Визначення епідеміологічних заключень в ідентифікації захворювань з використанням математичних моделей на основі машинного навчання багаторівних структур
DOI:
https://doi.org/10.15587/1729-4061.2024.310522Ключові слова:
математичні моделі SEIR, кластеризація, епідеміологія, багатошарові мережі, машинне навчанняАнотація
Об’єктом дослідження є епідеміологічне групування з використанням математичної моделі SEIR на основі багатошарової мережі на основі машинного навчання. Проблема, що вирішувалася, пов’язана з керуванням епідеміологічними даними у великому масштабі для визначення моделей захворювань та ідентифікації, таких як кількість випадків одужання, кількість випадків інфікування та кількість смертей та демографічних факторів. При цьому традиційні методи ускладнюють виконання таких процесів, як визначення закономірностей та ідентифікація захворювань. Тому необхідно використовувати машинне навчання та математичну модель SEIR (Susceptible-Exposed-Infectious-Covered), яку можна інтегрувати з багатошаровими мережами, щоб підвищити точність і ефективність ідентифікації захворювань і визначення закономірностей. Результати, отримані в результаті цього дослідження, є моделлю, яка може ідентифікувати та визначити закономірності поширення хвороби у великомасштабних епідеміологічних даних. У своєму застосуванні математична модель SEIR буде об’єднана в соціальний рівень і екологічний рівень у багаторівневій мережі. Це дослідження має певний рівень новизни в застосуванні математичної моделі SEIR до багаторівневих мереж і машинного навчання, щоб сформовану модель можна було використовувати для перегляду розподілу епідеміологічних даних для інформації, пов’язаної із захворюваннями. Машинне навчання спрямоване на обробку великомасштабних даних за мінімальний час, що призводить до кластеризації та ідентифікації таких захворювань, як грип, Covid-19 і пневмонія. Це дослідження має точність 94 % за допомогою техніки оцінки MAPE. Є надія, що отриману модель можна буде використовувати у світі охорони здоров’я для картографування захворювань у певних регіонах як еталону для пом’якшення поширення хвороби
Посилання
- Wu, F., Wu, T., Yuce, M. R. (2019). Design and Implementation of a Wearable Sensor Network System for IoT-Connected Safety and Health Applications. 2019 IEEE 5th World Forum on Internet of Things (WF-IoT). https://doi.org/10.1109/wf-iot.2019.8767280
- Liu, J., Zhao, Z., Ji, J., Hu, M. (2020). Research and application of wireless sensor network technology in power transmission and distribution system. Intelligent and Converged Networks, 1 (2), 199–220. https://doi.org/10.23919/icn.2020.0016
- Swamy, S. N., Jadhav, D., Kulkarni, N. (2017). Security threats in the application layer in IOT applications. 2017 International Conference on I-SMAC (IoT in Social, Mobile, Analytics and Cloud) (I-SMAC). https://doi.org/10.1109/i-smac.2017.8058395
- Shivalingagowda, C., Ahmad, H., Jayasree, P. V. Y., Sah, D. K. (2021). Wireless Sensor Network Routing Protocols Using Machine Learning. Architectural Wireless Networks Solutions and Security Issues, 99–120. https://doi.org/10.1007/978-981-16-0386-0_7
- Khutsoane, O., Isong, B., Gasela, N., Abu-Mahfouz, A. M. (2020). WaterGrid-Sense: A LoRa-Based Sensor Node for Industrial IoT Applications. IEEE Sensors Journal, 20 (5), 2722–2729. https://doi.org/10.1109/jsen.2019.2951345
