Розробка системи виявлення та класифікації внутрішніх дефектів труб
DOI:
https://doi.org/10.15587/1729-4061.2025.323293Ключові слова:
внутрішній дефект труби, виявлення, класифікація, алгоритми штучного інтелекту, розпізнавання образівАнотація
Об'єктом дослідження є виявлення та класифікація внутрішніх дефектів труб. Основною проблемою, що вимагає вирішення, є неефективність, висока вартість та низька точність традиційних методів ручного контролю, які часто вимагають великих витрат часу та схильні до людських помилок. Отримані результати включають створення мультимодальної платформи, що поєднує дані RGB-візуалізації та глибини з передовими алгоритмами штучного інтелекту, виявленням меж методом Кенні та заснованою на щільності просторовою кластеризацією для додатків з шумами (DBSCAN), забезпечуючи узагальнену середню точність (mAP) 93 % при виявленні та класифікації різних дефектів, таких як тріщини, корозія та засмічення. Короткий аналіз результатів показує, що висока продуктивність досягається завдяки синергії мультимодального зондування, розпізнавання образів за допомогою штучного інтелекту та надійної роботизованої навігації. Такий комплексний підхід забезпечує не тільки точність виявлення дефектів, але і їх виявлення в режимі реального часу. Особливості отриманих результатів, що безпосередньо спрямовані на вирішення визначеної проблеми, включають в себе високоточне виявлення дефектів в режимі реального часу та скорочення часу простою під час перевірки. Як наслідок, скорочується час перевірки, знижуються витрати та підвищується безпека трубопровідної системи, що забезпечує точне вимірювання показників (mAP 93 %) та зниження ризиків для безпеки праці. Розроблена система призначена для використання в традиційних промислових умовах, особливо у великих трубопровідних мережах та у випадках, коли традиційні методи малоефективні
Посилання
- Wang, W., Mao, X., Liang, H., Yang, D., Zhang, J., Liu, S. (2021). Experimental research on in-pipe leaks detection of acoustic signature in gas pipelines based on the artificial neural network. Measurement, 183, 109875. https://doi.org/10.1016/j.measurement.2021.109875
- Wong, B., McCann, J. A. (2021). Failure Detection Methods for Pipeline Networks: From Acoustic Sensing to Cyber-Physical Systems. Sensors, 21 (15), 4959. https://doi.org/10.3390/s21154959
- Barile, C., Casavola, C., Pappalettera, G., Kannan, V. P., Mpoyi, D. K. (2022). Acoustic Emission and Deep Learning for the Classification of the Mechanical Behavior of AlSi10Mg AM-SLM Specimens. Applied Sciences, 13 (1), 189. https://doi.org/10.3390/app13010189
