Ідентифікація повітряних цілей за допомогою гібридного алгоритму кластеризації
DOI:
https://doi.org/10.15587/1729-4061.2024.314289Ключові слова:
кластеризація небезпеки, лінійне масштабування, навчання без вчителя, комплексний показник, штучний інтелект, повітряна небезпекаАнотація
Досліджується процес створення кластеризатора рівня небезпеки повітряної тривоги.
У дослідженні вирішується проблема підвищення точності кластеризації оцінок рівня небезпеки повітряної тривоги за рахунок створення гібридного алгоритму кластеризатора.
Побудовано кластеризатор повітряних цілей, що передбачає використання модифікованої метрики визначення відстані та об’єднує оцінки рівня повітряної небезпеки прямо у алгоритмі.
Вказані особливості демонструють перевагу над існуючими алгоритмами за критеріями індексів силуету та Девіса-Болдіна. Запропонована модель демонструє індекс силуету 0,72306, а існуюча – 0,3481, а індекс Девіса-Болдіна становить 0,3389; 1,209 відповідно.
Моделі Random Forest Classifier, Gradient Boosting Classifier на оцінках визначених кластеризатором характеризуються більшою точністю, зокрема 0,87; 0,87 відповідно, а існуючих 0,48; 0,49 відповідно.
Особливістю кластеризатора є використання точніших оцінок, що подаються на вхід, які визначаються за принципом взаємодії та лінійного масштабування. Запропонований алгоритм передбачає використання модифікованої метрики хі-квадрат, що включає оцінки індексів безпеки держави. Відмінною рисою запропонованого підходу є використання точніше визначення центів кластерів методом самоорганізаційних карт Кохрена.
Це дозволяє вирішити проблему аналізу й підвищення точності прогнозування рівня повітряних загроз. Отримані результати пояснюються використанням точніших вхідних оцінок й вдало підібраній метриці визначення відстані між кластерами у поєднанні з картами самоорганізації Кохрена.
На практиці отримані результати можуть бути використані при аналізі рівня повітряної небезпеки наземною платформою
Посилання
- O’Shaughnessy, D. (2024). Trends and developments in automatic speech recognition research. Computer Speech & Language, 83, 101538. https://doi.org/10.1016/j.csl.2023.101538
- Seo, D., Kim, S., Oh, S., Kim, S.-H. (2022). K-Means Clustering-Based Safety System in Large-Scale Industrial Site Using Industrial Wireless Sensor Networks. Sensors, 22 (8), 2897. https://doi.org/10.3390/s22082897
- Leal Piedrahita, E. A. (2019). Hierarchical Clustering for Anomalous Traffic Conditions Detection in Power Substations. Ciencia e Ingeniería Neogranadina, 30 (1), 75–88. https://doi.org/10.18359/rcin.4236
- Yanko, A., Koshman, S., Krasnobayev, V. (2017). Algorithms of data processing in the residual classes system. 2017 4th International Scientific-Practical Conference Problems of Infocommunications. Science and Technology (PIC S&T), 117–121. https://doi.org/10.1109/infocommst.2017.8246363