- Wang, A., Dara, R., Yousefinaghani, S., Maier, E., Sharif, S. (2023). A Review of Social Media Data Utilization for the Prediction of Disease Outbreaks and Understanding Public Perception. Big Data and Cognitive Computing, 7 (2), 72. https://doi.org/10.3390/bdcc7020072
- Hajiakhoond Bidoki, N., Mantzaris, A. V., Sukthankar, G. (2019). An LSTM Model for Predicting Cross-Platform Bursts of Social Media Activity. Information, 10 (12), 394. https://doi.org/10.3390/info10120394
- Ertam, F., Kilincer, I. F., Yaman, O., Sengur, A. (2020). A New IoT Application for Dynamic WiFi based Wireless Sensor Network. 2020 International Conference on Electrical Engineering (ICEE). https://doi.org/10.1109/icee49691.2020.9249771
- Yahya, O. H., Alrikabi, H., Aljazaery, I. A. (2020). Reducing the Data Rate in Internet of Things Applications by Using Wireless Sensor Network. International Journal of Online and Biomedical Engineering (IJOE), 16 (03), 107–https://doi.org/10.3991/ijoe.v16i03.13021
- Mejjaouli, S., Babiceanu, R. F. (2015). RFID-wireless sensor networks integration: Decision models and optimization of logistics systems operations. Journal of Manufacturing Systems, 35, 234–245. https://doi.org/10.1016/j.jmsy.2015.02.005
- You, G., Zhu, Y. (2020). Structure and Key Technologies of Wireless Sensor Network. 2020 Cross Strait Radio Science & Wireless Technology Conference (CSRSWTC). https://doi.org/10.1109/csrswtc50769.2020.9372727
- Taherdoost, H. (2023). Enhancing Social Media Platforms with Machine Learning Algorithms and Neural Networks. Algorithms, 16 (6), 271. https://doi.org/10.3390/a16060271
- Gutierrez-Osorio, C., González, F. A., Pedraza, C. A. (2022). Deep Learning Ensemble Model for the Prediction of Traffic Accidents Using Social Media Data. Computers, 11 (9), 126. https://doi.org/10.3390/computers11090126
- Huang, J.-Y., Lee, W.-P., Lee, K.-D. (2022). Predicting Adverse Drug Reactions from Social Media Posts: Data Balance, Feature Selection and Deep Learning. Healthcare, 10 (4), 618. https://doi.org/10.3390/healthcare10040618
- Regulski, K., Opaliński, A., Swadźba, J., Sitkowski, P., Wąsowicz, P., Kwietniewska-Śmietana, A. (2024). Machine Learning Prediction Techniques in the Optimization of Diagnostic Laboratories’ Network Operations. Applied Sciences, 14 (6), 2429. https://doi.org/10.3390/app14062429
- Ghostine, R., Gharamti, M., Hassrouny, S., Hoteit, I. (2021). An Extended SEIR Model with Vaccination for Forecasting the COVID-19 Pandemic in Saudi Arabia Using an Ensemble Kalman Filter. Mathematics, 9 (6), 636. https://doi.org/10.3390/math9060636
- Aljohani, A. (2023). Predictive Analytics and Machine Learning for Real-Time Supply Chain Risk Mitigation and Agility. Sustainability, 15 (20), 15088. https://doi.org/10.3390/su152015088
- Sánchez Lasheras, F. (2021). Predicting the Future-Big Data and Machine Learning. Energies, 14 (23), 8041. https://doi.org/10.3390/en14238041
- He, Z., Yu, J., Gu, T., Yang, D. (2024). Query execution time estimation in graph databases based on graph neural networks. Journal of King Saud University - Computer and Information Sciences, 36 (4), 102018. https://doi.org/10.1016/j.jksuci.2024.102018
- Zhu, L., Zhang, H., Bai, L. (2024). Hierarchical pattern-based complex query of temporal knowledge graph. Knowledge-Based Systems, 284, 111301. https://doi.org/10.1016/j.knosys.2023.111301
