Реалізація алгоритму фільтру Калмана для оптимізації розрахунку значення відстані ультразвукового датчика в системі закону Гука
DOI:
https://doi.org/10.15587/1729-4061.2024.296667Ключові слова:
алгоритм фільтра Калмана, параметри відстані, ультразвуковий датчик, закон ГукаАнотація
Алгоритм фільтра Калмана дуже важливий як метод рекурсивного алгоритму для оптимізації виходу датчика від систем вимірювання фізичних параметрів, особливо від систем демонстрації фізичних практикумів. Одна з демонстраційних систем вимірювання параметрів відстані, яка використовується в системі демонстрації закону Гука, використовується в практичних заняттях з фізики, система має проблеми, пов’язані з коливанням або нестабільністю виходу датчика. Це дослідження реалізує алгоритм фільтра Калмана на ескізі Arduino IDE для зменшення шуму, який з’являється на виході ультразвукового датчика. Методологія, використана в цьому дослідженні, включає застосування алгоритму фільтра Калмана до ескізу Arduino IDE із значенням змінної рівняння алгоритму фільтра Калмана, модифікованим на значення R=10, H=1, і Q=1, і повертає відфільтроване вихідне значення Калмана. Результати вихідних даних Arduino експортуються в Ms. Excel для подальшого аналізу та створення відфільтрованого графіка вихідного сигналу ультразвукового датчика в порівнянні без використання фільтра Калмана. Фільтрація вихідного шуму ультразвукового датчика ефективно зменшує шум, показуючи зменшення значення середньоквадратичної помилки і отримуючи найкращу продуктивність до 89,23 %. Точність результатів фільтрації за допомогою фільтра Калмана можна побачити з розрахунку того, що постійна пружини відфільтрованих металевих матеріалів менша, ніж постійна пружини традиційного вимірювання. Точні та ефективні результати з реалізацією алгоритму фільтра Калмана можна розробити для змінних значень параметрів відстані та змінних алгоритму фільтра Калмана (R, Q і H) з іншими варіаціями значень, особливо змінними, які створюють криві фільтрації, близькі до прямих. Було зроблено висновок, що алгоритм фільтра Калмана зміг покращити продуктивність системи підтвердження закону Гука
Спонсор дослідження
- We express our gratitude to Jenderal Soedirman University Indonesia for supporting the implementation of this research so that this research runs well and smoothly.
Посилання
- T. Shao, Z. Duan, Q. Ge, and H. Liu, “Recursive performance ranking of Kalman filter with mismatched noise covariances,” IET Control Theory Appl., vol. 13, no. 4, pp. 459–466, Mar. 2019, doi: 10.1049/iet-cta.2018.5064.
- M. Masyruhan, U. Pratiwi, and Y. Al Hakim, “Perancangan Alat Peraga Hukum Hooke Berbasis Mikrokontroler Arduino Sebagai Media Pembelajaran Fisika,” SPEKTRA J. Kaji. Pendidik. Sains, vol. 6, no. 2, pp. 134–145, 2020.
- J. Xu, Y. Wang, K. Xu, and T. Zhang, “Feature genes selection using fuzzy rough uncertainty metric for tumor diagnosis,” Comput. Math. Methods Med., vol. 2019, 2019, doi: 10.1155/2019/6705648.
- S. Ura and A. K. Ghosh, “Time latency‐centric signal processing: A perspective of smart manufacturing,” Sensors, vol. 21, no. 21, 2021, doi: 10.3390/s21217336.
- E. N. Budisusila, S. A. D. Prasetyowati, B. Y. Suprapto, and Z. Nawawi, “Neural network training for serial multisensor of autonomous vehicle system,” Int. J. Electr. Comput. Eng., vol. 12, no. 5, pp. 5415–5426, 2022, doi: 10.11591/ijece.v12i5.pp5415-5426.
- A. E. Elwakeel et al., “Design and Validation of a Variable-Rate Control Metering Mechanism and Smart Monitoring System for a High-Precision Sugarcane Transplanter,” Agric., vol. 13, no. 12, pp. 1–20, 2023, doi: 10.3390/agriculture13122218.