- Onyshchenko, S., Haitan, O., Yanko A., Zdorenko, Y., Rudenko, O. (2024). Method for detection of the modified DDoS cyber attacks on a web resource of an Information and Telecommunication Network based on the use of intelligent systems. Proceedings of the Modern Data Science Technologies Workshop (MoDaST 2024), 219–235. Available at: https://ceur-ws.org/Vol-3723/paper12.pdf
- Krasnobayev, V., Yanko, A., Hlushko, A. (2023). Information Security of the National Economy Based on an Effective Data Control Method. Journal of International Commerce, Economics and Policy, 14 (03). https://doi.org/10.1142/s1793993323500217
- Zdorenko, Y., Lavrut, O., Lavrut, T., Nastishin, Y. (2020). Method of Power Adaptation for Signals Emitted in a Wireless Network in Terms of Neuro-Fuzzy System. Wireless Personal Communications, 115 (1), 597–609. https://doi.org/10.1007/s11277-020-07588-5
- Fachrizal, F., Zarlis, M., Sihombing, P., Suherman, S. (2024). Optimization of the LEACH algorithm in the selection of cluster heads based on residual energy in wireless sensor networks. Eastern-European Journal of Enterprise Technologies, 1 (9 (127)), 14–21. https://doi.org/10.15587/1729-4061.2024.298268
- Szalontai, B., Debreczeny, M., Fintor, K., Bagyinka, Cs. (2020). SVD-clustering, a general image-analyzing method explained and demonstrated on model and Raman micro-spectroscopic maps. Scientific Reports, 10 (1). https://doi.org/10.1038/s41598-020-61206-9
- Creţulescu, R. G., Morariu, D. I., Breazu, M., Volovici, D. (2019). DBSCAN Algorithm for Document Clustering. International Journal of Advanced Statistics and IT&C for Economics and Life Sciences, 9 (1), 58–66. https://doi.org/10.2478/ijasitels-2019-0007
- Gan, H., Yang, Z., Zhou, R. (2023). Adaptive safety-aware semi-supervised clustering. Expert Systems with Applications, 212, 118751. https://doi.org/10.1016/j.eswa.2022.118751
- Powell, B. A. (2022). Role-based lateral movement detection with unsupervised learning. Intelligent Systems with Applications, 16, 200106. https://doi.org/10.1016/j.iswa.2022.200106
- Pérez-Ortega, J., Nely Almanza-Ortega, N., Vega-Villalobos, A., Pazos-Rangel, R., Zavala-Díaz, C., Martínez-Rebollar, A. (2020). The K-Means Algorithm Evolution. Introduction to Data Science and Machine Learning. https://doi.org/10.5772/intechopen.85447
- Zhao, Y., Zhou, X. (2021). K-means Clustering Algorithm and Its Improvement Research. Journal of Physics: Conference Series, 1873 (1), 012074. https://doi.org/10.1088/1742-6596/1873/1/012074
- Zhao, H. (2022). Design and Implementation of an Improved K-Means Clustering Algorithm. Mobile Information Systems, 2022, 1–10. https://doi.org/10.1155/2022/6041484