- Altay, Y. A., Kuzivanov, D. O., Altay, D. A., Fedorov, A. V. (2024). Signal Processing for Acoustic Emission Signature Analysis and Defect Detection. 2024 26th International Conference on Digital Signal Processing and Its Applications (DSPA), 1–6. https://doi.org/10.1109/dspa60853.2024.10510110
- Wang, C., Tan, X. P., Tor, S. B., Lim, C. S. (2020). Machine learning in additive manufacturing: State-of-the-art and perspectives. Additive Manufacturing, 36, 101538. https://doi.org/10.1016/j.addma.2020.101538
- Sinha, S. K., Fieguth, P. W., Polak, M. A. (2003). Computer Vision Techniques for Automatic Structural Assessment of Underground Pipes. Computer-Aided Civil and Infrastructure Engineering, 18 (2), 95–112. https://doi.org/10.1111/1467-8667.00302
- Kim, H., Lee, B., Kim, R. (2006). Development of Computer-vision-based Pipe Inspection System. 2006 International Forum on Strategic Technology, 403–406. https://doi.org/10.1109/ifost.2006.312344
- Wang, M., Cheng, J. C. P. (2018). Development and Improvement of Deep Learning Based Automated Defect Detection for Sewer Pipe Inspection Using Faster R-CNN. Advanced Computing Strategies for Engineering, 171–192. https://doi.org/10.1007/978-3-319-91638-5_9
- Rayhana, R., Jiao, Y., Zaji, A., Liu, Z. (2021). Automated Vision Systems for Condition Assessment of Sewer and Water Pipelines. IEEE Transactions on Automation Science and Engineering, 18 (4), 1861–1878. https://doi.org/10.1109/tase.2020.3022402
- Moradi, S., Zayed, T., Golkhoo, F. (2019). Review on Computer Aided Sewer Pipeline Defect Detection and Condition Assessment. Infrastructures, 4 (1), 10. https://doi.org/10.3390/infrastructures4010010
- Oluwatosin, O. P., Syed, S. A., Apis, O., Kolawole, S. (2021). Application of Computer Vision in Pipeline Inspection Robot. Proceedings of the International Conference on Industrial Engineering and Operations Management. https://doi.org/10.46254/an11.20210374
- Colvalkar, A., Pawar, S. S., Patle, B. K. (2023). In-pipe inspection robotic system for defect detection and identification using image processing. Materials Today: Proceedings, 72, 1735–1742. https://doi.org/10.1016/j.matpr.2022.09.476
- Zholtayev, D., Dauletiya, D., Tileukulova, A., Akimbay, D., Nursultan, M., Bushanov, Y. et al. (2024). Smart Pipe Inspection Robot With In-Chassis Motor Actuation Design and Integrated AI-Powered Defect Detection System. IEEE Access, 12, 119520–119534. https://doi.org/10.1109/access.2024.3450502
- Haurum, J. B., Moeslund, T. B. (2021). Sewer-ML: A Multi-Label Sewer Defect Classification Dataset and Benchmark. 2021 IEEE/CVF Conference on Computer Vision and Pattern Recognition (CVPR), 13451–13462. https://doi.org/10.1109/cvpr46437.2021.01325