- Saleem, F., AL-Ghamdi, A. S. A.-M., Alassafi, M. O., AlGhamdi, S. A. (2022). Machine Learning, Deep Learning, and Mathematical Models to Analyze Forecasting and Epidemiology of COVID-19: A Systematic Literature Review. International Journal of Environmental Research and Public Health, 19 (9), 5099. https://doi.org/10.3390/ijerph19095099
- Gopal, K., Lee, L. S., Seow, H.-V. (2021). Parameter Estimation of Compartmental Epidemiological Model Using Harmony Search Algorithm and Its Variants. Applied Sciences, 11 (3), 1138. https://doi.org/10.3390/app11031138
- Xu, Z., Qian, M. (2023). Predicting Popularity of Viral Content in Social Media through a Temporal-Spatial Cascade Convolutional Learning Framework. Mathematics, 11 (14), 3059. https://doi.org/10.3390/math11143059
- Abu-Salih, B., Al-Tawil, M., Aljarah, I., Faris, H., Wongthongtham, P., Chan, K. Y., Beheshti, A. (2021). Relational Learning Analysis of Social Politics using Knowledge Graph Embedding. Data Mining and Knowledge Discovery, 35 (4), 1497–1536. https://doi.org/10.1007/s10618-021-00760-w
- Malozyomov, B. V., Martyushev, N. V., Sorokova, S. N., Efremenkov, E. A., Valuev, D. V., Qi, M. (2024). Analysis of a Predictive Mathematical Model of Weather Changes Based on Neural Networks. Mathematics, 12 (3), 480. https://doi.org/10.3390/math12030480
- Shafqat, W., Byun, Y.-C. (2019). Topic Predictions and Optimized Recommendation Mechanism Based on Integrated Topic Modeling and Deep Neural Networks in Crowdfunding Platforms. Applied Sciences, 9 (24), 5496. https://doi.org/10.3390/app9245496
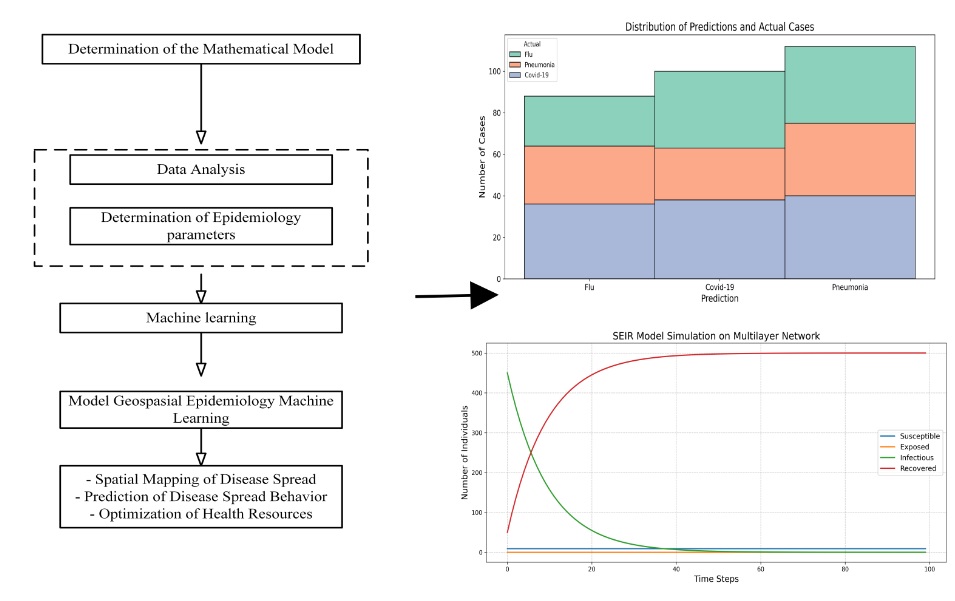
##submission.downloads##
Опубліковано
Як цитувати
Номер
Розділ
Ліцензія
Авторське право (c) 2024 Riah Ukur Ginting, Muhammad Zarlis, Poltak Sihombing, Syahril Efendi

Ця робота ліцензується відповідно до Creative Commons Attribution 4.0 International License.
Закріплення та умови передачі авторських прав (ідентифікація авторства) здійснюється у Ліцензійному договорі. Зокрема, автори залишають за собою право на авторство свого рукопису та передають журналу право першої публікації цієї роботи на умовах ліцензії Creative Commons CC BY. При цьому вони мають право укладати самостійно додаткові угоди, що стосуються неексклюзивного поширення роботи у тому вигляді, в якому вона була опублікована цим журналом, але за умови збереження посилання на першу публікацію статті в цьому журналі.
Ліцензійний договір – це документ, в якому автор гарантує, що володіє усіма авторськими правами на твір (рукопис, статтю, тощо).
Автори, підписуючи Ліцензійний договір з ПП «ТЕХНОЛОГІЧНИЙ ЦЕНТР», мають усі права на подальше використання свого твору за умови посилання на наше видання, в якому твір опублікований. Відповідно до умов Ліцензійного договору, Видавець ПП «ТЕХНОЛОГІЧНИЙ ЦЕНТР» не забирає ваші авторські права та отримує від авторів дозвіл на використання та розповсюдження публікації через світові наукові ресурси (власні електронні ресурси, наукометричні бази даних, репозитарії, бібліотеки тощо).
За відсутності підписаного Ліцензійного договору або за відсутністю вказаних в цьому договорі ідентифікаторів, що дають змогу ідентифікувати особу автора, редакція не має права працювати з рукописом.
Важливо пам’ятати, що існує і інший тип угоди між авторами та видавцями – коли авторські права передаються від авторів до видавця. В такому разі автори втрачають права власності на свій твір та не можуть його використовувати в будь-який спосіб.