- Wiharto, W., Suryani, E. (2020). The Comparison of Clustering Algorithms K-Means and Fuzzy C-Means for Segmentation Retinal Blood Vessels. Acta Informatica Medica, 28 (1), 42. https://doi.org/10.5455/aim.2020.28.42-47
- Mohassel, P., Rosulek, M., Trieu, N. (2020). Practical Privacy-Preserving K-means Clustering. Proceedings on Privacy Enhancing Technologies, 2020 (4), 414–433. https://doi.org/10.2478/popets-2020-0080
- Hu, H., Liu, J., Zhang, X., Fang, M. (2023). An Effective and Adaptable K-means Algorithm for Big Data Cluster Analysis. Pattern Recognition, 139, 109404. https://doi.org/10.1016/j.patcog.2023.109404
- Chen, Y. T., Witten, D. M. (2023). Selective inference for k-means clustering. Journal of Machine Learning Research, 24 (152), 1–41. Available at: https://jmlr.org/papers/v24/22-0371.html
- Mortensen, K. O., Zardbani, F., Haque, M. A., Agustsson, S. Y., Mottin, D., Hofmann, P., Karras, P. (2023). Marigold: Efficientk-Means Clustering in High Dimensions. Proceedings of the VLDB Endowment, 16 (7), 1740–1748. https://doi.org/10.14778/3587136.3587147
- Hu, H., Li, Z., Li, X., Yu, M., Pan, X. (2021). ScCAEs: deep clustering of single-cell RNA-seq via convolutional autoencoder embedding and soft K-means. Briefings in Bioinformatics, 23 (1). https://doi.org/10.1093/bib/bbab321
- Somu, N., Raman M R, G., Ramamritham, K. (2021). A deep learning framework for building energy consumption forecast. Renewable and Sustainable Energy Reviews, 137, 110591. https://doi.org/10.1016/j.rser.2020.110591
- Bisen, D., Lilhore, U. K., Manoharan, P., Dahan, F., Mzoughi, O., Hajjej, F. et al. (2023). A Hybrid Deep Learning Model Using CNN and K-Mean Clustering for Energy Efficient Modelling in Mobile EdgeIoT. Electronics, 12 (6), 1384. https://doi.org/10.3390/electronics12061384
- Kondruk, N. (2017). Clustering method based on fuzzy binary relation. Eastern-European Journal of Enterprise Technologies, 2 (4 (86)), 10–16. https://doi.org/10.15587/1729-4061.2017.94961
- Mohammed, A. S., Balaji, B. S., Basha M. S., S. (2019). Fuzzy applied energy aware clustering based routing for iot networks. Advanced Information Systems, 3 (4), 140–145. https://doi.org/10.20998/2522-9052.2019.4.22
- Krepych, S., Spivak, I. (2021). Improvement of svd algorithm to increase the efficiency of recommendation systems. Advanced Information Systems, 5 (4), 55–59. https://doi.org/10.20998/2522-9052.2021.4.08
- Khoroshun, G., Ryazantsev, O., Cherpitskyi, M. (2023). Clustering and anomalies of USA stock market volatility index data. Advanced Information Systems, 7 (2), 9–15. https://doi.org/10.20998/2522-9052.2023.2.02