- Ru, G., Gao, B., Tang, Q., Jiang, S., Zhang, Y., Luo, F., Woo, W. L. (2023). Electromagnetic Coupling Sensing of Pipe In-Line Inspection System. IEEE Transactions on Instrumentation and Measurement, 72, 1–15. https://doi.org/10.1109/tim.2023.3310083
- Lyu, F., Zhou, X., Ding, Z., Qiao, X., Song, D. (2024). Application Research of Ultrasonic-Guided Wave Technology in Pipeline Corrosion Defect Detection: A Review. Coatings, 14 (3), 358. https://doi.org/10.3390/coatings14030358
- Niu, Y., Sun, L., Wang, Y., Shen, G., Shi, Y. (2024). New technology for pipeline defect detection. Science China Technological Sciences, 67 (4), 1294–1296. https://doi.org/10.1007/s11431-023-2473-x
- Mustafaev, B., Kim, S., Kim, E. (2023). Enhancing Metal Surface Defect Recognition Through Image Patching and Synthetic Defect Generation. IEEE Access, 11, 113339–113359. https://doi.org/10.1109/access.2023.3322734
- Li, Y., Wang, H., Dang, L. M., Song, H.-K., Moon, H. (2022). Vision-Based Defect Inspection and Condition Assessment for Sewer Pipes: A Comprehensive Survey. Sensors, 22 (7), 2722. https://doi.org/10.3390/s22072722
- Kenzhekhan, A., Bakytzhanova, A., Omirbayev, S., Tuieubayev, Y., Daniyalov, M., Yeshmukhametov, A. (2023). Design and Development of an In-Pipe Mobile Robot for Pipeline Inspection with AI Defect Detection System. 2023 23rd International Conference on Control, Automation and Systems (ICCAS), 579–584. https://doi.org/10.23919/iccas59377.2023.10316817
- Araújo, J. F., Ramos, V. M., Silva, C. A., Silva, H. D. (2024). Development of a Virtual Environment to Assist in the Identification and Analysis of Defects in Industrial Control Panels. IEEE Revista Iberoamericana de Tecnologias Del Aprendizaje, 19, 258–266. https://doi.org/10.1109/rita.2024.3475883
- Wang, X., Yang, L., Sun, T., Rasool, G., Sun, M., Hu, N., Guo, Z. (2023). A review of development and application of out-of-pipe detection technology without removing cladding. Measurement, 219, 113249. https://doi.org/10.1016/j.measurement.2023.113249
- Zhang, M., Guo, Y., Xie, Q., Zhang, Y., Wang, D., Chen, J. (2022). Defect identification for oil and gas pipeline safety based on autonomous deep learning network. Computer Communications, 195, 14–26. https://doi.org/10.1016/j.comcom.2022.08.001
- Changwang, S., Shaowei, H., Haifen, Z., Fuqu, P., Changxi, S., Hao, Q. (2024). Automatic Detection of Water Supply Pipe Defects Based on Underwater Image Enhancement and Improved YOLOX. Journal of Construction Engineering and Management, 150 (10). https://doi.org/10.1061/jcemd4.coeng-14919
- Fioravanti, C. C. B., Centeno, T. M., De Biase Da Silva Delgado, M. R. (2019). A Deep Artificial Immune System to Detect Weld Defects in DWDI Radiographic Images of Petroleum Pipes. IEEE Access, 7, 180947–180964. https://doi.org/10.1109/access.2019.2959810
