10.1145/3230833.3232809
- Song, D., Kim, C., Park, S.-K. (2018). A multi-temporal framework for high-level activity analysis: Violent event detection in visual surveillance. Information Sciences, 447, 83–103. https://doi.org/10.1016/j.ins.2018.02.065
- Carneiro, S. A., da Silva, G. P., Guimaraes, S. J. F., Pedrini, H. (2019). Fight Detection in Video Sequences Based on Multi-Stream Convolutional Neural Networks. 2019 32nd SIBGRAPI Conference on Graphics, Patterns and Images (SIBGRAPI). https://doi.org/10.1109/sibgrapi.2019.00010
- Febin, I. P., Jayasree, K., Joy, P. T. (2019). Violence detection in videos for an intelligent surveillance system using MoBSIFT and movement filtering algorithm. Pattern Analysis and Applications, 23 (2), 611–623. https://doi.org/10.1007/s10044-019-00821-3
- Marinoiu, E., Zanfir, M., Olaru, V., Sminchisescu, C. (2018). 3D Human Sensing, Action and Emotion Recognition in Robot Assisted Therapy of Children with Autism. 2018 IEEE/CVF Conference on Computer Vision and Pattern Recognition. https://doi.org/10.1109/cvpr.2018.00230
- Koppula, H. S., Gupta, R., Saxena, A. (2013). Learning human activities and object affordances from RGB-D videos. The International Journal of Robotics Research, 32 (8), 951–970. https://doi.org/10.1177/0278364913478446
- Bermejo Nievas, E., Deniz Suarez, O., Bueno García, G., Sukthankar, R. (2011). Violence Detection in Video Using Computer Vision Techniques. Lecture Notes in Computer Science, 332–339. https://doi.org/10.1007/978-3-642-23678-5_39
- Zhou, P., Ding, Q., Luo, H., Hou, X. (2017). Violent Interaction Detection in Video Based on Deep Learning. Journal of Physics: Conference Series, 844, 012044. https://doi.org/10.1088/1742-6596/844/1/012044
- Pawar, K., Attar, V. (2018). Deep learning approaches for video-based anomalous activity detection. World Wide Web, 22 (2), 571–601. https://doi.org/10.1007/s11280-018-0582-1
- Zhao, H., Torralba, A., Torresani, L., Yan, Z. (2019). HACS: Human Action Clips and Segments Dataset for Recognition and Temporal Localization. 2019 IEEE/CVF International Conference on Computer Vision (ICCV). https://doi.org/10.1109/iccv.2019.00876
- Olweus, D. (1978). Aggression in the schools: Bullies and whipping boys. Hemisphere.
- Solberg, M. E., Olweus, D. (2003). Prevalence estimation of school bullying with the Olweus Bully/Victim Questionnaire. Aggressive Behavior, 29 (3), 239–268. https://doi.org/10.1002/ab.10047
- Shetgiri, R. (2013). Bullying and Victimization Among Children. Advances in Pediatrics, 60 (1), 33–51. https://doi.org/10.1016/j.yapd.2013.04.004
- Fung, A. L. C. (2019). Adolescent Reactive and Proactive Aggression, and Bullying in Hong Kong: Prevalence, Psychosocial Correlates, and Prevention. Journal of Adolescent Health, 64 (6), S65–S72. https://doi.org/10.1016/j.jadohealth.2018.09.018
- Lereya, S. T., Copeland, W. E., Costello, E. J., Wolke, D. (2015). Adult mental health consequences of peer bullying and maltreatment in childhood: two cohorts in two countries. The Lancet Psychiatry, 2 (6), 524–531. https://doi.org/10.1016/s2215-0366(15)00165-0
- Buch-Frohlich, A., Paradis, A., Hébert, M., Cyr, M., Frappier, J.-Y. (2019). Bullying and sexual harassment as predictors of suicidality in sexually abused adolescent girls. International Journal of Victimology, 35, 63–73.