- Shefer, O., Laktionov, O., Pents, V., Hlushko, A., Kuchuk, N. (2024). Practical principles of integrating artificial intelligence into the technology of regional security predicting. Advanced Information Systems, 8 (1), 86–93. https://doi.org/10.20998/2522-9052.2024.1.11
- davies_bouldin_score. Scikit-learn. Available at: https://scikit-learn.org/stable/modules/generated/sklearn.metrics.davies_bouldin_score.html
- silhouette_score. Scikit-learn. Available at: https://scikit-learn.org/stable/modules/generated/sklearn.metrics.silhouette_score.html
- Laktionov, A. (2019). Application of index estimates for improving accuracy during selection of machine operators. Eastern-European Journal of Enterprise Technologies, 3 (1 (99)), 18–26. https://doi.org/10.15587/1729-4061.2019.165884
- García-Tejedor, Á. J., Nogales, A. (2022). An open-source Python library for self-organizing-maps. Software Impacts, 12, 100280. https://doi.org/10.1016/j.simpa.2022.100280
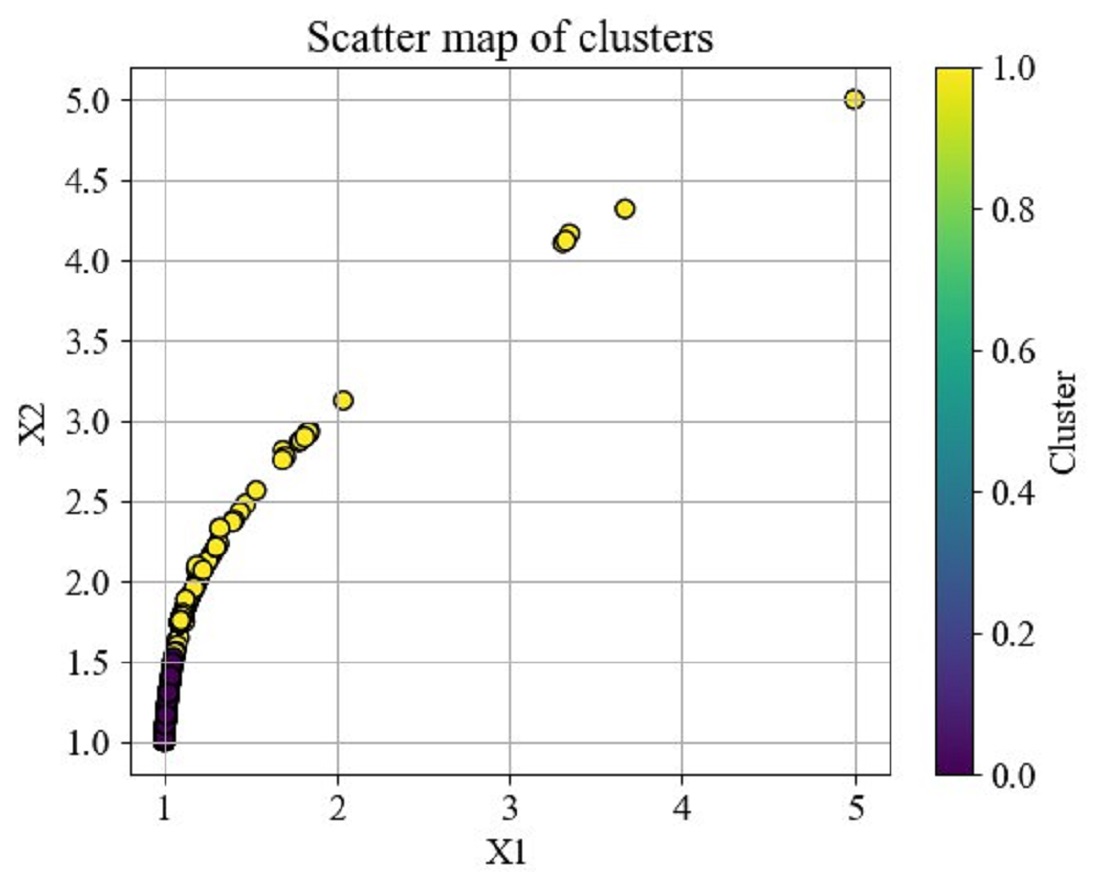
##submission.downloads##
Опубліковано
Як цитувати
Номер
Розділ
Ліцензія
Авторське право (c) 2024 Oleksandr Laktionov, Alina Yanko, Nazar Pedchenko

Ця робота ліцензується відповідно до Creative Commons Attribution 4.0 International License.
Закріплення та умови передачі авторських прав (ідентифікація авторства) здійснюється у Ліцензійному договорі. Зокрема, автори залишають за собою право на авторство свого рукопису та передають журналу право першої публікації цієї роботи на умовах ліцензії Creative Commons CC BY. При цьому вони мають право укладати самостійно додаткові угоди, що стосуються неексклюзивного поширення роботи у тому вигляді, в якому вона була опублікована цим журналом, але за умови збереження посилання на першу публікацію статті в цьому журналі.
Ліцензійний договір – це документ, в якому автор гарантує, що володіє усіма авторськими правами на твір (рукопис, статтю, тощо).
Автори, підписуючи Ліцензійний договір з ПП «ТЕХНОЛОГІЧНИЙ ЦЕНТР», мають усі права на подальше використання свого твору за умови посилання на наше видання, в якому твір опублікований. Відповідно до умов Ліцензійного договору, Видавець ПП «ТЕХНОЛОГІЧНИЙ ЦЕНТР» не забирає ваші авторські права та отримує від авторів дозвіл на використання та розповсюдження публікації через світові наукові ресурси (власні електронні ресурси, наукометричні бази даних, репозитарії, бібліотеки тощо).
За відсутності підписаного Ліцензійного договору або за відсутністю вказаних в цьому договорі ідентифікаторів, що дають змогу ідентифікувати особу автора, редакція не має права працювати з рукописом.
Важливо пам’ятати, що існує і інший тип угоди між авторами та видавцями – коли авторські права передаються від авторів до видавця. В такому разі автори втрачають права власності на свій твір та не можуть його використовувати в будь-який спосіб.