- Lin, W., Li, P., Xie, X. (2022). A Novel Detection and Assessment Method for Operational Defects of Pipe Jacking Tunnel Based on 3D Longitudinal Deformation Curve: A Case Study. Sensors, 22 (19), 7648. https://doi.org/10.3390/s22197648
- Jeon, K.-W., Jung, E.-J., Bae, J.-H., Park, S.-H., Kim, J.-J., Chung, G. et al. (2024). Development of an In-Pipe Inspection Robot for Large-Diameter Water Pipes. Sensors, 24 (11), 3470. https://doi.org/10.3390/s24113470
- Luo, D., Du, K., Niu, D. (2024). Intelligent Diagnosis of Urban Underground Drainage Network: From Detection to Evaluation. Structural Control and Health Monitoring, 2024 (1). https://doi.org/10.1155/2024/9217395
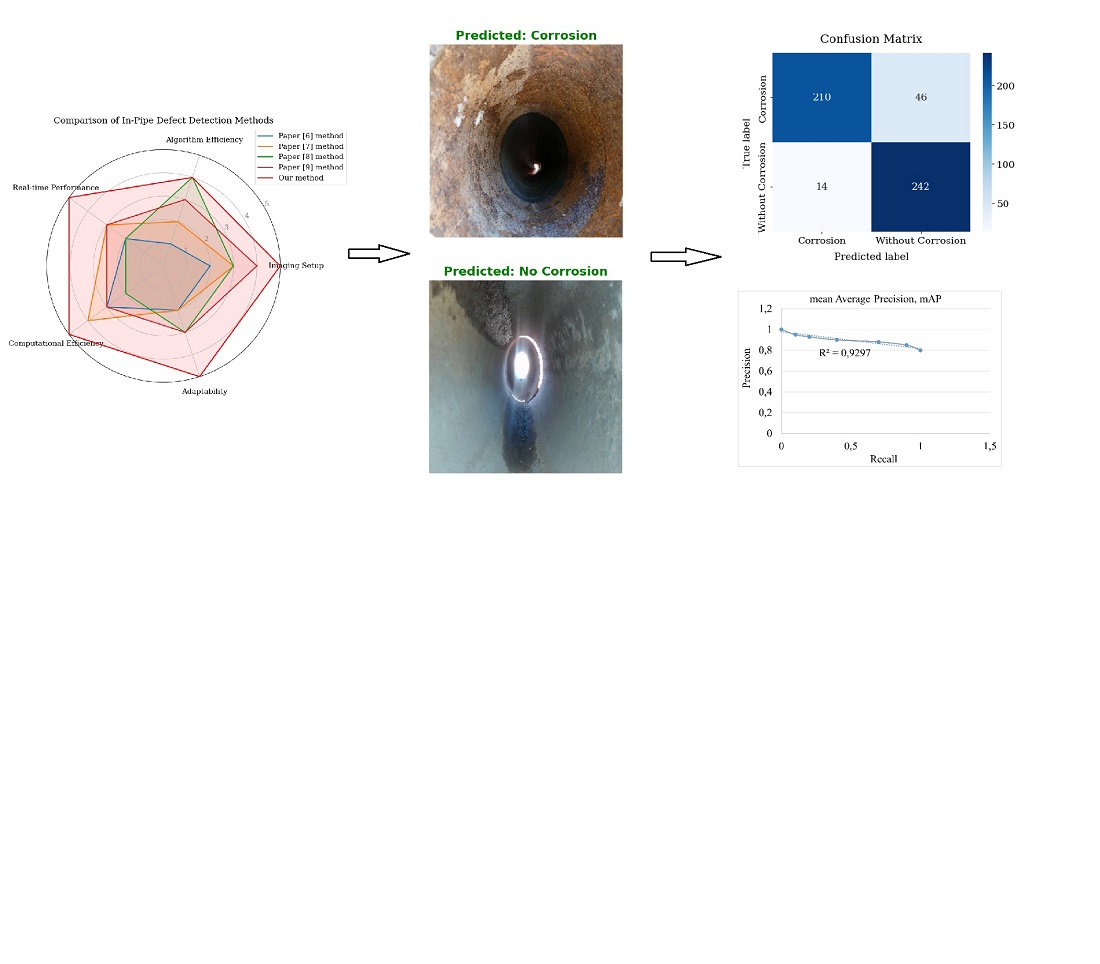
##submission.downloads##
Опубліковано
Як цитувати
Номер
Розділ
Ліцензія
Авторське право (c) 2025 Perizat Rakhmetova, Gani Sergazin, Yeldos Altay, Daniyar Dauletiya, Lazzat Kurmangaliyeva

Ця робота ліцензується відповідно до Creative Commons Attribution 4.0 International License.
Закріплення та умови передачі авторських прав (ідентифікація авторства) здійснюється у Ліцензійному договорі. Зокрема, автори залишають за собою право на авторство свого рукопису та передають журналу право першої публікації цієї роботи на умовах ліцензії Creative Commons CC BY. При цьому вони мають право укладати самостійно додаткові угоди, що стосуються неексклюзивного поширення роботи у тому вигляді, в якому вона була опублікована цим журналом, але за умови збереження посилання на першу публікацію статті в цьому журналі.
Ліцензійний договір – це документ, в якому автор гарантує, що володіє усіма авторськими правами на твір (рукопис, статтю, тощо).
Автори, підписуючи Ліцензійний договір з ПП «ТЕХНОЛОГІЧНИЙ ЦЕНТР», мають усі права на подальше використання свого твору за умови посилання на наше видання, в якому твір опублікований. Відповідно до умов Ліцензійного договору, Видавець ПП «ТЕХНОЛОГІЧНИЙ ЦЕНТР» не забирає ваші авторські права та отримує від авторів дозвіл на використання та розповсюдження публікації через світові наукові ресурси (власні електронні ресурси, наукометричні бази даних, репозитарії, бібліотеки тощо).
За відсутності підписаного Ліцензійного договору або за відсутністю вказаних в цьому договорі ідентифікаторів, що дають змогу ідентифікувати особу автора, редакція не має права працювати з рукописом.
Важливо пам’ятати, що існує і інший тип угоди між авторами та видавцями – коли авторські права передаються від авторів до видавця. В такому разі автори втрачають права власності на свій твір та не можуть його використовувати в будь-який спосіб.