Development of a neural network approach for risk management in helicopter technical condition diagnostics
DOI:
https://doi.org/10.15587/1729-4061.2024.312345Keywords:
helicopter engine, Digital Twin (DT), risk management, neural network, simulation modelAbstract
The object of the study is the quality of helicopter maintenance based on digital diagnostic tools. To ensure the required quality, quantitative risk assessment models for the in-depth and express diagnostics system of helicopter gas turbine engines in a neural network environment are proposed. The assessment of diagnostic efficiency is based on the analysis of probable control risks by standard deviations, which distinguishes the proposed approach from the traditional one. Two diagnostic modes are considered: rapid diagnostics exemplified by vibration diagnostics, and in-depth diagnostics, including both vibration diagnostics and pyrometric control. These diagnostic methods make it possible to implement a remote monitoring system at aircraft repair facilities, which significantly reduces maintenance labor intensity. As a result, it was found that control risks depend not only on the metrological level of measuring instruments but also on a combination of the statistical nature of control agents in their system composition according to the following characteristics: statistical parameters of the controlled indicator, distribution laws, and values of uncertainty of measuring instruments, as well as the uncertainty of control standards (tolerances). In the modeling process, risks were assessed as a function of the ratio of uncertainties of measuring instruments to the uncertainty of the controlled parameter, with varying values of the standard (tolerance). This approach will allow, in practice, the creation of a more effective system for monitoring and collecting statistical information on the operational reliability of the Mi-8 helicopter engine, where the quality of control is predicted to a greater extent based on the metrological indicators of the measuring instruments and methods
References
- On approval of the Concept for the development of artificial intelligence for 2024–2029. Information and legal system of regulatory legal acts of the Republic of Kazakhstan. Available at: https://adilet.zan.kz/rus/docs/P2400000592
- Sreenatha, M., Mallikarjuna, P. B. (2023). A Fault Diagnosis Technique for Wind Turbine Gearbox: An Approach using Optimized BLSTM Neural Network with Undercomplete Autoencoder. Engineering, Technology & Applied Science Research, 13 (1), 10170–10174. https://doi.org/10.48084/etasr.5595
- Prommachan, W., Surin, P., Srinoi, P., Pipathattakul, M. (2024). Selection Criteria for Evaluating Predictive Maintenance Techniques for Rotating Machinery using the Analytic Hierarchical Process (AHP). Engineering, Technology & Applied Science Research, 14 (1), 13058–13065. https://doi.org/10.48084/etasr.6816
- Martins, J. A., Romao, E. C. (2024). Fracture Analysis of a Cycloidal Gearbox as a Yaw Drive on a Wind Turbine. Engineering, Technology & Applied Science Research, 14 (1), 12640–12645. https://doi.org/10.48084/etasr.6613
- Molina-Jorge, Ó., Terrón-López, M.-J., Latorre-Dardé, R. (2024). A Quantitative Assessment Approach to Implement Pneumatic Waste Collection System Using a New Expert Decision Matrix Related to UN SDGs. Applied Sciences, 14 (18), 8306. https://doi.org/10.3390/app14188306
- Fundamentals of Intelligent Neural Networks (2017). Available at: https://neural.radkopeter.ru/chapter/%D0%BE%D1%81%D0%BD%D0%BE%D0%B2%D1%8B-%D0%B8%D0%BD%D1%81/
- Nayt, F. H. (2003). Risk, Uncertainty, and Profit. Moscow. Available at: https://elib.hse.ru/incoming/docs/book5774903060.pdf
- EUROCHEM/CITAC Guide "Quantifying Uncertainty in Analytical Measurements" (2000).
- IEC 31010:2019. Risk management – Risk assessment techniques.
- Marzhan, Y., Talshyn, K., Kairat, K., Saule, B., Karlygash, A., Yerbol, O. (2022). Smart technologies of the risk-management and decision-making systems in a fuzzy data environment. Indonesian Journal of Electrical Engineering and Computer Science, 28 (3), 1463. https://doi.org/10.11591/ijeecs.v28.i3.pp1463-1474
- Alibekkyzy, K., Koshekov, K., Keribayeva, T., Akayev, A., Baidildina, A. (2023). Robust Data Transfer Paradigm Based on VLC Technologies. Trudy Universiteta. https://doi.org/10.52209/1609-1825_2023_2_397
- Ainakulov, Z., Pirmanov, I., Koshekov, K., Astapenko, N., Fedorov, I., Zuev, D., Kurmankulova, G. (2022). Risk Assessment of the Operation of Aviation Maintenance Personnel Trained on Virtual Reality Simulators. Transport and Telecommunication Journal, 23 (4), 320–333. https://doi.org/10.2478/ttj-2022-0026
- Negri, E., Fumagalli, L., Macchi, M. (2017). A Review of the Roles of Digital Twin in CPS-based Production Systems. Procedia Manufacturing, 11, 939–948. https://doi.org/10.1016/j.promfg.2017.07.198
- Tao, F., Zhang, H., Liu, A., Nee, A. Y. C. (2019). Digital Twin in Industry: State-of-the-Art. IEEE Transactions on Industrial Informatics, 15 (4), 2405–2415. https://doi.org/10.1109/tii.2018.2873186
- Jordan, M. I., Bishop, C. M. (1996). Neural networks. ACM Computing Surveys, 28 (1), 73–75. https://doi.org/10.1145/234313.234348
- Baumont, C., Ertur, C., Le Gallo, J. (2000). Convergence des régions européennes (une approche par l’économétrie spatiale). HAL. Available at: https://hal.science/hal-01526961/document
- Ainakulov, Z., Koshekov, K., Astapenko, N., Pirmanov, I., Koshekov, A. (2023). The experience of introducing digital twins into the educational process on the example of training in the repair of aircraft equipment units. Journal of Theoretical and Applied Information Technology, 101 (12), 5123–5134. Available at: http://www.jatit.org/volumes/Vol101No12/24Vol101No12.pdf
- Vintizenko, I., Tcherkasov, A. (2010). Diadich Quantitative Risks of Chains of Consecutive Economic Projects. Bulletin of the Adyge State University, 4, 63–69.