- S. R. Utama, A. Firdausi, and G. P. N. Hakim, “Control and Monitoring Automatic Floodgate Based on NodeMCU and IOT with Fuzzy Logic Testing,” J. Robot. Control, vol. 3, no. 1, pp. 14–17, 2022, doi: 10.18196/jrc.v3i1.11199.
- B. Khan, S. Nizamani, N. H. Kazi, S. Nizamani, and S. Nizamani, “A Prototype of Obstacle Avoidance for Autonomous Vehicle,” Iraqi J. Sci., vol. 63, no. 5, pp. 2203–2210, 2022, doi: 10.24996/ijs.2022.63.5.33.
- J. Price, E. Aaberg, C. Mo, and J. Miller, “Tracking Sensor Location by Video Analysis in Double-Shell Tank Inspections,” Appl. Sci., vol. 13, no. 15, 2023, doi: 10.3390/app13158708.
- Z. Czaja, “Simple Measurement Method for Resistive Sensors Based on ADCs of Microcontrollers,” IEEE Sens. J., pp. 1–1, 2023, doi: 10.1109/JSEN.2023.3341214.
- W. Jiang, Z. Luo, W. Wang, J. Liu, and L. Zhang, “Dynamic Sensitivity Evaluation for Force Transducers by Using a Gray-Bootstrap Method,” IEEE Sens. J., vol. 23, no. 23, pp. 29019–29028, Dec. 2023, doi: 10.1109/JSEN.2023.3323783.
- K. M. Khaled and R. S. Hegazy, “Metrological Characterization of Spring Impact Hammer Calibration,” Instrum. Mes. Métrologie, vol. 22, no. 5, pp. 215–221, Oct. 2023, doi: 10.18280/i2m.220505.
- J. R. Barber, “Eigenstrains and Inclusions,” 2022, pp. 457–474.
- U. B. Pili, “Work-based measurement of k with a spring-mass system: a demo of the work done by a variable force,” Phys. Educ., vol. 58, no. 4, p. 043002, Jul. 2023, doi: 10.1088/1361-6552/acdbb2.
- M. S. Al-Din Tahir, S. S. Hassan, and J. S. Chiad, “The mathematical model for lateral stiffness of variable length conical spring,” Pollack Period., vol. 17, no. 2, pp. 31–35, Jun. 2022, doi: 10.1556/606.2021.00494.
- C. Fang, J. Zhang, Y. Huang, X. Liu, and X. Dong, “Orientation dependence of elastic properties of Mg binary alloys: A first-principles study,” Comput. Condens. Matter, vol. 22, p. e00447, Mar. 2020, doi: 10.1016/j.cocom.2019.e00447.
- J. Zhang et al., “Insight into the Influence of Alloying Elements on the Elastic Properties and Strengthening of Copper: A High-Throughput First-Principles Calculations,” Metals (Basel)., vol. 13, no. 5, p. 875, May 2023, doi: 10.3390/met13050875.
- Y. Wei and Z. Chong, “A More Efficient Approach to Demonstrate Hooke’s Law,” Phys. Teach., vol. 60, no. 6, pp. 444–444, Sep. 2022, doi: 10.1119/5.0043860.
- G. A. Garcia, K. M. Wakumoto, and J. J. Brown, “Large-Deflection Nonlinear Mechanics of Curved Cantilevers Under Contact Point Loading,” J. Appl. Mech., vol. 88, no. 12, Dec. 2021, doi: 10.1115/1.4052000.
- V. Ivchenko, “Exploring statically indeterminate: examples allowing a simple resolution,” Phys. Educ., vol. 56, no. 4, p. 043007, Jul. 2021, doi: 10.1088/1361-6552/abf74a.
- J. J. Bissell, A. Ali, and B. J. Postle, “Illustrating dimensionless scaling with Hooke’s law,” Phys. Educ., vol. 57, no. 2, p. 023008, Mar. 2022, doi: 10.1088/1361-6552/ac45c9.
- R. Ahmad, B. Rinner, R. Wazirali, S. K. M. Abujayyab, and R. Almajalid, “Two-Level Sensor Self-Calibration Based on Interpolation and Autoregression for Low-Cost Wireless Sensor Networks,” IEEE Sens. J., vol. 23, no. 20, pp. 25242–25253, Oct. 2023, doi: 10.1109/JSEN.2023.3309759.