- Yang, T., Guo, L., Hong, F., Wang, Z., Yu, Y., Lu, C. (2020). Association Between Bullying and Suicidal Behavior Among Chinese Adolescents: An Analysis of Gender Differences. Psychology Research and Behavior Management, Volume 13, 89–96. https://doi.org/10.2147/prbm.s228007
- Lloyd, K., Rosin, P. L., Marshall, D., Moore, S. C. (2017). Detecting violent and abnormal crowd activity using temporal analysis of grey level co-occurrence matrix (GLCM)-based texture measures. Machine Vision and Applications, 28 (3-4), 361–371. https://doi.org/10.1007/s00138-017-0830-x
- Bilinski, P., Bremond, F. (2016). Human violence recognition and detection in surveillance videos. 2016 13th IEEE International Conference on Advanced Video and Signal Based Surveillance (AVSS). https://doi.org/10.1109/avss.2016.7738019
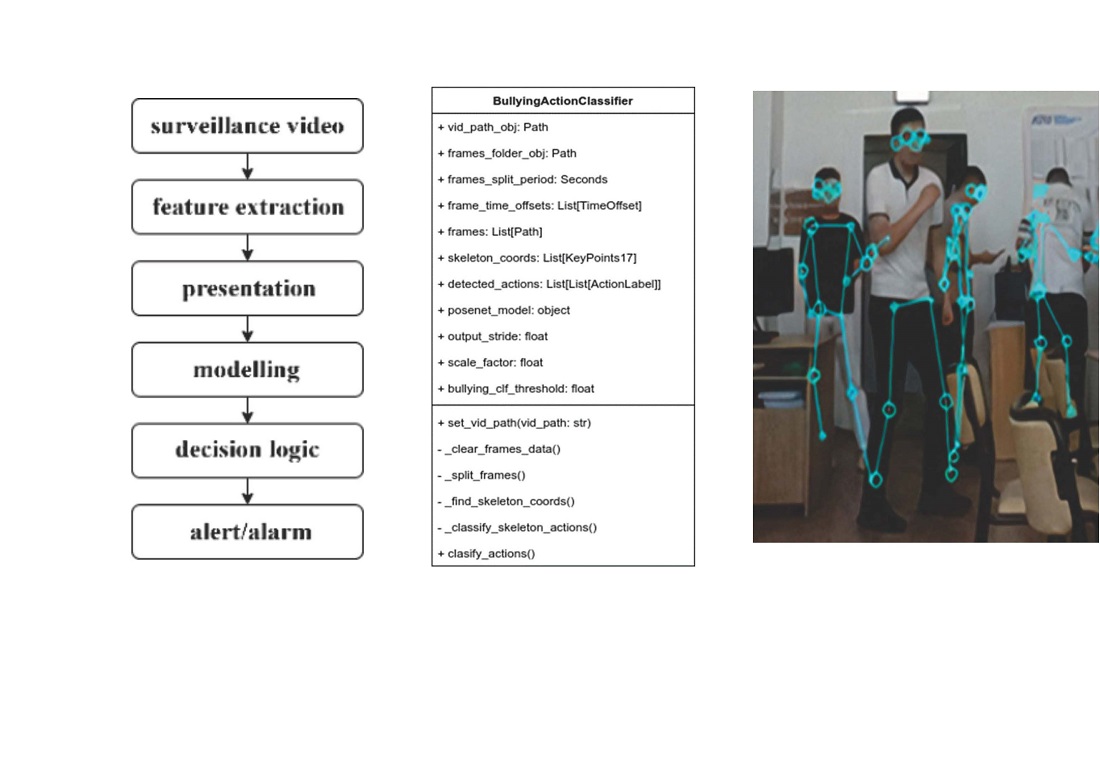
##submission.downloads##
Опубліковано
Як цитувати
Номер
Розділ
Ліцензія
Авторське право (c) 2024 Yedilkhan Amirgaliyev, Iurii Krak, Indira Bukenova, Bayan Kazangapova, Gani Bukenov

Ця робота ліцензується відповідно до Creative Commons Attribution 4.0 International License.
Закріплення та умови передачі авторських прав (ідентифікація авторства) здійснюється у Ліцензійному договорі. Зокрема, автори залишають за собою право на авторство свого рукопису та передають журналу право першої публікації цієї роботи на умовах ліцензії Creative Commons CC BY. При цьому вони мають право укладати самостійно додаткові угоди, що стосуються неексклюзивного поширення роботи у тому вигляді, в якому вона була опублікована цим журналом, але за умови збереження посилання на першу публікацію статті в цьому журналі.
Ліцензійний договір – це документ, в якому автор гарантує, що володіє усіма авторськими правами на твір (рукопис, статтю, тощо).
Автори, підписуючи Ліцензійний договір з ПП «ТЕХНОЛОГІЧНИЙ ЦЕНТР», мають усі права на подальше використання свого твору за умови посилання на наше видання, в якому твір опублікований. Відповідно до умов Ліцензійного договору, Видавець ПП «ТЕХНОЛОГІЧНИЙ ЦЕНТР» не забирає ваші авторські права та отримує від авторів дозвіл на використання та розповсюдження публікації через світові наукові ресурси (власні електронні ресурси, наукометричні бази даних, репозитарії, бібліотеки тощо).
За відсутності підписаного Ліцензійного договору або за відсутністю вказаних в цьому договорі ідентифікаторів, що дають змогу ідентифікувати особу автора, редакція не має права працювати з рукописом.
Важливо пам’ятати, що існує і інший тип угоди між авторами та видавцями – коли авторські права передаються від авторів до видавця. В такому разі автори втрачають права власності на свій твір та не можуть його використовувати в будь-який спосіб.