- Nahar, S., Inder, B. (2002). Testing convergence in economic growth for OECD countries. Applied Economics, 34 (16), 2011–2022. https://doi.org/10.1080/00036840110117837
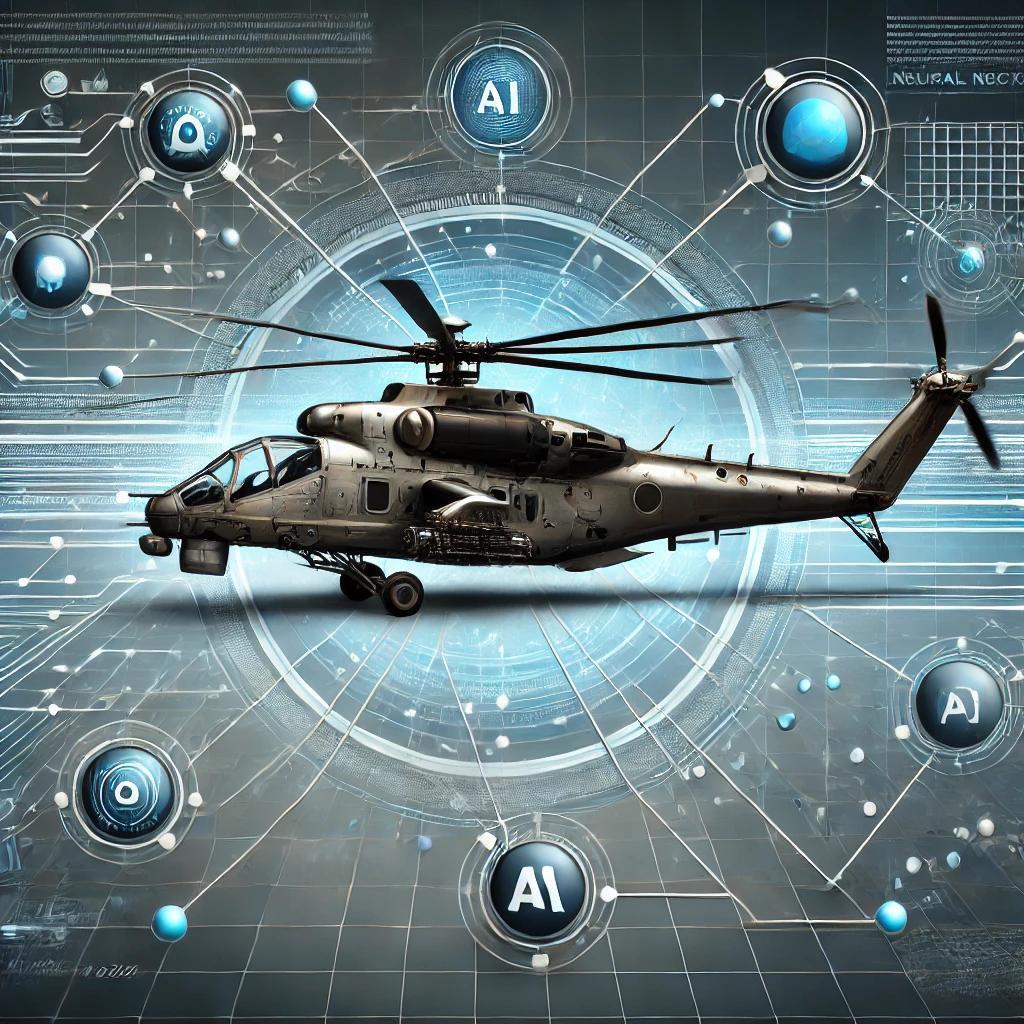
Downloads
Published
How to Cite
Issue
Section
License
Copyright (c) 2024 Kayrat Koshekov, Ildar Pirmanov, Saltanat Kenbeilova, Abay Koshekov, Rustam Togambayev, Beglan Toiganbayev

This work is licensed under a Creative Commons Attribution 4.0 International License.
The consolidation and conditions for the transfer of copyright (identification of authorship) is carried out in the License Agreement. In particular, the authors reserve the right to the authorship of their manuscript and transfer the first publication of this work to the journal under the terms of the Creative Commons CC BY license. At the same time, they have the right to conclude on their own additional agreements concerning the non-exclusive distribution of the work in the form in which it was published by this journal, but provided that the link to the first publication of the article in this journal is preserved.
A license agreement is a document in which the author warrants that he/she owns all copyright for the work (manuscript, article, etc.).
The authors, signing the License Agreement with TECHNOLOGY CENTER PC, have all rights to the further use of their work, provided that they link to our edition in which the work was published.
According to the terms of the License Agreement, the Publisher TECHNOLOGY CENTER PC does not take away your copyrights and receives permission from the authors to use and dissemination of the publication through the world's scientific resources (own electronic resources, scientometric databases, repositories, libraries, etc.).
In the absence of a signed License Agreement or in the absence of this agreement of identifiers allowing to identify the identity of the author, the editors have no right to work with the manuscript.
It is important to remember that there is another type of agreement between authors and publishers – when copyright is transferred from the authors to the publisher. In this case, the authors lose ownership of their work and may not use it in any way.