- V. M. Deshpande and R. Bhattacharya, “Guaranteed Robust Performance of $mathcal {H}_{infty }$ Filters With Sparse and Low Precision Sensing,” IEEE Trans. Automat. Contr., pp. 1–8, 2023, doi: 10.1109/TAC.2023.3276824.
- G. Schönfelder, “Signal Processing and Calibration,” in Sensors in Science and Technology, Wiesbaden: Springer Fachmedien Wiesbaden, 2022, pp. 745–755.
- S. Kanagasabapathi and M. Thushara, “FORWARD AND BACKWARD STATIC ANALYSIS FOR CRITICAL NUMERICAL ACCURACY IN FLOATING POINT PROGRAMS,” Comput. Sci., vol. 21, no. 2, Apr. 2020, doi: 10.7494/csci.2020.21.2.3421.
- J. Radosz and D. Pleban, “Ultrasonic noise measurements in the work environment,” J. Acoust. Soc. Am., vol. 144, no. 4, pp. 2532–2538, Oct. 2018, doi: 10.1121/1.5063812.
- S. Zhang, Y. Huang, X. Li, and H. Jeong, “Modeling of wave fields generated by ultrasonic transducers using a quasi-Monte Carlo method,” J. Acoust. Soc. Am., vol. 149, no. 1, pp. 7–15, Jan. 2021, doi: 10.1121/10.0002972.
- X. Chen, “Research on Optimal Control Method of Tennis Racket String Diameter Based on Kalman Filter Algorithm,” J. Electr. Comput. Eng., vol. 2022, 2022, doi: 10.1155/2022/9356608.
- X. Li and G. Hao, “Event-Triggered Kalman Filter and Its Performance Analysis,” Sensors, vol. 23, no. 4, pp. 1–13, 2023, doi: 10.3390/s23042202.
- L. Hu, R. Hu, Z. Ma, and W. Jiang, “State of Charge Estimation and Evaluation of Lithium Battery Using Kalman Filter Algorithms,” Materials (Basel)., vol. 15, no. 24, 2022, doi: 10.3390/ma15248744.
- X. Liu and X. Zhang, “State of Charge Estimation for Power Battery Using Improved Extended Kalman Filter Method Based on Neural Network,” Appl. Sci., vol. 13, no. 18, 2023, doi: 10.3390/app131810547.
- Q. Yang, K. Ma, L. Xu, L. Song, X. Li, and Y. Li, “A Joint Estimation Method Based on Kalman Filter of Battery State of Charge and State of Health,” Coatings, vol. 12, no. 8, 2022, doi: 10.3390/coatings12081047.
- Y. Zha, X. Liu, F. Ma, and C. C. Liu, “Vehicle state estimation based on extended Kalman filter and radial basis function neural networks,” Int. J. Distrib. Sens. Networks, vol. 18, no. 6, 2022, doi: 10.1177/15501329221102730.
- D. C. Lucena, R. C. S. Freire, J. M. M. Villanueva, and B. A. Luciano, “An innovative ultrasonic time of flight method based on extended Kalman filter for wind speed measurement,” J. Instrum., vol. 15, no. 04, pp. P04024–P04024, Apr. 2020, doi: 10.1088/1748-0221/15/04/P04024.
- J. C. Fabiano dos Santos, P. P. Pinheiro, and J. A. de Franca, “Recovering of Corrupted Ultrasonic Waves, for Determination of TOF Using the Zero-Crossing Detection Technique,” IEEE Trans. Instrum. Meas., vol. 68, no. 11, pp. 4234–4241, Nov. 2019, doi: 10.1109/TIM.2018.2890326.
- X. Lin et al., “Wearable Sensor-Based Monitoring of Environmental Exposures and the Associated Health Effects: A Review,” Biosensors, vol. 12, no. 12, p. 1131, Dec. 2022, doi: 10.3390/bios12121131.
- A. and Barhoum and Z. Altintas, Advanced Sensor Technology. Elsevier, 2023.
- E. and Hering and G. Schönfelder, Sensors in Science and Technology. Wiesbaden: Springer Fachmedien Wiesbaden, 2022.
- X. Bi, “Infrared Sensors and Ultrasonic Sensors,” 2021, pp. 143–168.
- O. Stepanov and A. Motorin, “Performance Criteria for the Identification of Inertial Sensor Error Models,” Sensors, vol. 19, no. 9, p. 1997, Apr. 2019, doi: 10.3390/s19091997.
- V. Jotsov, O. Kochan, and S. Jun, “Decreasing Influence of the Error Due to Acquired Inhomogeneity of Sensors by the Means of Artificial Intelligence,” 2018, pp. 89–130.
- P. Mercorelli, “A Fault Detection and Data Reconciliation Algorithm in Technical Processes with the Help of Haar Wavelets Packets,” Algorithms, vol. 10, no. 1, p. 13, Jan. 2017, doi: 10.3390/a10010013.
- P. Khatri, K. K. Gupta, and R. K. Gupta, “Drift compensation of commercial water quality sensors using machine learning to extend the calibration lifetime,” J. Ambient Intell. Humaniz. Comput., vol. 12, no. 2, pp. 3091–3099, Feb. 2021, doi: 10.1007/s12652-020-02469-y.
- P. M. Matyakubova, P. R. Ismatullaev, N. I. Avezova, and M. M. Makhmudzhonov, “Block Diagram of APCS of Installations for Wet-Heat Processing of Grain Products,” J. Eng. Phys. Thermophys., vol. 96, no. 6, pp. 1652–1657, Nov. 2023, doi: 10.1007/s10891-023-02835-5.
- Y. Toyoda, Y. Ishibashi, P. Huang, Y. Tateiwa, and H. Watanabe, “Efficiency of Cooperation between Remote Robot Systems with Force Feedback-Comparison with Cooperation between User and Remote Robot System,” Int. J. Mech. Eng. Robot. Res., pp. 900–905, 2020, doi: 10.18178/ijmerr.9.6.900-905.
- B. Lacaze, “Random propagation times for ultrasonics through polyethyilene,” Ultrasonics, vol. 111, p. 106313, Mar. 2021, doi: 10.1016/j.ultras.2020.106313.
- M. Lv, H. Wei, X. Fu, W. Wang, and D. Zhou, “A Loosely Coupled Extended Kalman Filter Algorithm for Agricultural Scene-Based Multi-Sensor Fusion,” Front. Plant Sci., vol. 13, no. April, 2022, doi: 10.3389/fpls.2022.849260.
- C. Schreppel, A. Pfeiffer, J. Ruggaber, and J. Brembeck, “Implementation of a C Library of Kalman Filters for Application on Embedded Systems,” Computers, vol. 11, no. 11, 2022, doi: 10.3390/computers11110165.
- H. Ma, L. Yan, Y. Xia, and M. Fu, Kalman filtering and information fusion. Springer, 2020.
- K. Fronckova and A. Slaby, “Kalman filter employment in image processing,” in Computational Science and Its Applications–ICCSA 2020: 20th International Conference, Cagliari, Italy, July 1–4, 2020, Proceedings, Part I 20, 2020, pp. 833–844.
- Q. Li, R. Li, K. Ji, and W. Dai, “Kalman filter and its application,” in 2015 8th International Conference on Intelligent Networks and Intelligent Systems (ICINIS), 2015, pp. 74–77.
- J. Shu, F. Hamano, and J. Angus, “Application of extended Kalman filter for improving the accuracy and smoothness of Kinect skeleton-joint estimates,” J. Eng. Math., vol. 88, pp. 161–175, 2014.
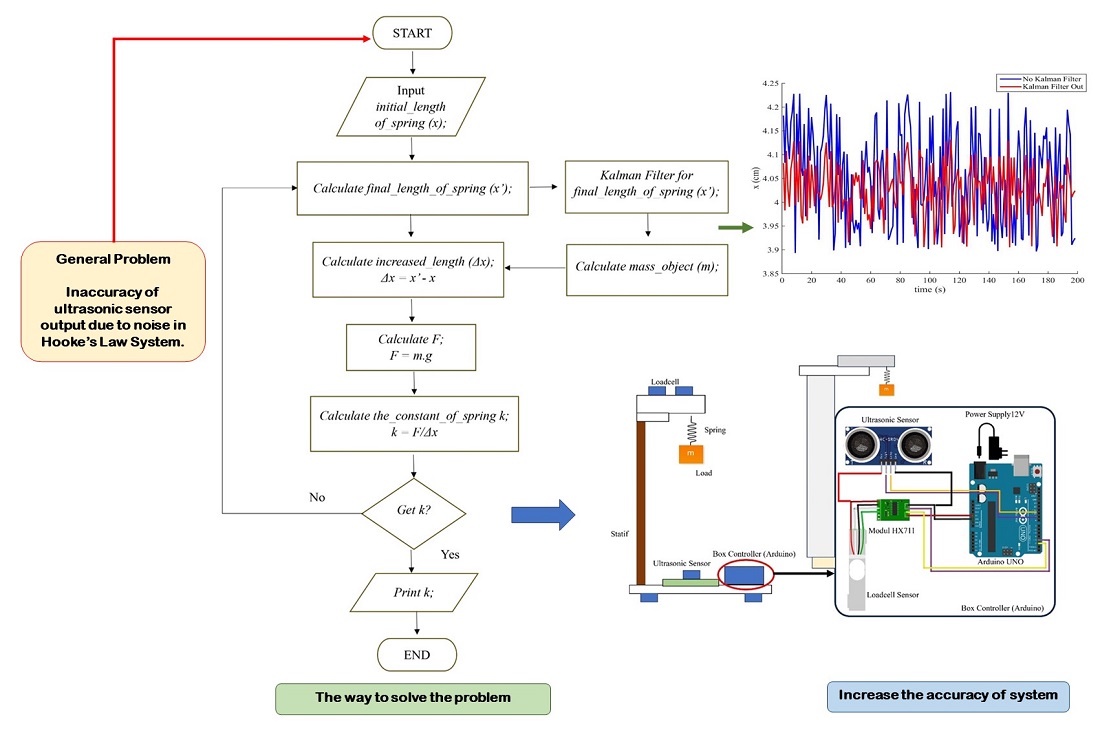
##submission.downloads##
Опубліковано
Як цитувати
Номер
Розділ
Ліцензія
Авторське право (c) 2024 Umi Pratiwi, Imam Fadli, Wahyu Tri Cahyanto, Hartono

Ця робота ліцензується відповідно до Creative Commons Attribution 4.0 International License.
Закріплення та умови передачі авторських прав (ідентифікація авторства) здійснюється у Ліцензійному договорі. Зокрема, автори залишають за собою право на авторство свого рукопису та передають журналу право першої публікації цієї роботи на умовах ліцензії Creative Commons CC BY. При цьому вони мають право укладати самостійно додаткові угоди, що стосуються неексклюзивного поширення роботи у тому вигляді, в якому вона була опублікована цим журналом, але за умови збереження посилання на першу публікацію статті в цьому журналі.
Ліцензійний договір – це документ, в якому автор гарантує, що володіє усіма авторськими правами на твір (рукопис, статтю, тощо).
Автори, підписуючи Ліцензійний договір з ПП «ТЕХНОЛОГІЧНИЙ ЦЕНТР», мають усі права на подальше використання свого твору за умови посилання на наше видання, в якому твір опублікований. Відповідно до умов Ліцензійного договору, Видавець ПП «ТЕХНОЛОГІЧНИЙ ЦЕНТР» не забирає ваші авторські права та отримує від авторів дозвіл на використання та розповсюдження публікації через світові наукові ресурси (власні електронні ресурси, наукометричні бази даних, репозитарії, бібліотеки тощо).
За відсутності підписаного Ліцензійного договору або за відсутністю вказаних в цьому договорі ідентифікаторів, що дають змогу ідентифікувати особу автора, редакція не має права працювати з рукописом.
Важливо пам’ятати, що існує і інший тип угоди між авторами та видавцями – коли авторські права передаються від авторів до видавця. В такому разі автори втрачають права власності на свій твір та не можуть його використовувати в будь-який спосіб.